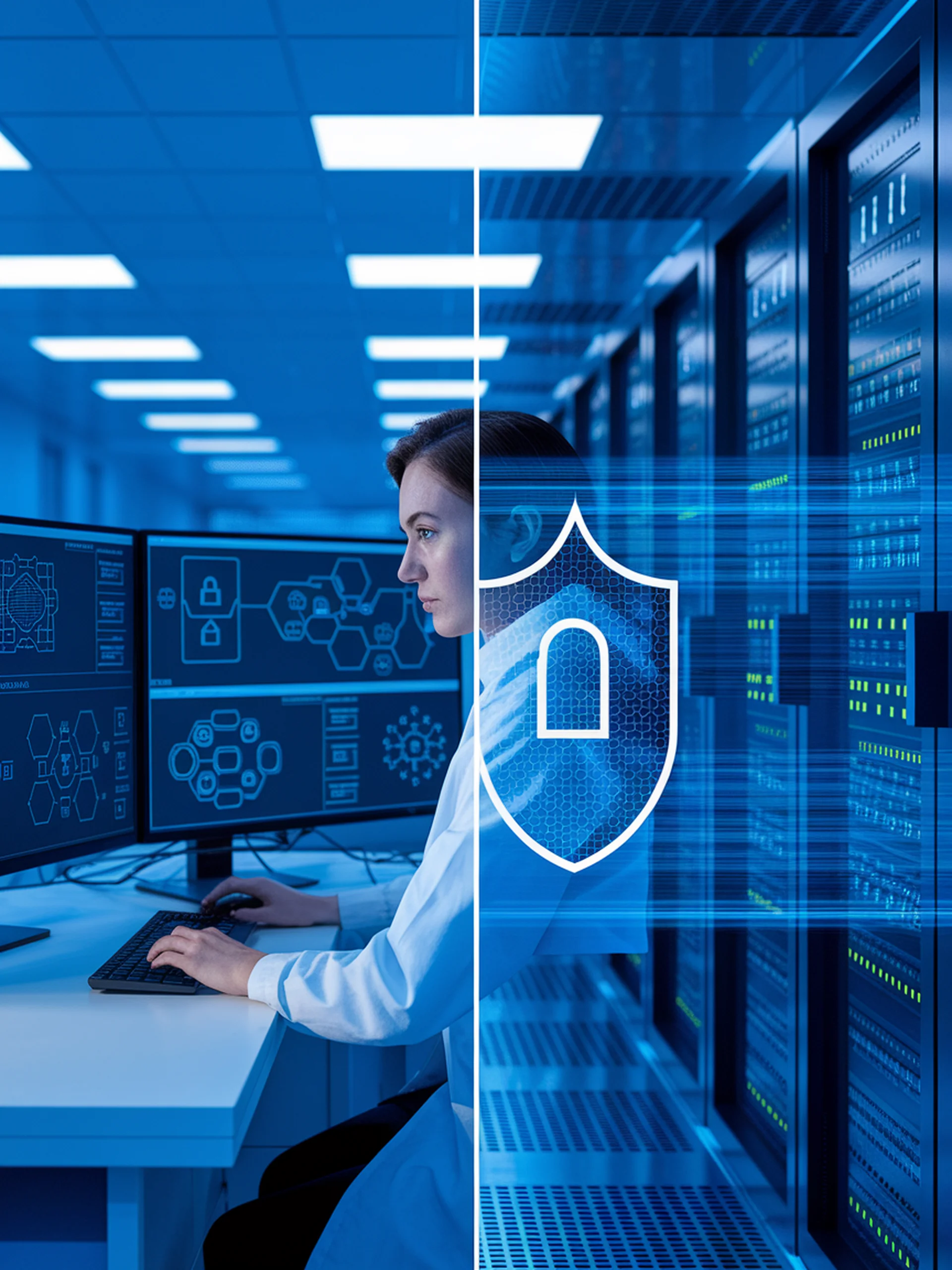
Privacy-Preserving Knowledge Transfer for LLMs
Balancing domain-specific knowledge utility with data privacy
This research introduces a novel model-based knowledge transfer approach that enables LLMs to leverage domain expertise while maintaining strict privacy protections.
- Addresses key limitations in both retrieval-augmented generation (RAG) and differentially private data synthesis
- Develops a framework allowing domain experts to transfer knowledge without exposing sensitive data
- Implements a two-stage approach with a small model learning domain knowledge under privacy constraints before transferring to larger LLMs
- Demonstrates superior performance compared to existing privacy-preserving methods
This advancement is particularly significant for security applications where protecting sensitive information is paramount while still enabling LLMs to leverage specialized knowledge.
Model-Based Privacy-Preserving Knowledge Transfer for Large Language Models