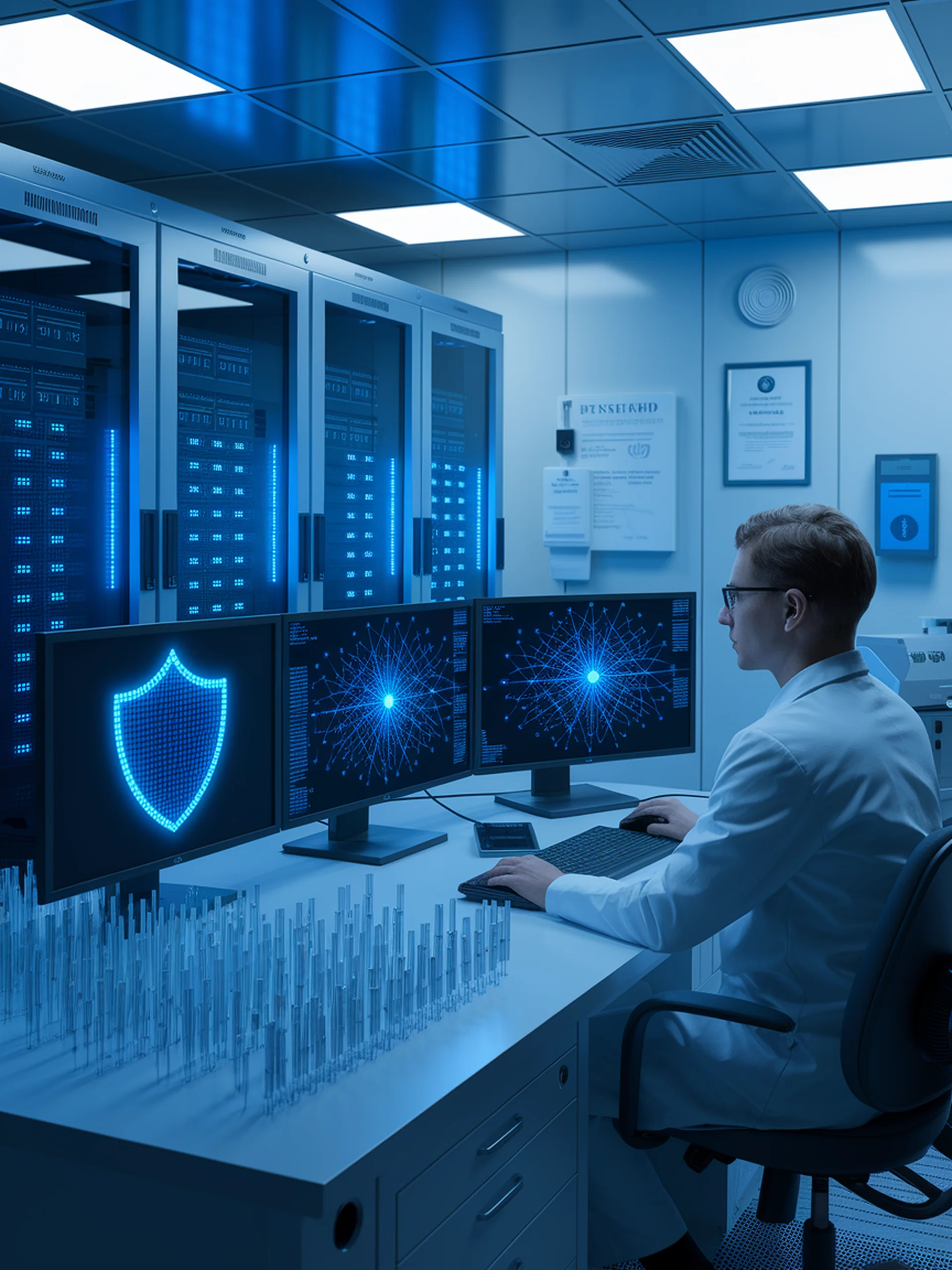
Protecting Privacy in LLM Fine-tuning
Addressing security vulnerabilities in the fine-tuning process
This research provides a comprehensive survey of privacy risks that emerge when fine-tuning Large Language Models (LLMs) on sensitive datasets.
- Identifies major attack vectors including membership inference, data extraction, and backdoor attacks
- Evaluates existing defense mechanisms for securing the fine-tuning process
- Outlines future research directions to enhance privacy protections
As organizations increasingly customize LLMs with proprietary or sensitive data, understanding these vulnerabilities becomes critical for maintaining data security and regulatory compliance.
Privacy in Fine-tuning Large Language Models: Attacks, Defenses, and Future Directions