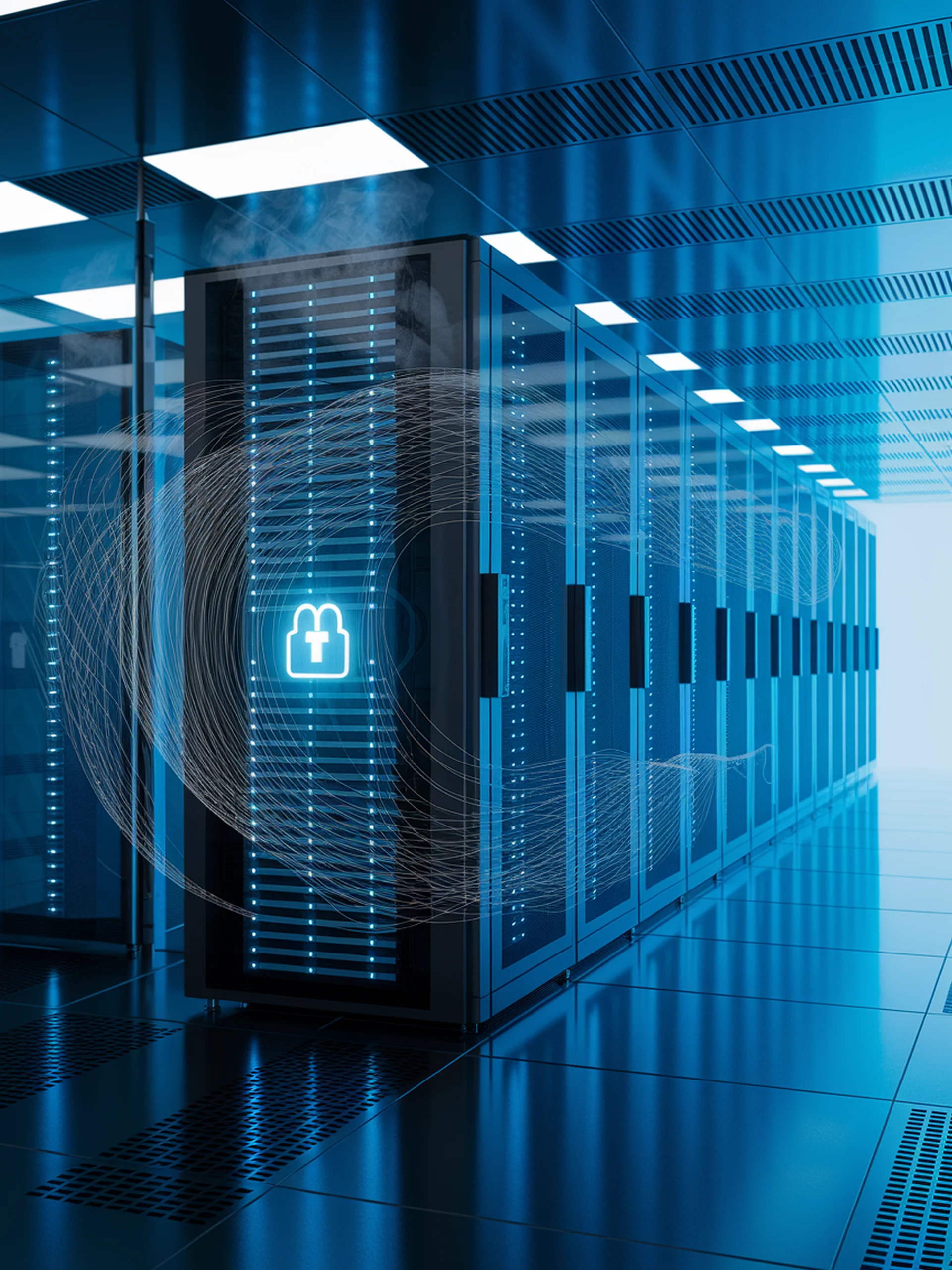
Privacy-Preserving Language Models at Scale
Understanding the tradeoffs between privacy, computation, and model utility
This research establishes scaling laws for differentially private language models, providing critical guidance for balancing privacy protections with model performance when training on sensitive user data.
- Privacy protections significantly impact traditional scaling relationships
- Mathematical framework predicts performance across model sizes and privacy levels
- Enables informed decisions about compute-privacy-utility tradeoffs
- Helps organizations determine optimal resource allocation for private LLMs
For security professionals, these findings offer evidence-based strategies to implement privacy guarantees while maximizing model utility, ensuring sensitive user data can be leveraged responsibly for AI development.