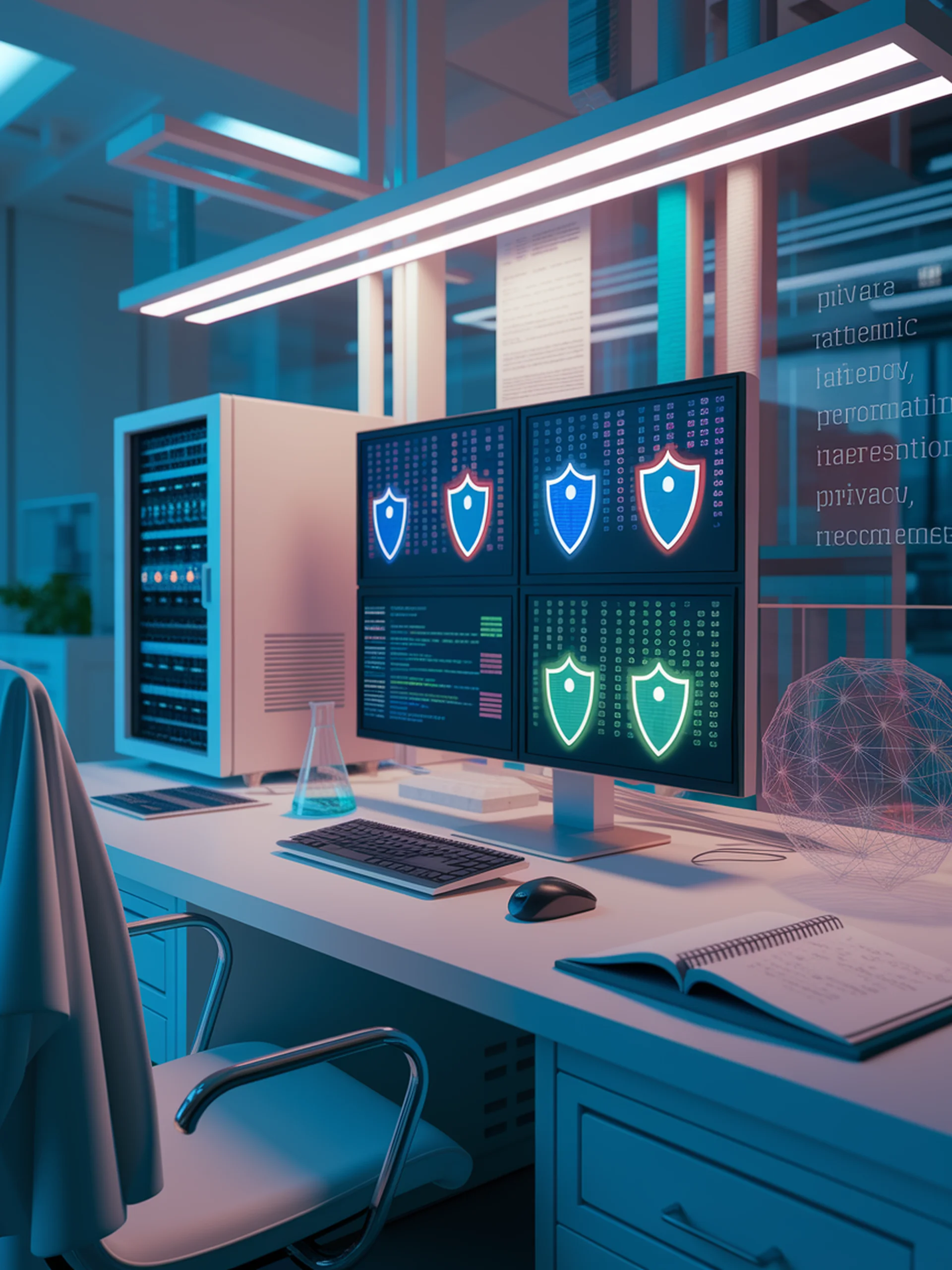
Privacy-Preserving Data Synthesis
Creating high-quality synthetic data with privacy guarantees
This research introduces a novel approach to generate synthetic data that maintains the utility of real data while ensuring differential privacy protection.
- Leverages multiple pre-trained language models through weighted fusion techniques
- Employs contrastive learning to improve quality in data-deficient scenarios
- Provides formal privacy guarantees through differential privacy mechanisms
- Demonstrates practical applications for sensitive data environments
Key significance: Organizations can now create high-quality training datasets without compromising individual privacy, especially crucial for sensitive domains like healthcare and finance.
Contrastive Private Data Synthesis via Weighted Multi-PLM Fusion