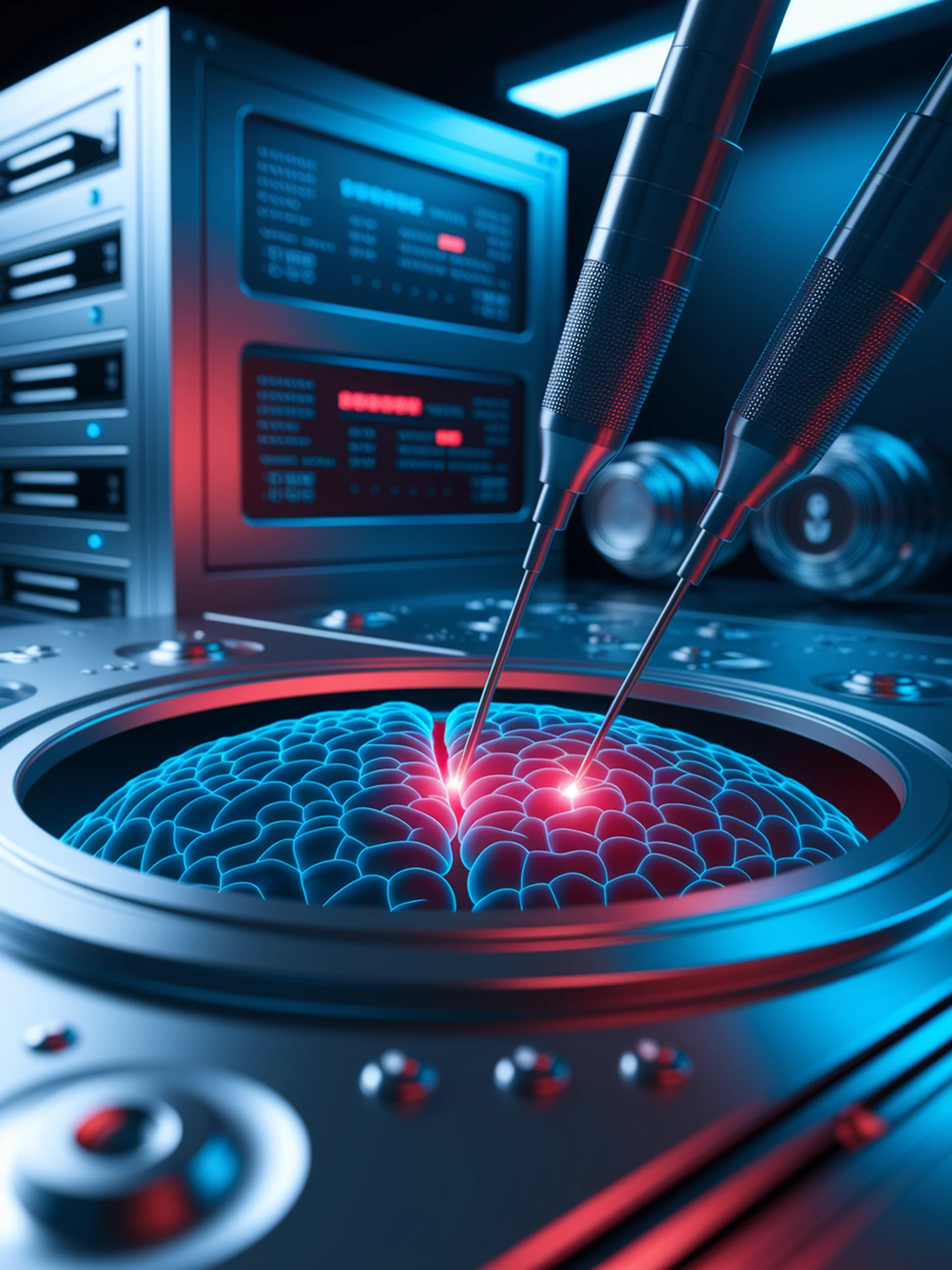
Precision Unlearning for AI Security
A novel approach to selectively remove harmful information from language models
FALCON introduces fine-grained activation manipulation techniques that allow precise removal of sensitive information from large language models while preserving overall performance.
- Uses contrastive orthogonal unlearning to target specific knowledge rather than broad categories
- Achieves superior balance between information removal and model utility preservation
- Operates at the activation level for more precise control than traditional loss-based approaches
- Addresses critical security concerns by preventing harmful information retention in AI systems
This research matters for security professionals as it provides a more reliable method to ensure AI systems cannot retain or expose sensitive data, significantly reducing potential security vulnerabilities in deployed models.