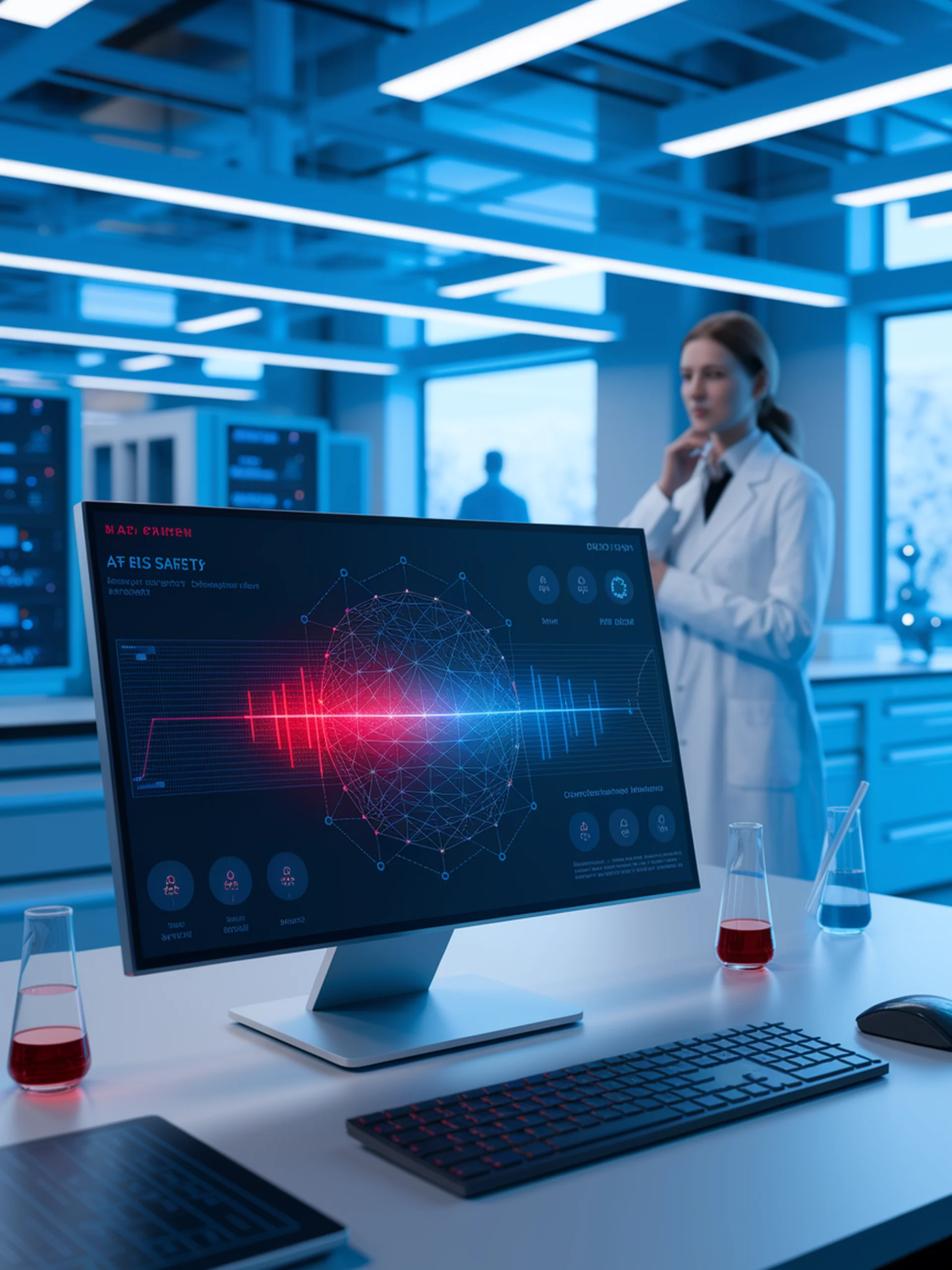
Adaptive Abstention in AI Decision-Making
Enhancing LLM/VLM Safety Through Dynamic Risk Management
This research introduces a conformal abstention policy framework for large language and vision-language models, enabling them to withhold predictions when uncertainty is high.
- Improves on traditional conformal prediction by dynamically adapting abstention thresholds based on task complexity
- Employs a reinforcement learning approach to learn optimal abstention policies
- Demonstrates significant performance gains across diverse tasks while maintaining statistical guarantees
- Provides a practical solution for deploying AI systems in security-critical environments
For security applications, this method offers crucial reliability improvements by allowing models to recognize their limitations and abstain from high-risk decisions, reducing potential harm from incorrect predictions.