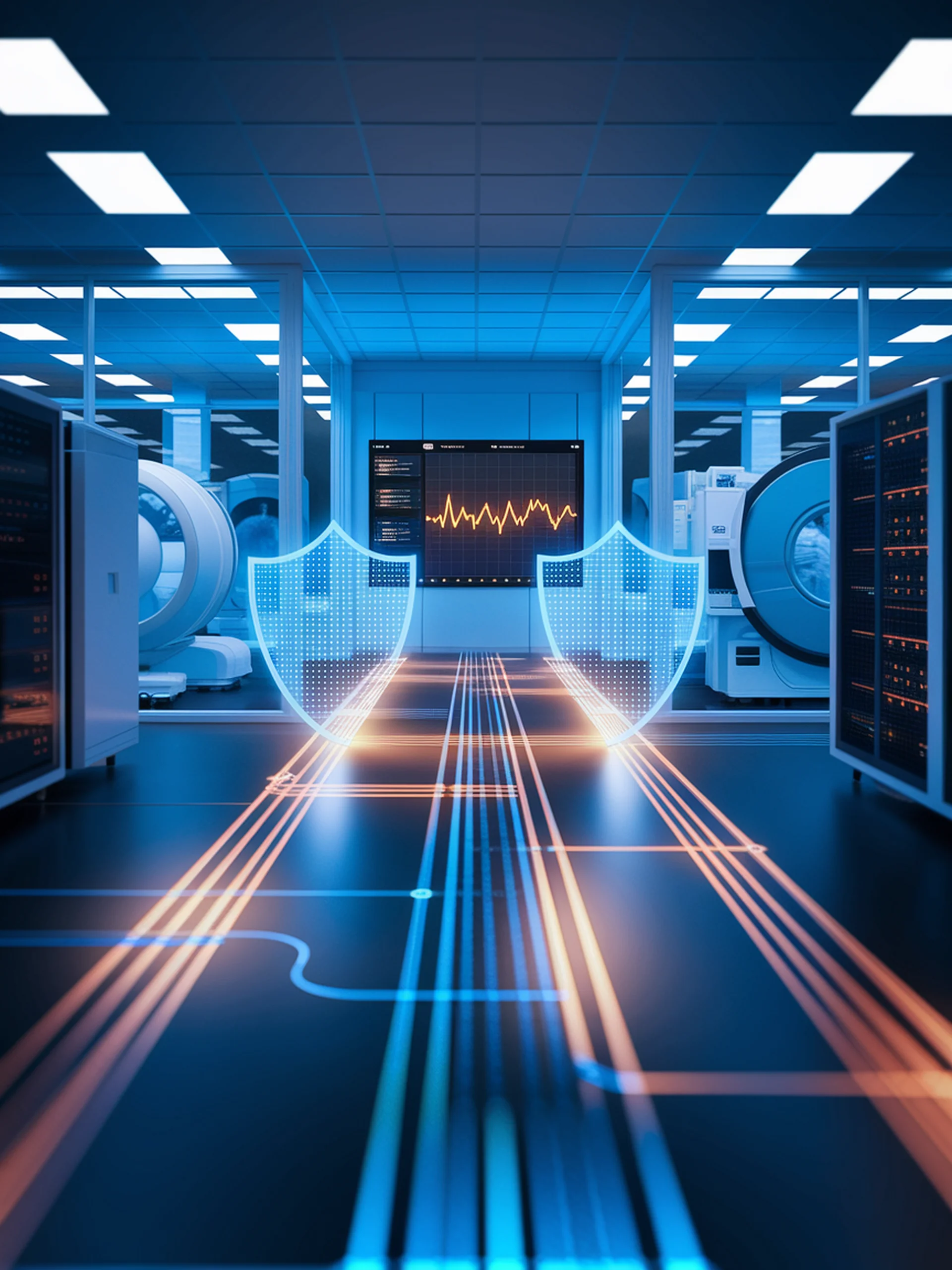
Privacy-Preserving Federated Learning for LLMs
Interactive Framework for Balancing Privacy and Performance
This research presents a novel interactive framework that bridges the gap between Differential Privacy (DP) and practical federated learning implementations for large language models.
- Demonstrates how privacy-utility trade-offs can be visualized and managed through an interactive system
- Provides empirical evidence that DP can be implemented with acceptable performance costs
- Enables organizations to customize privacy settings based on specific risk tolerances and use cases
- Shows that privacy-preserving techniques can be applied to large-scale language models without prohibitive performance degradation
This work is significant for the security community as it offers practical implementations of privacy protections for sensitive data while maintaining model utility—critical for widespread adoption in regulated industries and sensitive applications.