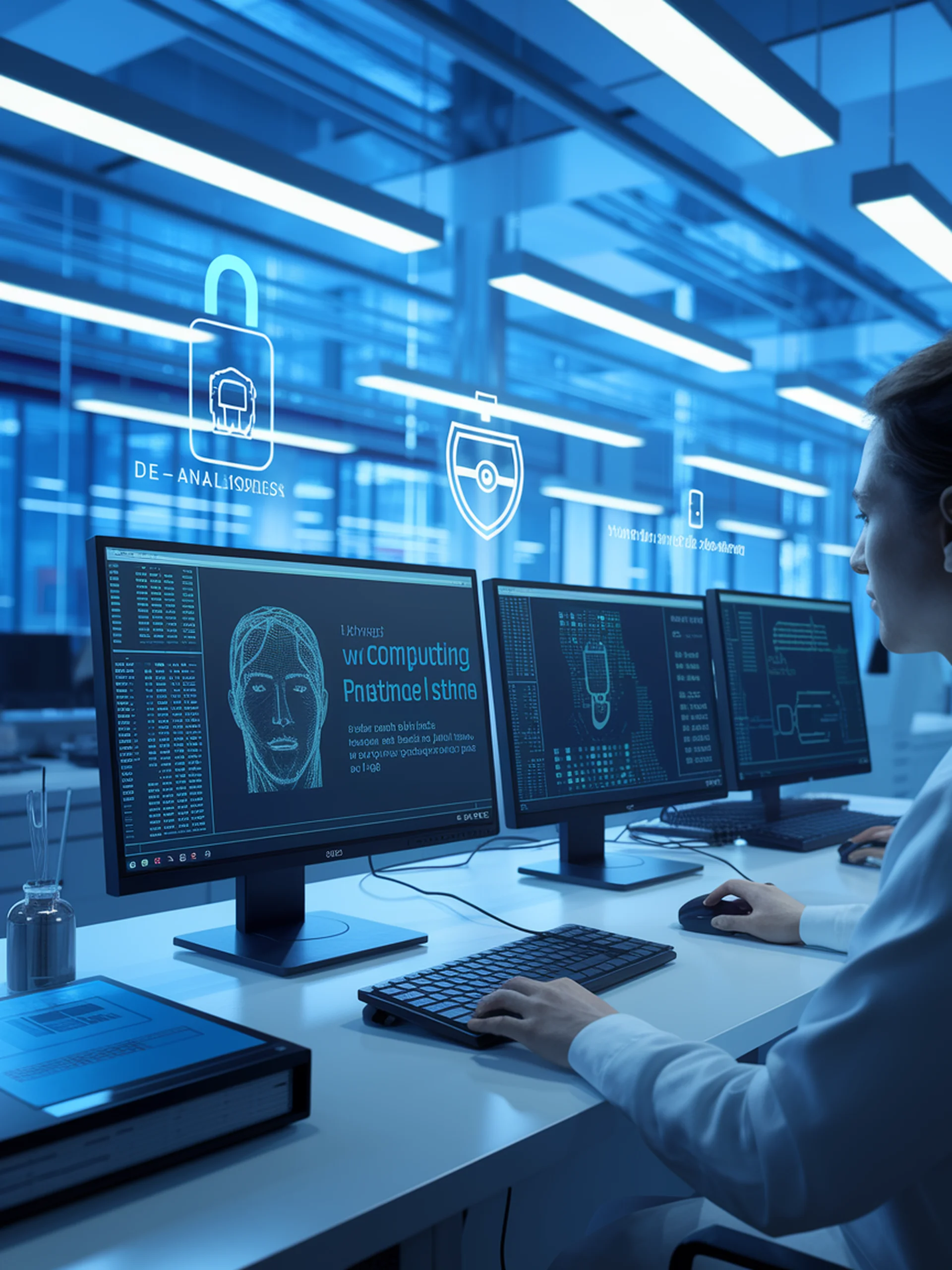
Synthetic Clinical Data for Privacy-Preserving AI
Using LLMs to create training data for de-identification systems
This research demonstrates how large language models can generate synthetic clinical data with privacy annotations, addressing the critical shortage of training datasets in sensitive domains.
- Domain-adapted LLMs create realistic synthetic clinical texts
- Advanced NER models automatically add de-identification tags
- The synthetic corpora effectively train models for identifying personally identifiable information
- Overcomes privacy barriers that limit healthcare AI progress
For healthcare organizations, this approach provides a path to develop robust de-identification systems without exposing real patient data, accelerating AI adoption while maintaining privacy compliance.
Data-Constrained Synthesis of Training Data for De-Identification