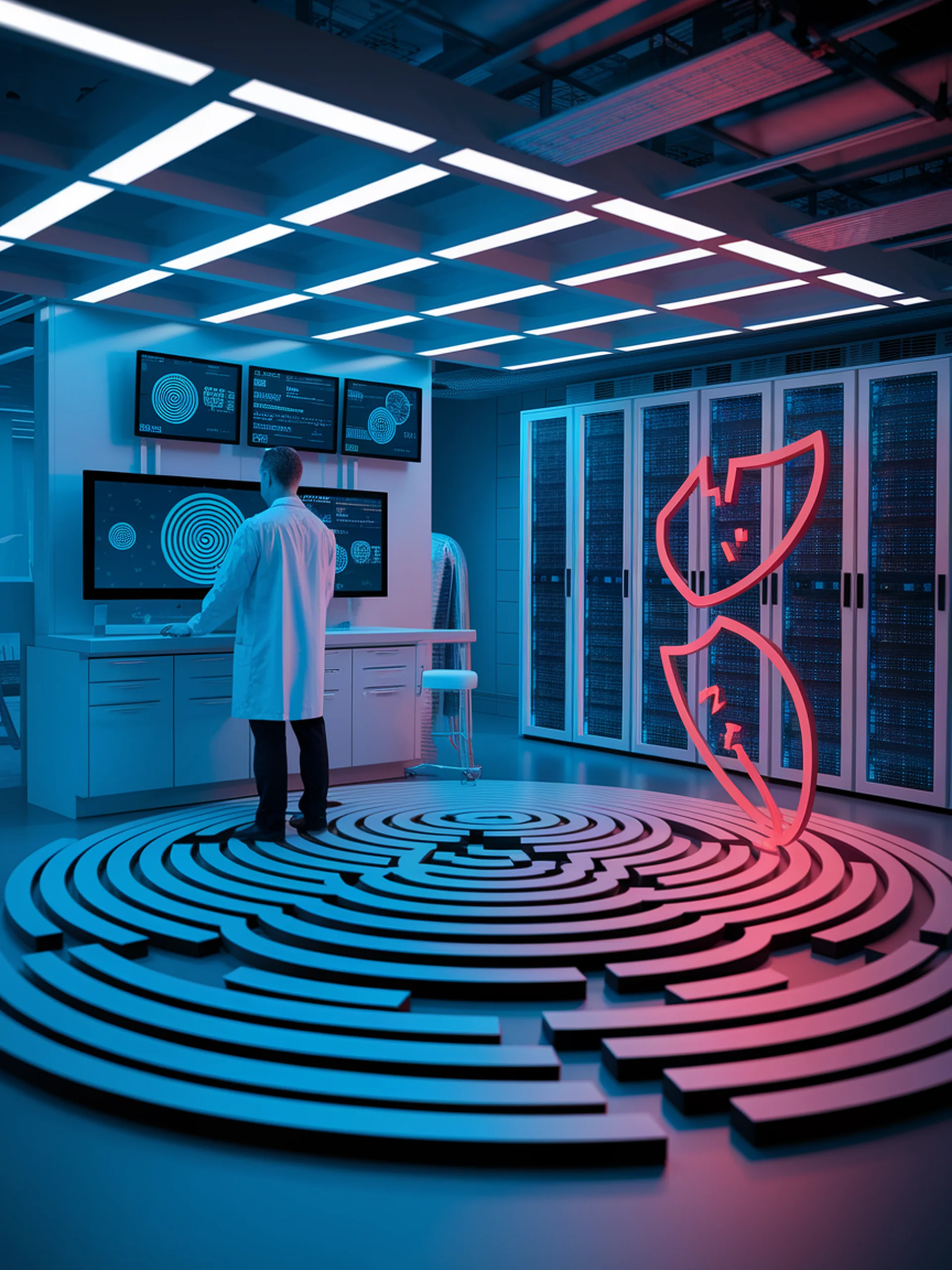
Privacy Ripple Effects in LLMs
How adding or removing personal data impacts model security
This research reveals that PII memorization in language models is a dynamic property affected by surrounding data, creating ripple effects when personal information is added or removed.
- Adding personal information can cause unexpected memorization of supposedly unrelated PII
- Removing data often leaves residual traces that can still expose sensitive information
- Models show varying vulnerability to these effects depending on architecture and training approach
- The findings demonstrate that PII privacy guarantees require more sophisticated protections than simple data removal
For security professionals, this research highlights critical gaps in current privacy protection approaches for LLMs and suggests the need for more robust data management frameworks.
Privacy Ripple Effects from Adding or Removing Personal Information in Language Model Training