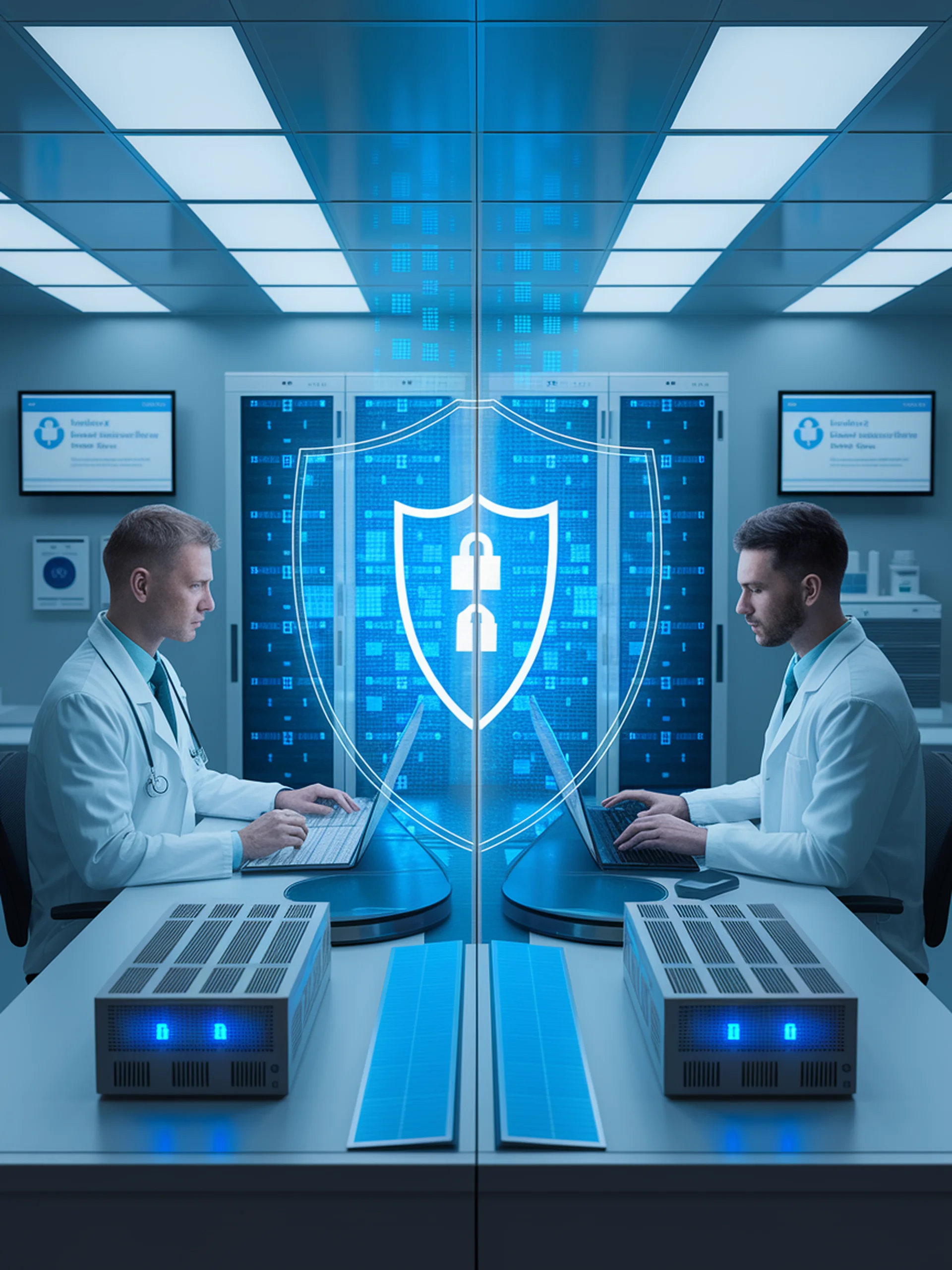
Privacy-Preserving LLM Fine-Tuning
Protecting Sensitive Data in Healthcare and Finance with Reward-Driven Synthesis
RewardDS introduces a novel framework that enables fine-tuning large language models while preserving privacy in sensitive domains.
- Uses reward-driven data synthesis to replace private data with high-quality synthetic alternatives
- Implements Differential Privacy (DP) guarantees for robust privacy protection
- Intelligently filters out flawed synthetic data to maximize model performance
- Demonstrates effectiveness in healthcare and financial applications
Why This Matters for Healthcare: Medical institutions can now fine-tune specialized LLMs without risking patient data exposure, enabling advanced AI applications while maintaining strict privacy compliance and patient trust.
RewardDS: Privacy-Preserving Fine-Tuning for Large Language Models via Reward Driven Data Synthesis