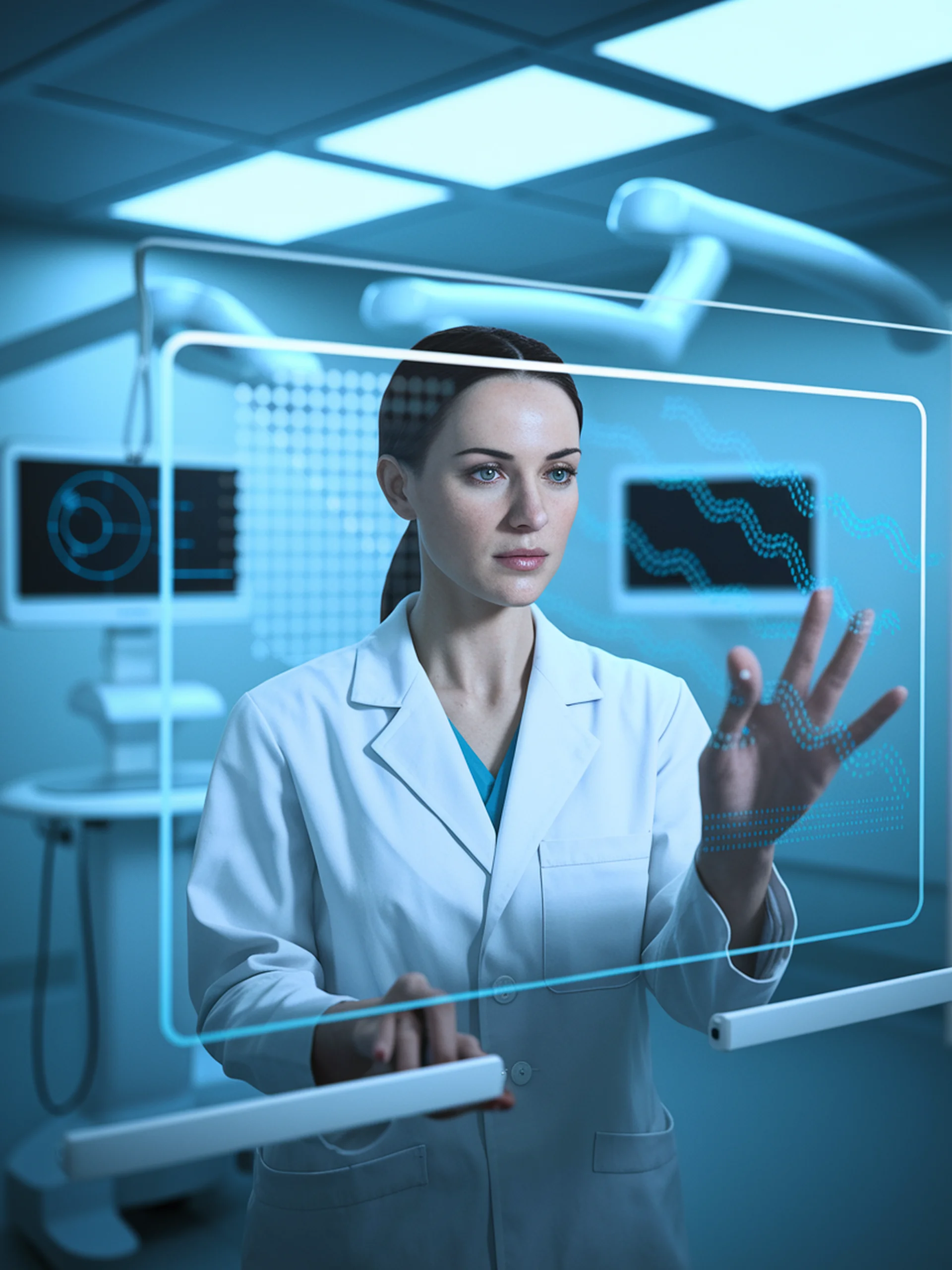
Making LLMs Safer for Women's Healthcare
Using Semantic Entropy to Reduce Hallucinations in Clinical Contexts
This research introduces a novel approach to identify and reduce hallucinations in large language models when applied to women's health contexts.
- Uses semantic entropy to detect when LLMs are likely to produce unreliable answers
- Demonstrates improved safety in obstetrics & gynecology applications
- Provides a framework for measuring uncertainty in clinical LLM responses
- Shows potential to significantly reduce risks in medical AI deployment
Why it matters: In high-stakes healthcare settings, particularly women's health, LLM errors can have serious consequences for patient outcomes. This approach helps bridge the reliability gap preventing wider adoption of AI in clinical decision support.
Reducing Large Language Model Safety Risks in Women's Health using Semantic Entropy