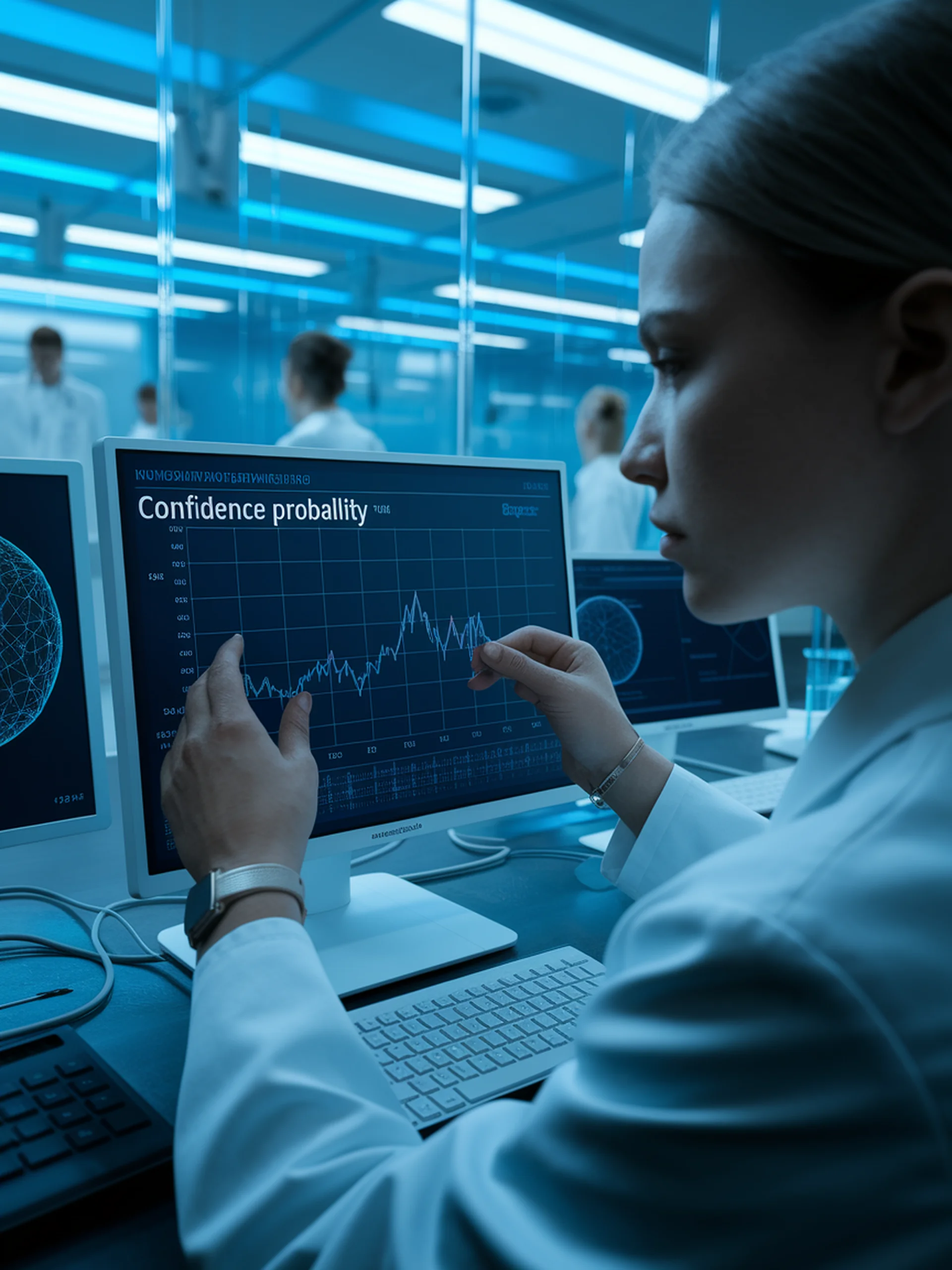
Teaching AI to Know When It Doesn't Know
A reinforcement learning approach for confidence calibration in LLMs
This research introduces a novel betting game framework that trains large language models to accurately express confidence in their answers, enhancing safety and trustworthiness.
Key Innovations:
- Uses reinforcement learning to calibrate LLM confidence estimations
- Models confidence as a betting game with rewards for accurate self-assessment
- Penalizes both over-confidence and under-confidence
- Specifically targets factual question-answering scenarios
For security applications, this approach significantly reduces the risk of LLMs providing misleadingly confident but incorrect information, which is crucial for deployment in high-stakes environments like healthcare or critical infrastructure.