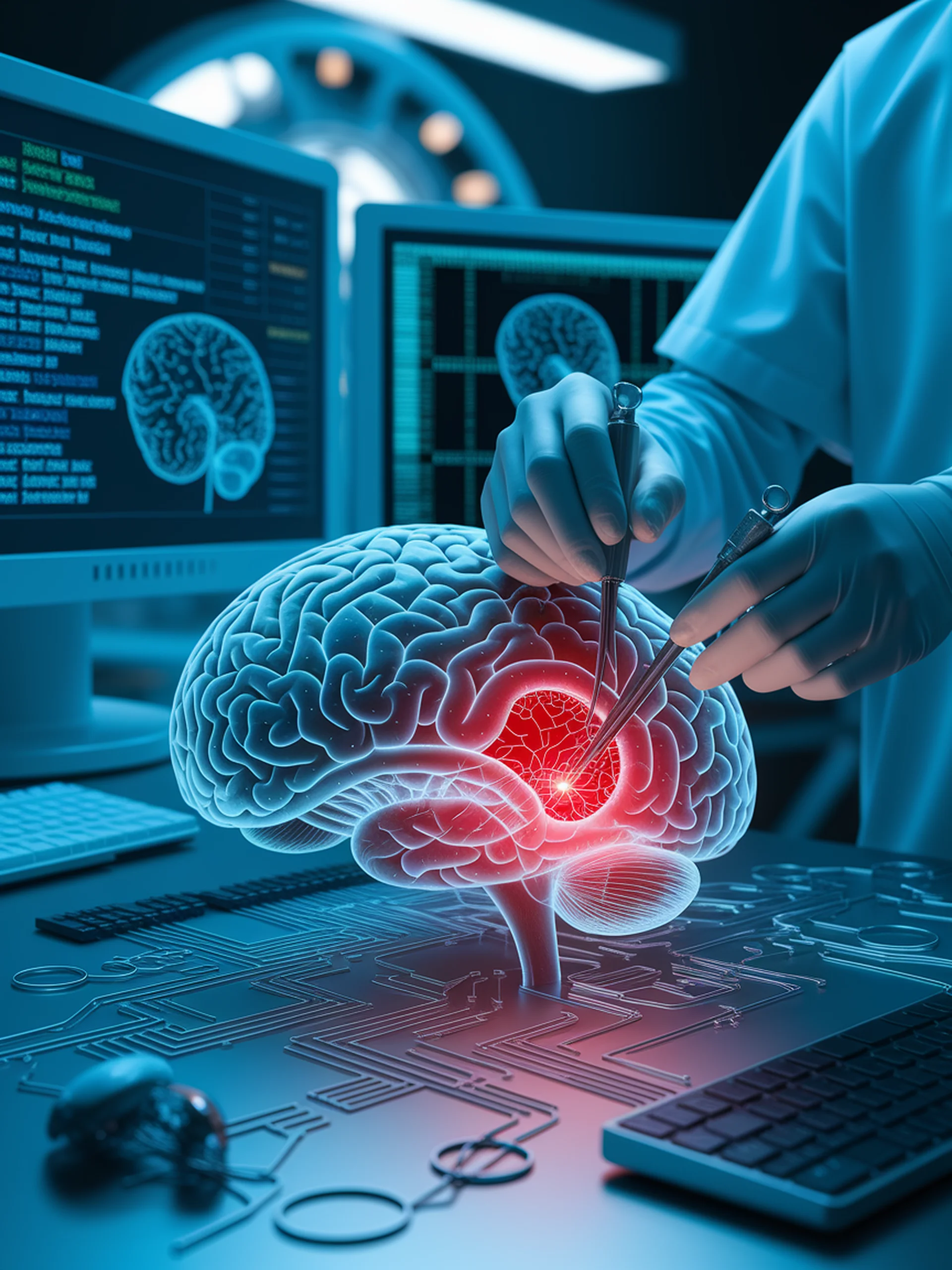
Surgical Knowledge Removal in LLMs
New technique to selectively unlearn harmful information from AI models
Researchers have developed a novel method to selectively remove dangerous knowledge from Large Language Models while preserving their general functionality.
- Uses Conditional Sparse Autoencoder Clamping to target specific harmful knowledge areas
- Successfully reduces model capabilities in dangerous domains like bioweapons and cyberattacks
- Maintains model performance on general tasks, avoiding comprehensive degradation
- Addresses critical security concerns about AI systems with dangerous knowledge
This advancement offers a practical solution for AI safety, enabling developers to create models that can't be misused for harmful purposes even when compromised or manipulated.
Original Paper: Don't Forget It! Conditional Sparse Autoencoder Clamping Works for Unlearning