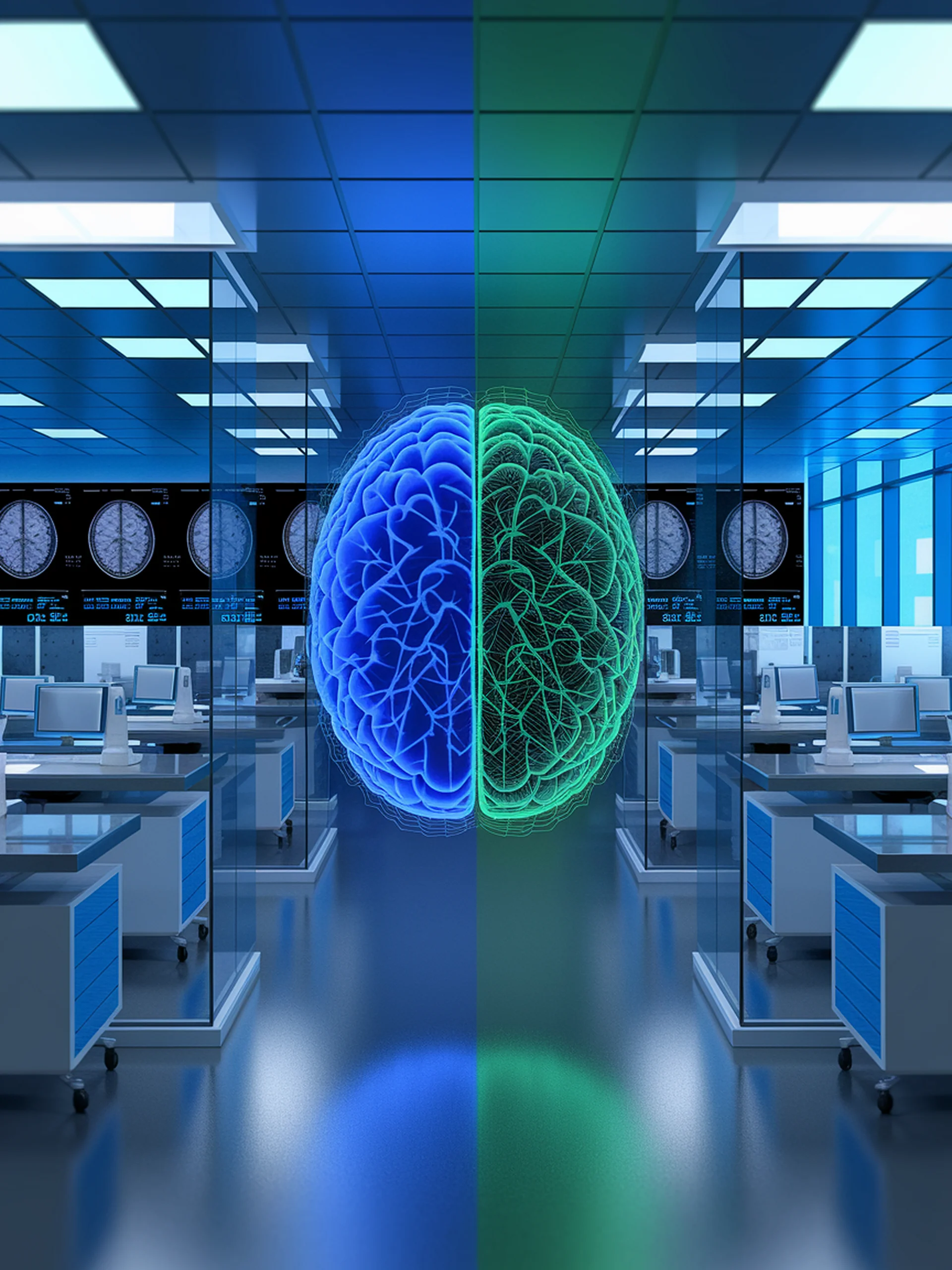
Preserving Safety in Fine-Tuned LLMs
A selective layer merging approach that maintains alignment while optimizing for tasks
SafeMERGE is a novel framework that addresses the critical challenge of safety erosion when fine-tuning large language models for specific applications.
- Selectively merges layers between fine-tuned and safety-aligned models based on cosine similarity measures
- Preserves safety alignment while maintaining task performance
- Outperforms existing methods like PEFT and full fine-tuning in safety-utility balance
- Requires no additional training or special fine-tuning procedures
For medical applications, this approach enables safer deployment of specialized LLMs that maintain ethical guardrails while providing domain-specific expertise, reducing potential harm from biased or dangerous outputs in healthcare settings.