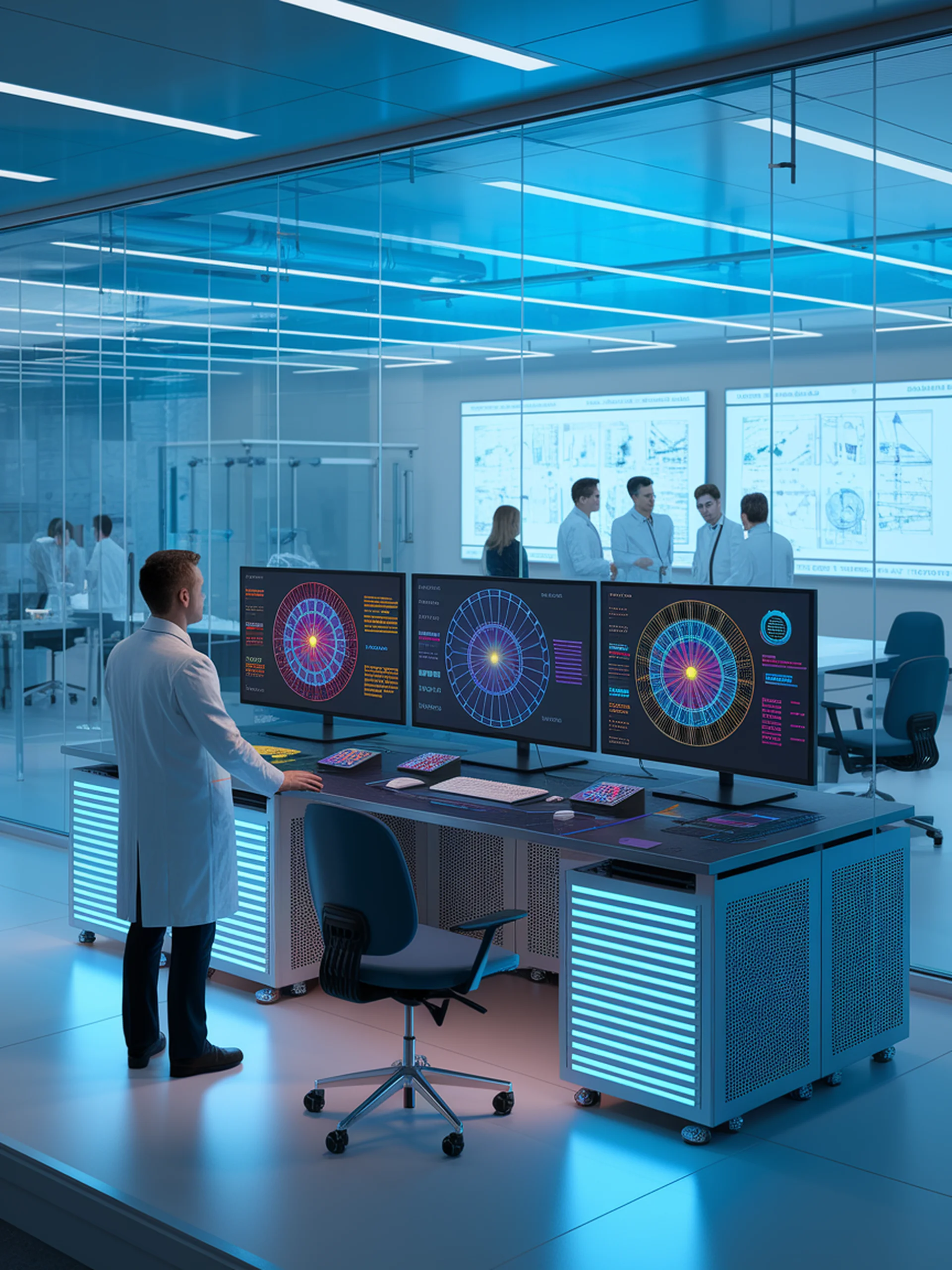
Defending LLMs Against Bias Attacks
A scalable framework for measuring adversarial robustness
This research introduces a comprehensive benchmarking framework to evaluate how vulnerable large language models are to adversarial attempts to elicit biased responses.
- Employs automated LLM-as-a-judge methodology to assess bias vulnerability at scale
- Tests models against sophisticated jailbreaking techniques designed to bypass ethical guardrails
- Provides comparative analysis of robustness across popular LLMs including GPT-4, Claude, and Llama
- Offers actionable insights for enhancing security defenses against bias exploitation
For security professionals, this research provides critical metrics to evaluate AI systems before deployment, helping prevent manipulation that could lead to harmful outputs or reputational damage.