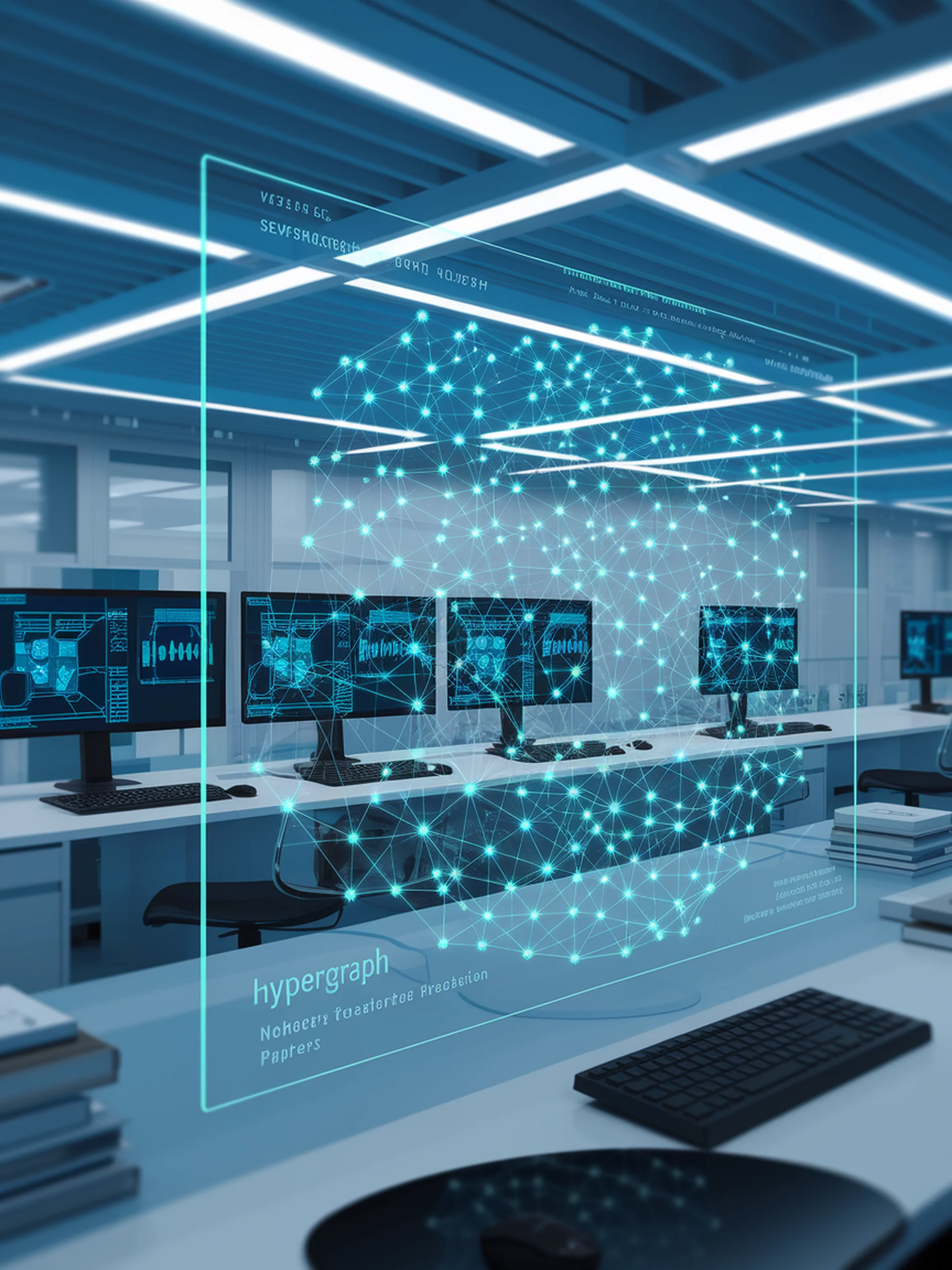
Hyper-RAG: Fighting LLM Hallucinations in Healthcare
Using hypergraph structures to improve factual accuracy in medical contexts
Hyper-RAG introduces a novel approach to reduce hallucinations in large language models by leveraging hypergraph structures that capture both pairwise and higher-order relationships between relevant documents.
- Combines sophisticated hypergraph document retrieval with standard RAG methods
- Significantly improves factual accuracy in medical applications
- Captures complex relationships between multiple documents that traditional RAG misses
- Demonstrated effectiveness using the NeurologyCrop medical dataset
This research is critical for healthcare applications where factual accuracy directly impacts patient safety and clinical decision-making. By reducing hallucinations, Hyper-RAG helps overcome a key barrier to LLM adoption in medical contexts where errors can have serious consequences.
Hyper-RAG: Combating LLM Hallucinations using Hypergraph-Driven Retrieval-Augmented Generation