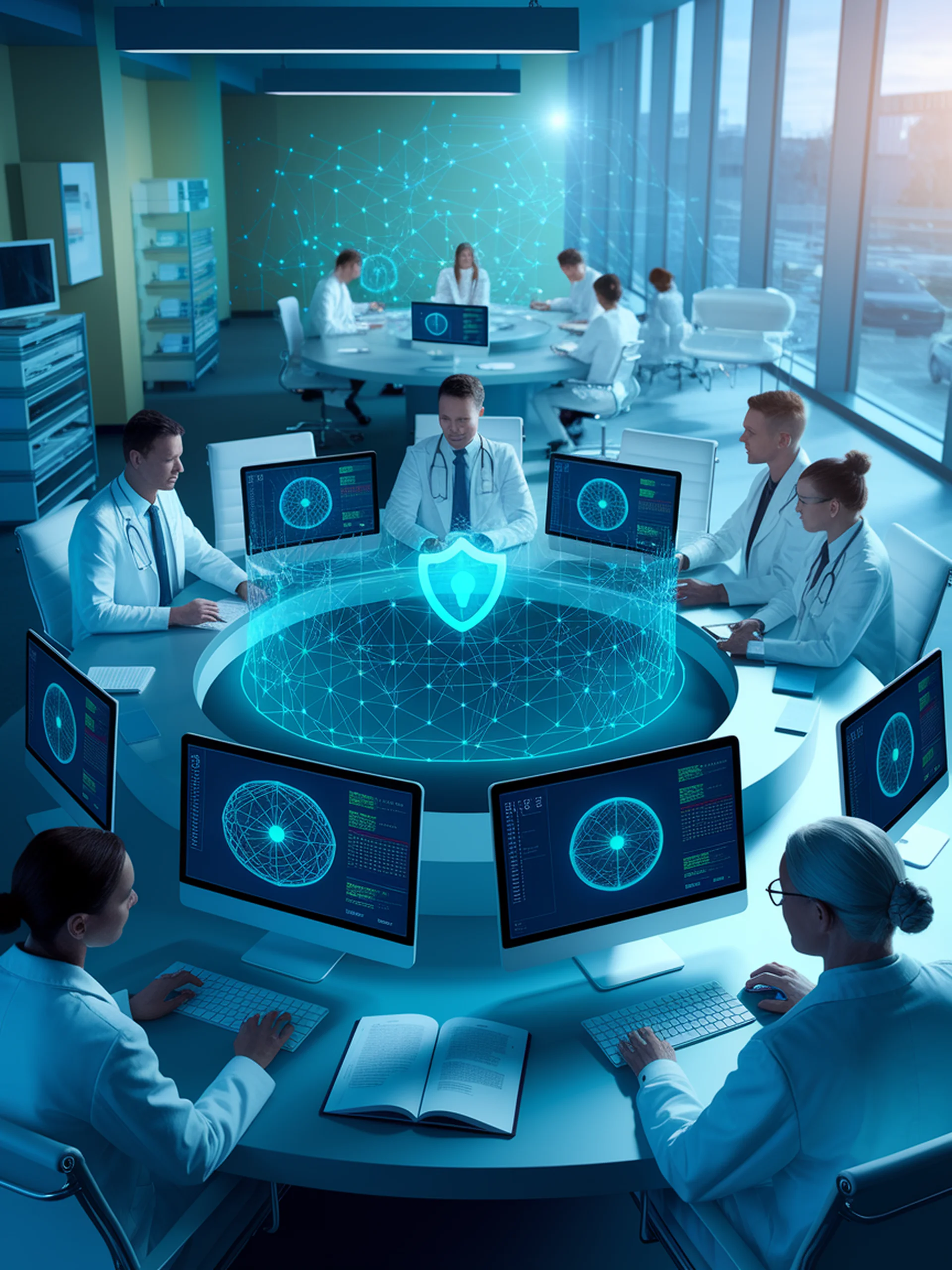
Smarter Federated Learning for Healthcare NLP
Training Large Language Models Efficiently While Preserving Privacy
This research introduces Layer-Skipping Federated Learning to efficiently train large language models across healthcare organizations without compromising patient privacy.
- Selectively fine-tunes only specific layers of pre-trained LLMs, reducing computational and communication costs
- Addresses key challenges in healthcare NLP: privacy concerns, communication overhead, and data heterogeneity
- Demonstrates effectiveness on clinical tasks using i2b2 and MIMIC-III datasets
- Maintains model performance while significantly improving training efficiency
This innovation enables healthcare organizations to collaboratively build powerful NLP models for clinical applications while keeping sensitive patient data secure and private.