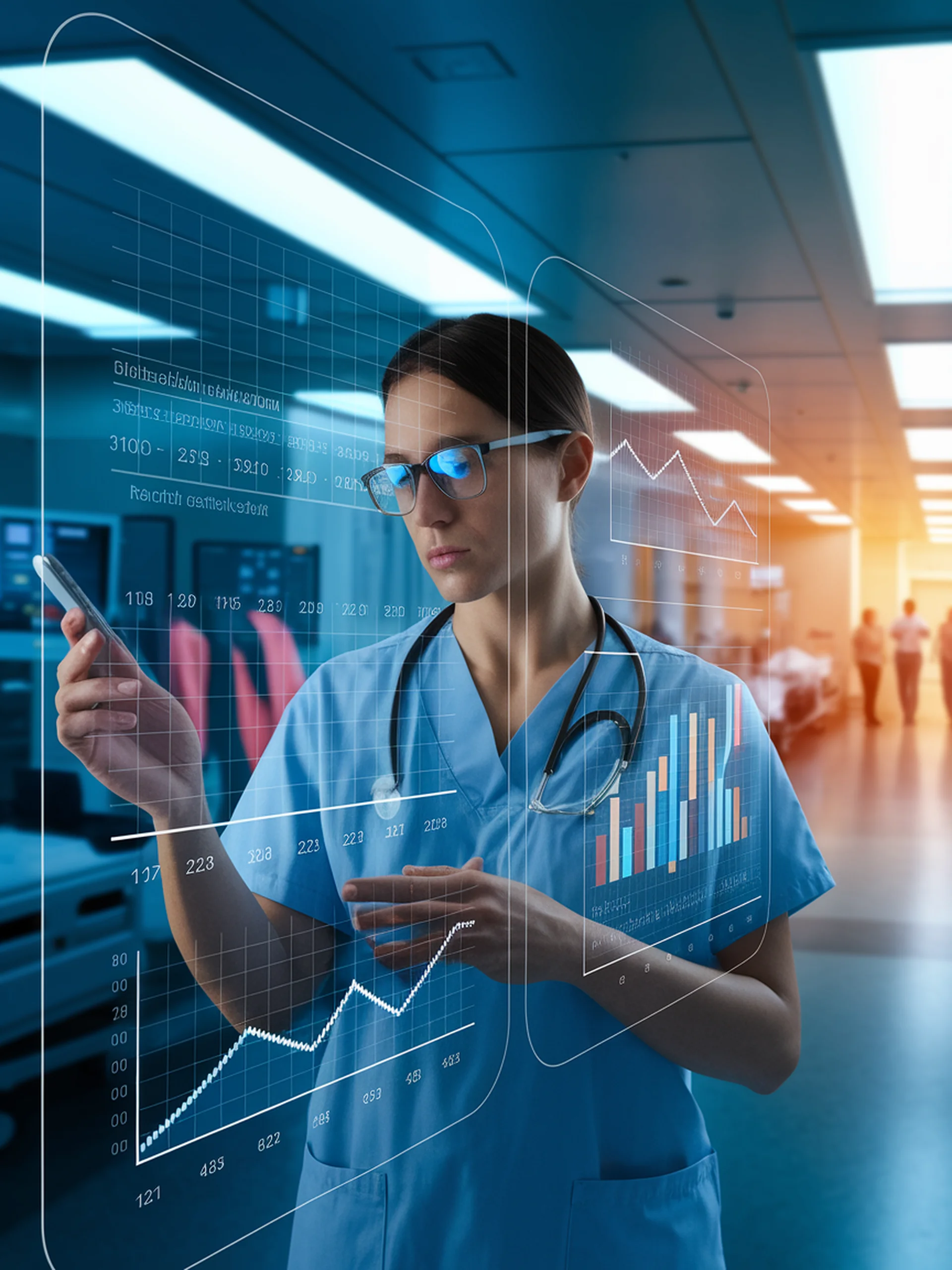
Can We Trust LLMs in High-Stakes Environments?
Enhancing reliability through uncertainty quantification
This research explores methods for making large language models more trustworthy in critical applications by accurately measuring their confidence levels.
- LLMs often produce plausible but incorrect responses, creating significant risks in healthcare, legal, and other high-stakes domains
- Uncertainty quantification (UQ) helps estimate confidence in LLM outputs, enabling better risk management
- Traditional UQ methods face challenges with modern LLMs due to their scale and complexity
- Effective confidence calibration is especially critical in medical applications where incorrect AI recommendations could impact patient safety and treatment outcomes
Uncertainty Quantification and Confidence Calibration in Large Language Models: A Survey