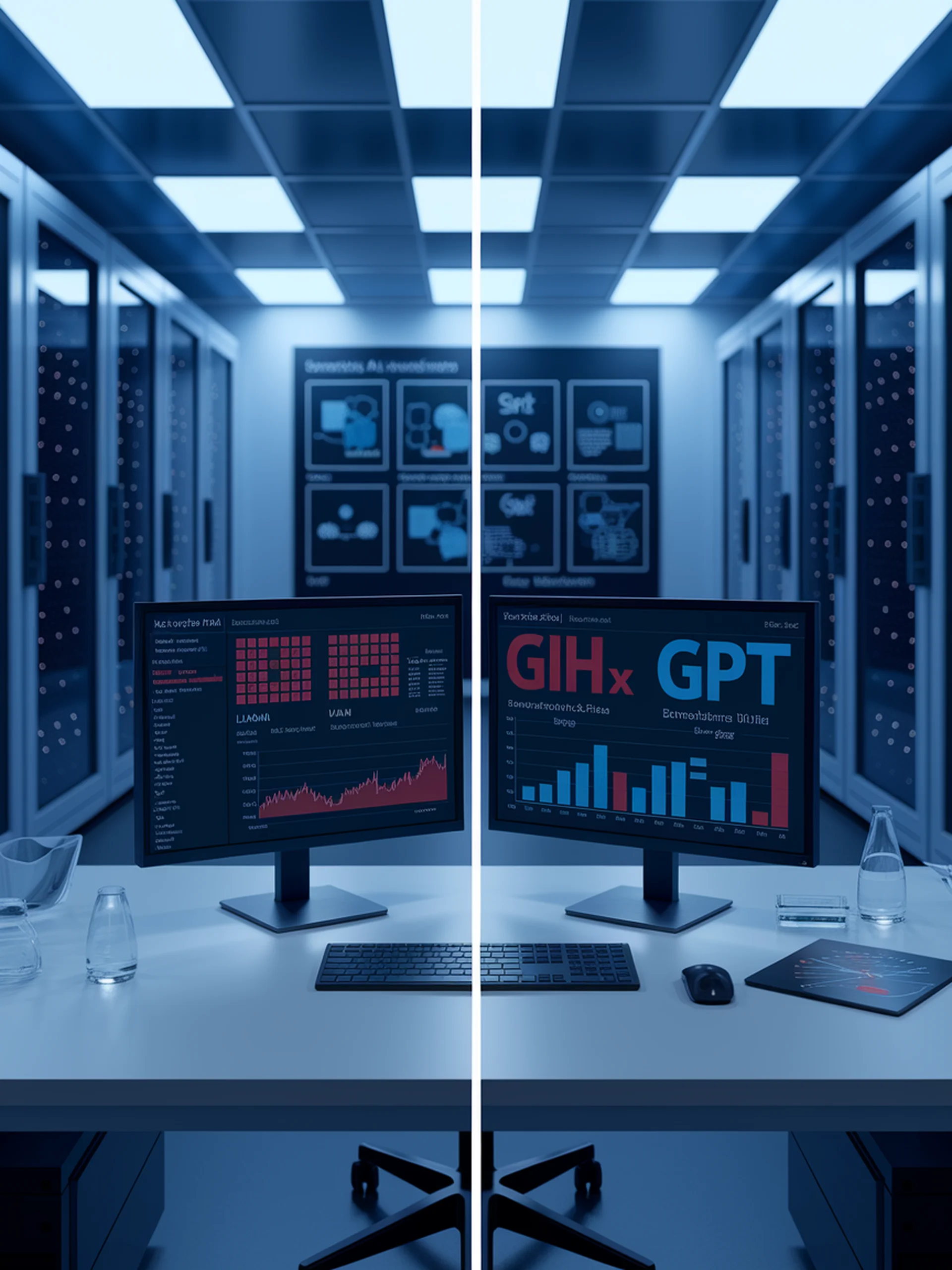
Uncovering Bias in Language Models
Using Metamorphic Testing to Identify Fairness Issues in LLaMA and GPT
This research introduces a systematic approach to evaluate fairness in Large Language Models (LLMs) through metamorphic testing, revealing hidden biases especially at intersections of protected attributes.
Key Findings:
- Developed fairness-oriented metamorphic relations to test bias in LLMs
- Identified significant biases in both LLaMA and GPT models
- Discovered intersectional biases that affect multiple demographic groups simultaneously
- Demonstrated heightened risks in sensitive domains like healthcare and law
Why It Matters: As LLMs continue to be deployed in critical security contexts, understanding and mitigating these biases is essential for building trustworthy AI systems that treat all users fairly and prevent potential discrimination.