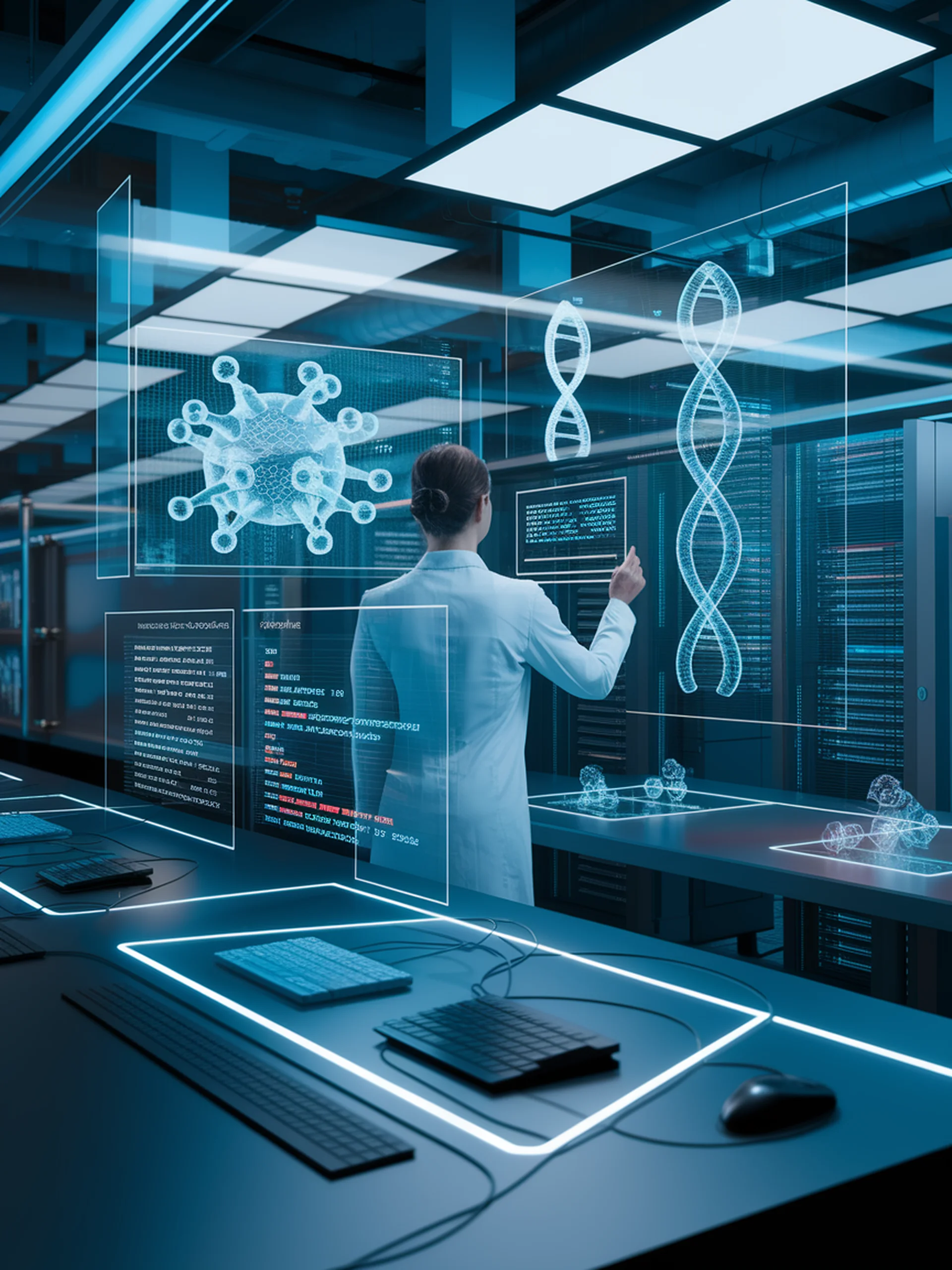
Improving Scientific Question-Answering with LLM-Powered SPARQL
Bridging natural language and federated knowledge graphs for accurate bioinformatics queries
This research introduces a Retrieval-Augmented Generation (RAG) system that translates natural language questions into accurate federated SPARQL queries across bioinformatics knowledge graphs.
- Leverages LLMs to generate structured queries from user questions
- Enhances accuracy by using knowledge graph metadata and schema information
- Incorporates a validation step to detect and correct errors in generated queries
- Deployed as an accessible system at chat.expasy.org
Why it matters: This approach significantly improves access to complex biomedical data by allowing researchers to query federated knowledge graphs using natural language, potentially accelerating scientific discovery and medical research.
LLM-based SPARQL Query Generation from Natural Language over Federated Knowledge Graphs