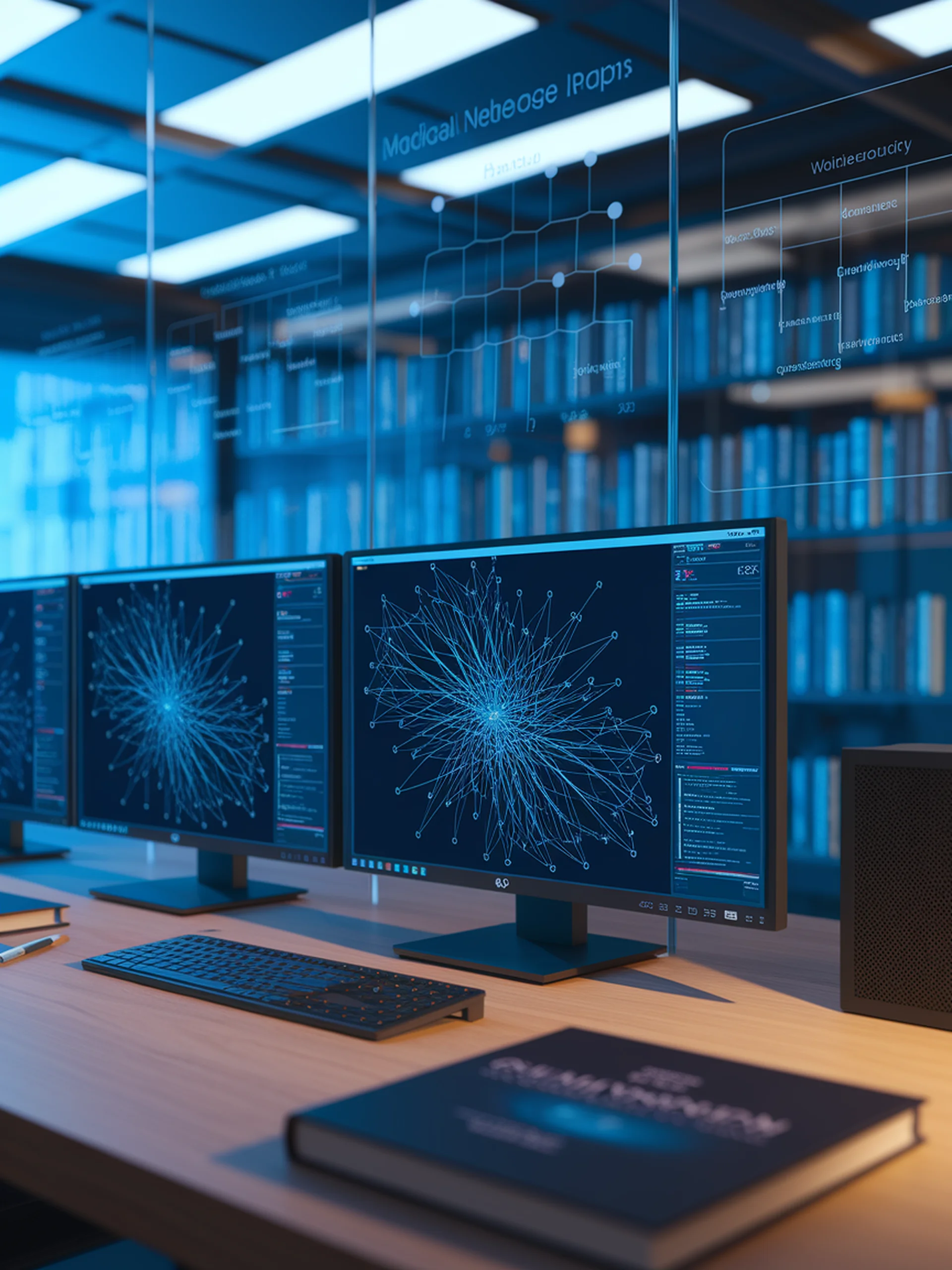
Enhancing LLMs for Long-Tail Knowledge
Dynamic Uncertainty Ranking for Specialized Domain Questions
This research introduces a novel approach to help large language models better handle specialized knowledge that appears rarely in training data.
Key Findings:
- Introduces Dynamic Uncertainty Ranking to improve retrieval-augmented in-context learning
- Enhances LLM performance on specialized domain questions without extensive retraining
- Reduces reliance on pre-trained data memorization for answering long-tail questions
- Demonstrates practical applications across specialized domains including medical knowledge
Medical Significance: This approach enables more reliable medical information retrieval in LLMs by dynamically identifying and addressing uncertainty in specialized medical knowledge—critical for healthcare applications where accuracy and comprehensive knowledge are paramount.