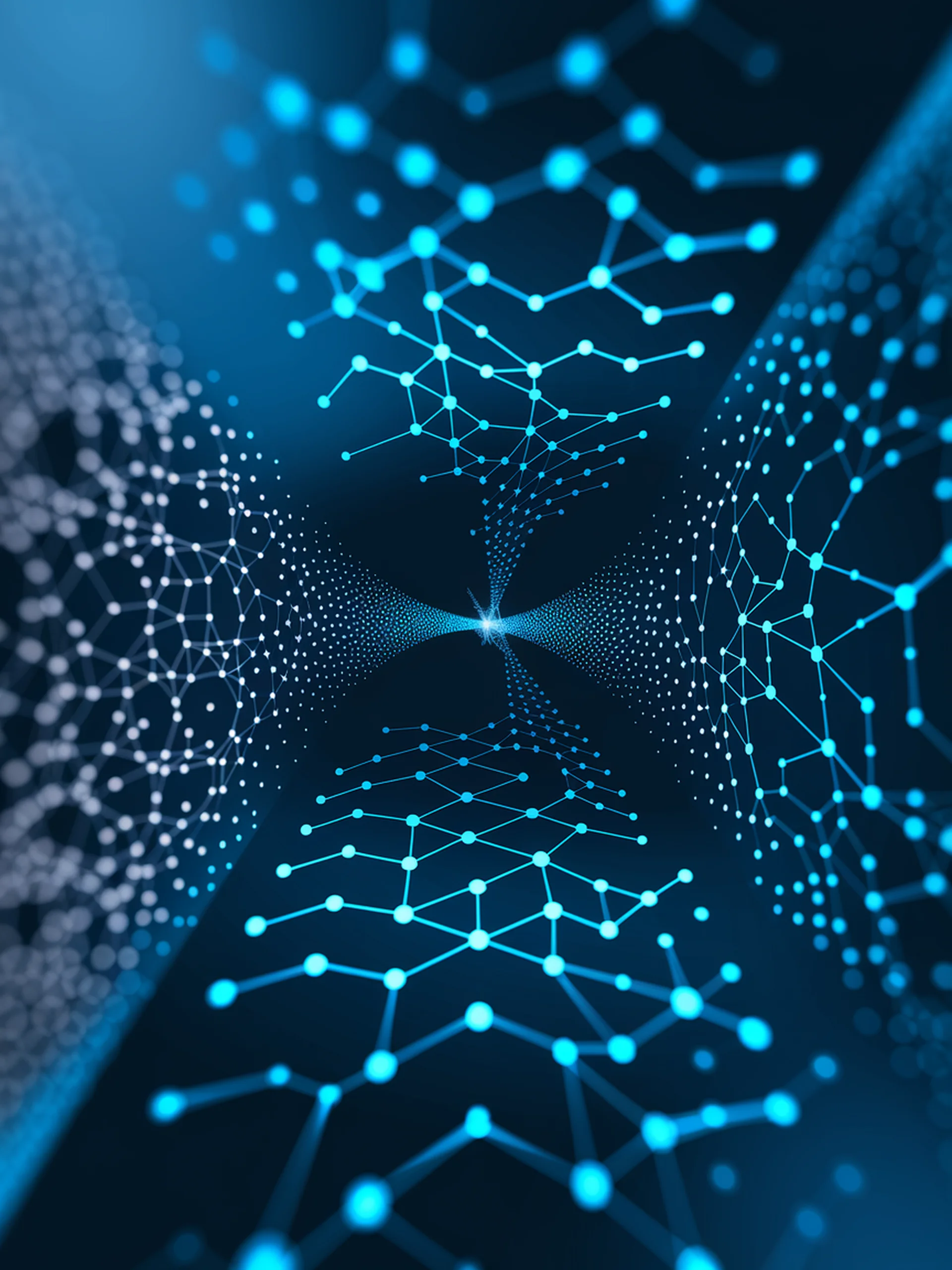
Transforming Graphs for Universal Learning
LLMs as converters for cross-domain graph analysis
This research explores how Large Language Models can convert standard graphs into text-attributed graphs (TAGs), enabling powerful cross-domain analysis previously hindered by feature compatibility issues.
- Solves the challenge of analyzing graphs with different feature spaces
- Leverages LLMs to create universally compatible text-attributed nodes
- Enables transfer learning across diverse graph domains
- Particularly valuable for cross-domain applications like drug discovery
Medical Impact: By enabling more effective cross-domain graph analysis, this approach could significantly accelerate drug discovery processes, allowing researchers to transfer insights between molecular structures and identify novel therapeutic candidates more efficiently.