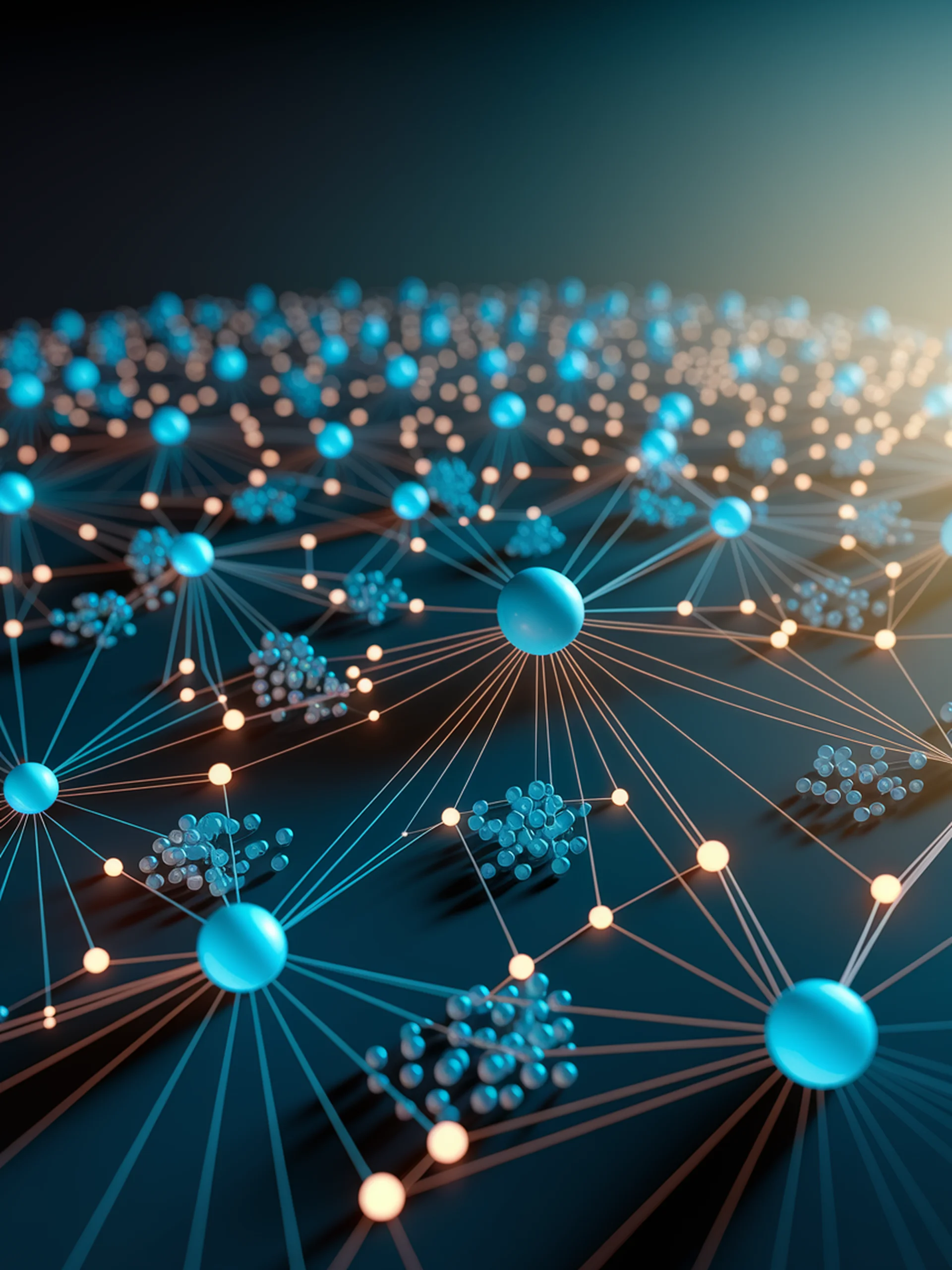
Enhancing Knowledge Retrieval with Graph-RAG
A cost-efficient multi-granular approach for complex domains
KET-RAG introduces a novel framework that enhances retrieval-augmented generation by constructing knowledge graphs from text chunks, enabling more precise multi-hop reasoning.
- Combines entity-level and chunk-level indexing to capture fine-grained relationships between concepts
- Particularly valuable for domain-specific knowledge requiring complex reasoning paths
- Offers cost-efficient implementation compared to existing Graph-RAG approaches
- Excels in connecting information across multiple documents or sections
In medical contexts, this approach significantly improves information retrieval for complex clinical questions that require connecting findings, treatments, and medical concepts across different documents—essential for evidence-based medicine and research applications.
KET-RAG: A Cost-Efficient Multi-Granular Indexing Framework for Graph-RAG