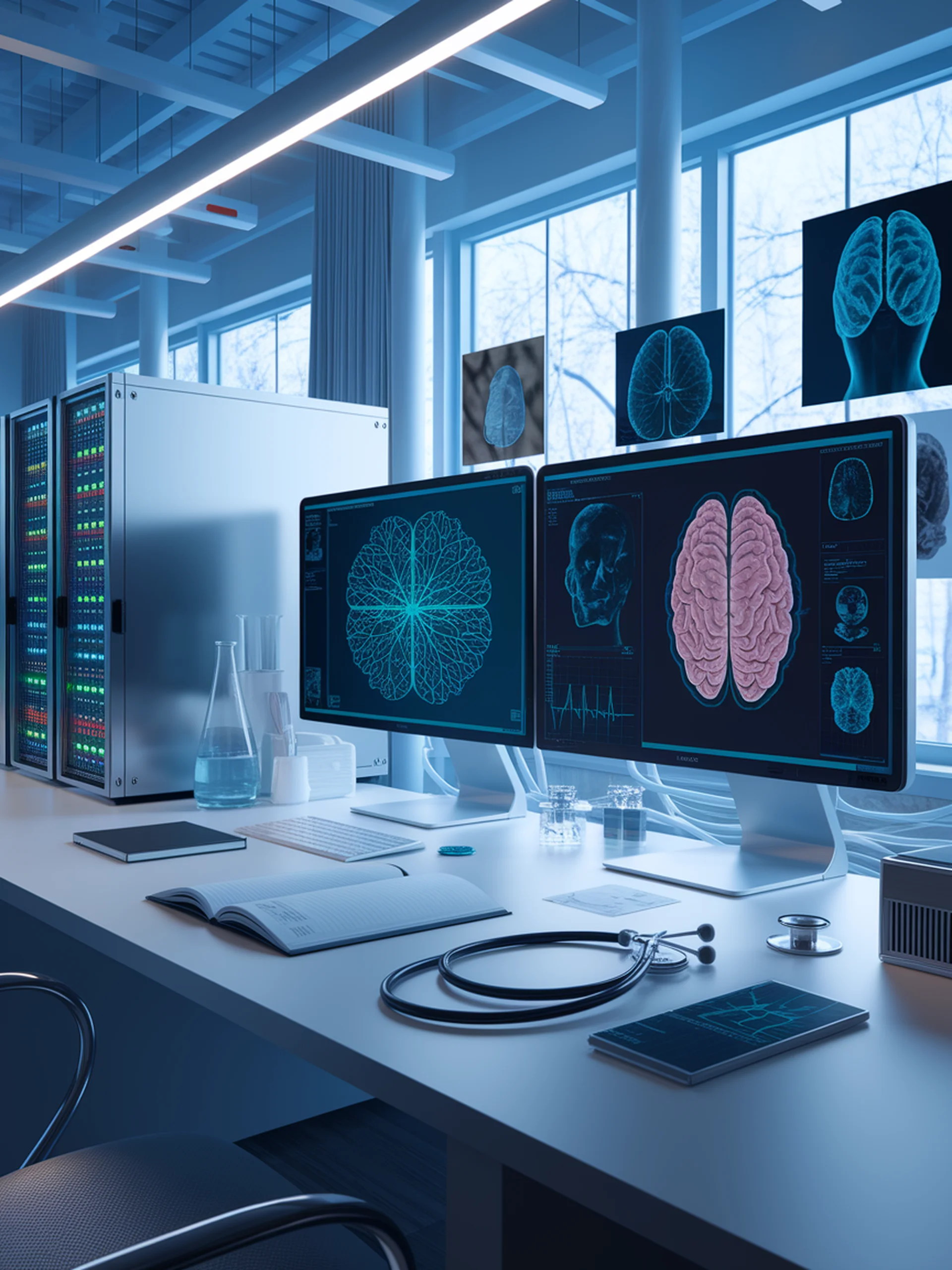
Self-Adaptive Continual Learning for LLMs
Automating knowledge selection and retention in domain-specific contexts
This research introduces a framework for automatic continual instruction tuning that enables LLMs to learn incrementally while intelligently selecting new knowledge and preserving existing capabilities.
- Dynamically filters incoming data to identify valuable new information
- Maintains model performance on previous tasks while learning new skills
- Implements automatic version rollback and checkpoint evaluation for quality control
- Demonstrates effectiveness in real-world medical scenarios where high-quality, reliable information is critical
For healthcare applications, this approach enables medical AI systems to continuously adapt to new medical knowledge, protocols, and treatments while maintaining accuracy on established medical information—addressing a critical challenge in deploying trustworthy AI in healthcare settings.
Towards Automatic Continual Learning: A Self-Adaptive Framework for Continual Instruction Tuning