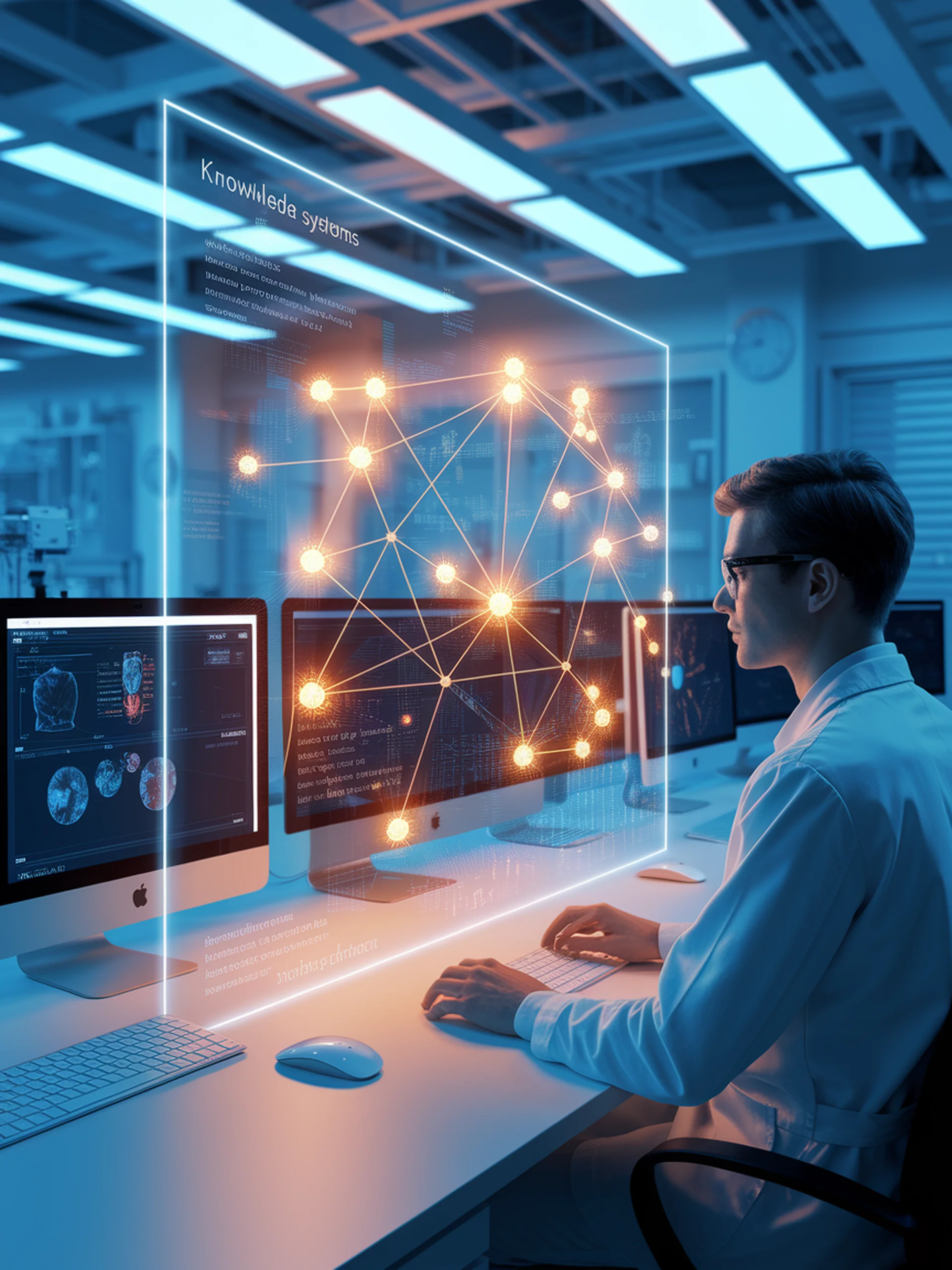
LLMs for Medical Knowledge Completion
Evaluating how large language models can fill gaps in medical knowledge graphs
This research investigates how Large Language Models (LLMs) can address incompleteness in medical knowledge graphs, particularly for disease-treatment mappings.
- LLMs can effectively impute missing relationships in medical knowledge graphs with 69.7% accuracy on treatment recommendations
- Different prompting strategies significantly impact performance, with chain-of-thought reasoning outperforming direct questioning
- Models show varying sensitivity to medical domains, with some excelling in rare diseases while others perform better with common conditions
- Evaluation reveals critical limitations in hallucination control and reasoning that require domain-specific safeguards
This research matters because incomplete medical knowledge graphs directly impact clinical decision support systems and research tools that healthcare professionals rely on daily.
Can LLMs Support Medical Knowledge Imputation? An Evaluation-Based Perspective