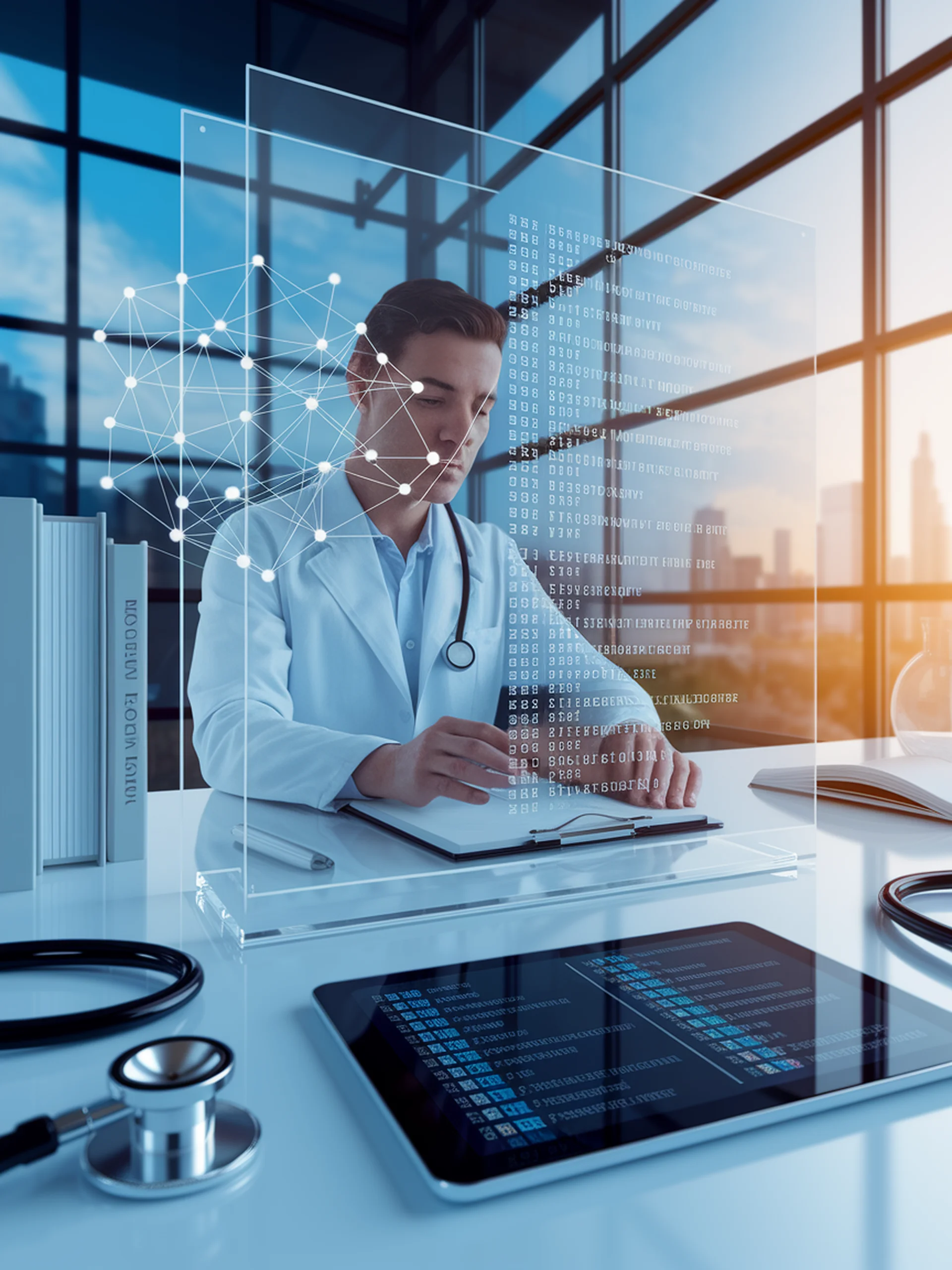
Transparent AI for Medical Coding
Enhancing Interpretability in Automated Medical Code Assignment
This research introduces a novel dictionary learning approach that improves transparency in how large language models (LLMs) assign medical codes to clinical text, addressing a critical healthcare need.
- Developed a transparent architecture that identifies relevant clinical concepts beyond simple token attention
- Demonstrated improved interpretability while maintaining high performance in medical code assignment
- Created a more trustworthy AI solution for healthcare professionals by revealing the reasoning behind automated coding decisions
This matters because trustworthy, explainable AI is essential for clinical adoption, regulatory compliance, and reducing the burden of manual medical coding while maintaining accuracy and patient safety.