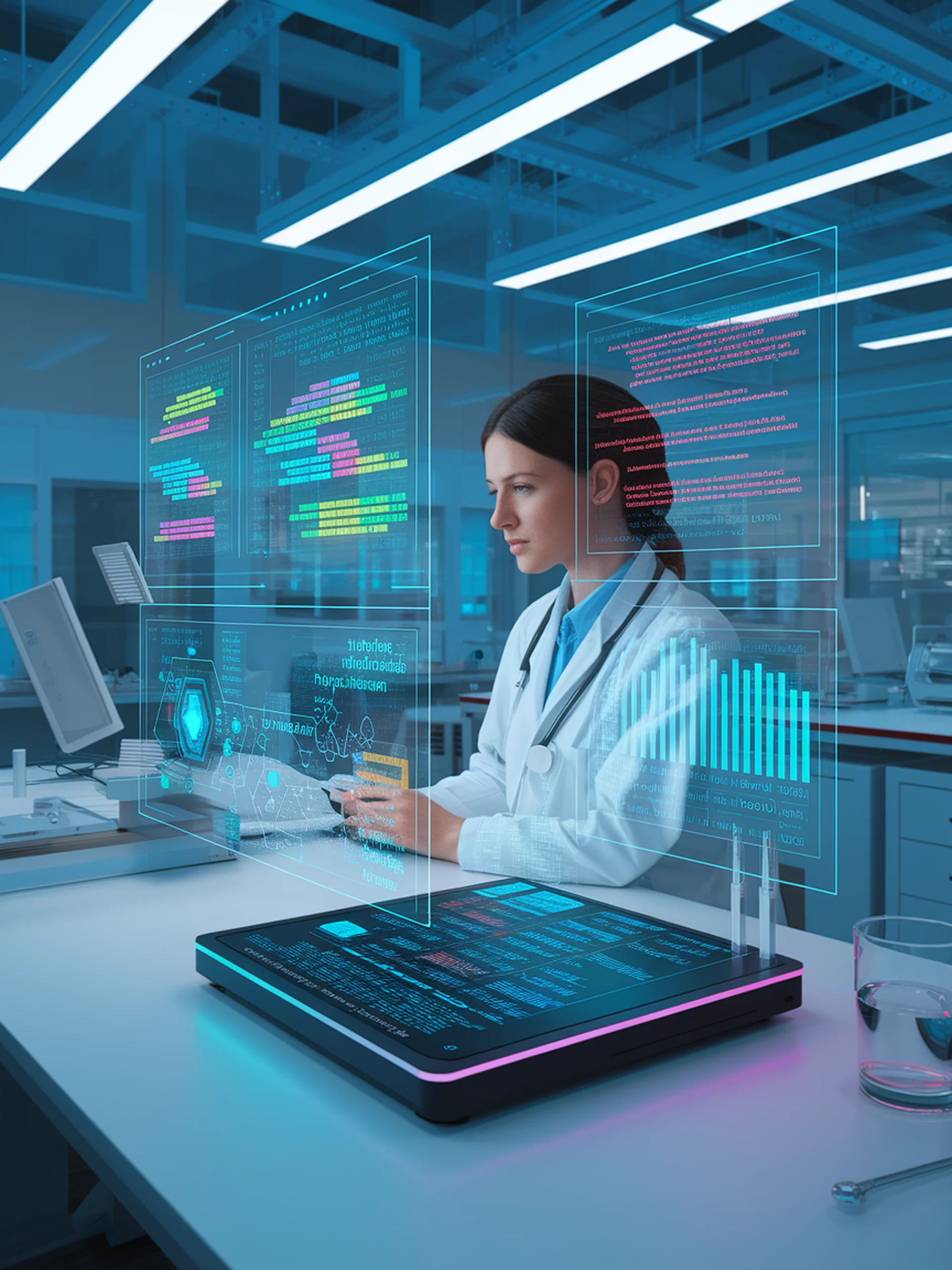
Boosting NLP in Low-Resource Medical Settings
Using Pseudo-Annotations to Enhance Named Entity Detection with LLMs
PICLe introduces a novel approach for medical text analysis when labeled data is scarce, using LLMs to generate pseudo-annotations for in-context learning.
- Enables effective Named Entity Detection in biomedical texts with minimal labeled examples
- Demonstrates that the quality of in-context examples significantly impacts model performance
- Provides a practical solution for low-resource medical NLP applications where obtaining labeled data is challenging
- Evaluated across five biomedical datasets with consistent performance gains
This research offers practical value for healthcare organizations looking to implement NLP solutions when facing data limitations or privacy constraints in specialized medical domains.