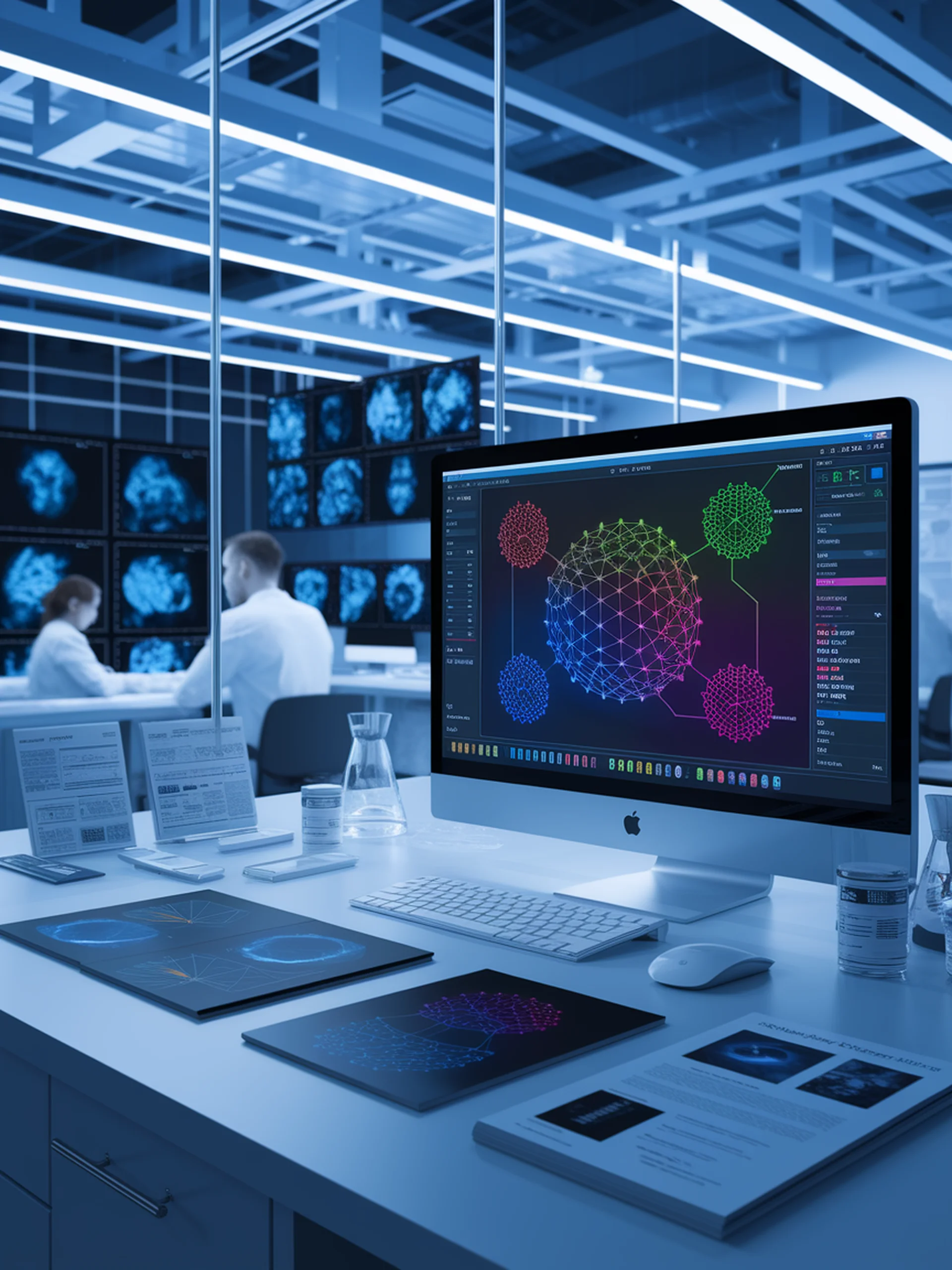
Smarter Medical NLP with Multi-Mode Retrieval
Enhancing LLM performance in biomedical contexts through strategic example selection
This research introduces MMRAG (Multi-Mode Retrieval-Augmented Generation), a framework that significantly improves large language model performance on biomedical tasks by optimizing in-context example selection.
- Combines four complementary retrieval strategies (Random, Top, Diversity, and Class modes) to select the most effective examples
- Achieves 31.4% average improvement over standard retrieval methods across multiple biomedical datasets
- Demonstrates that strategic example selection is more impactful than simply increasing the number of examples
- Provides a flexible framework that adapts to different biomedical NLP tasks
This advancement matters for healthcare because it enables more accurate and reliable AI applications in medical contexts without requiring expensive model retraining or fine-tuning.