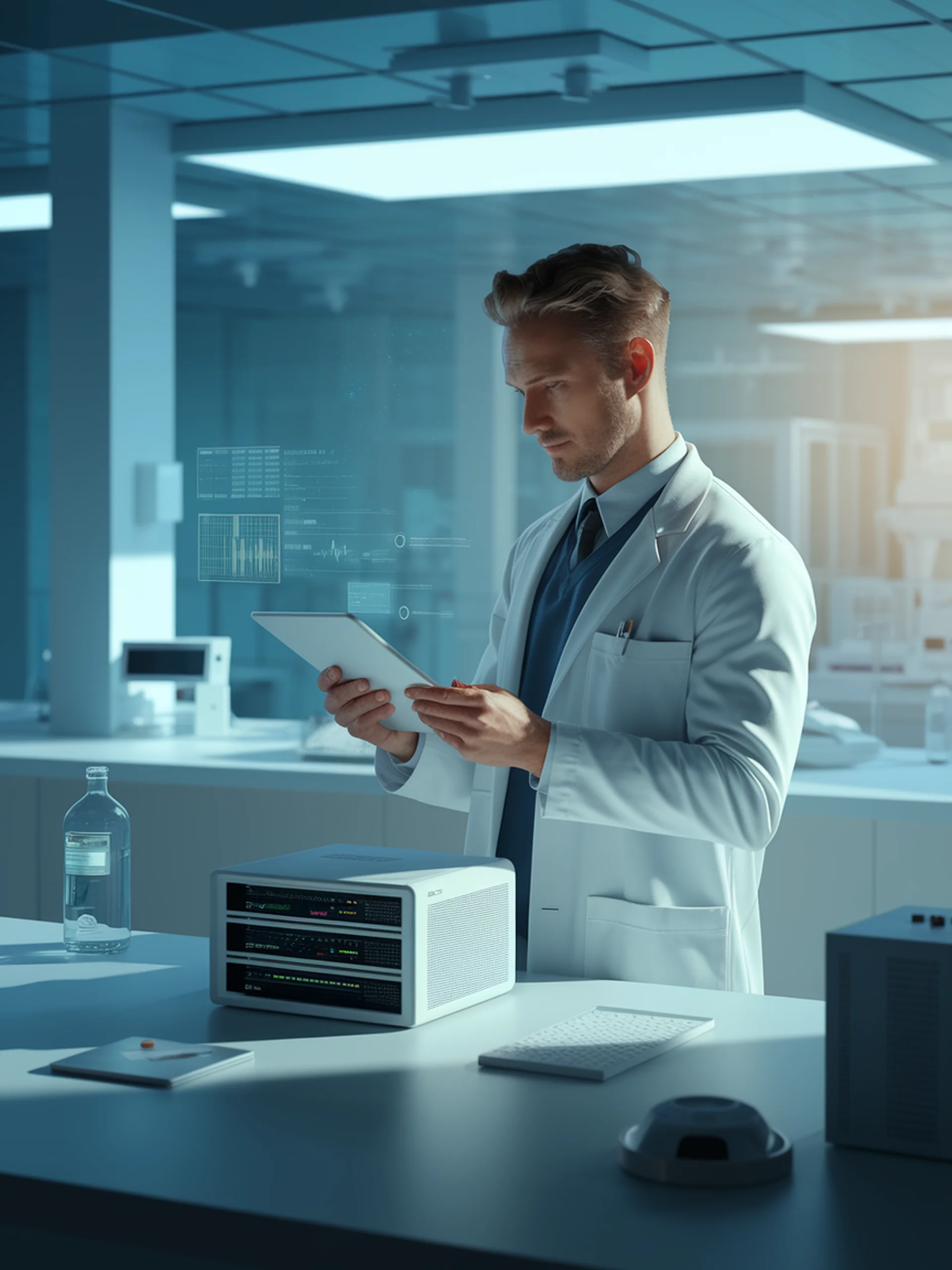
Maximizing Medical AI with Limited Data
Fine-tuning small LLMs achieves strong results on specialized medical tasks
This research demonstrates that smaller language models fine-tuned on limited medical datasets can achieve performance comparable to larger models for clinical text processing tasks.
- Fine-tuning improves performance on both text classification and entity recognition in medical contexts
- Notable performance gains achieved with as few as 100-200 training examples
- Local deployment of smaller models offers practical advantages for clinical workflows
- Results suggest healthcare institutions can effectively leverage AI without massive datasets or compute resources
This work matters for healthcare providers seeking to implement AI solutions with limited training data while maintaining patient privacy and operational efficiency.