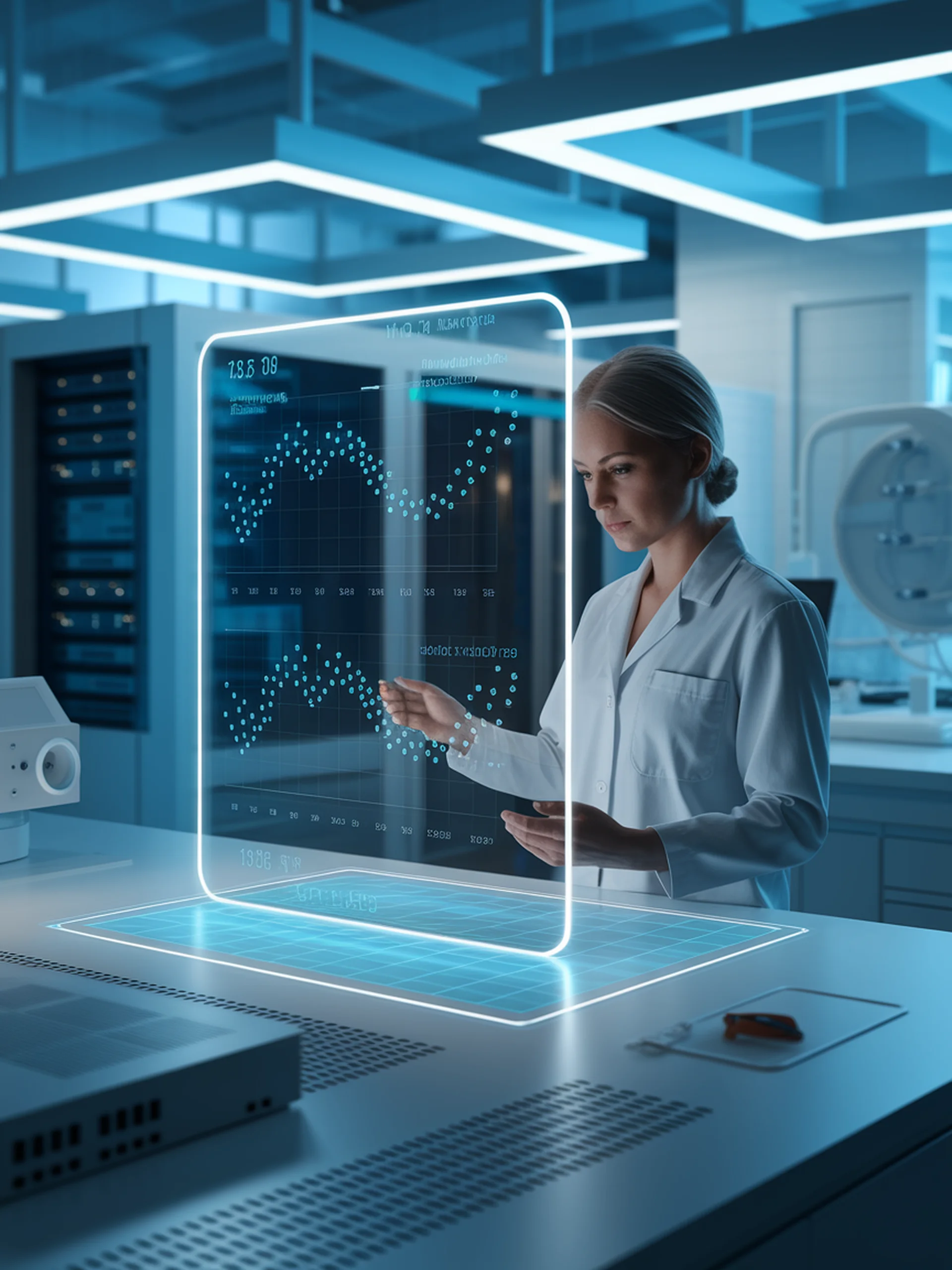
Harnessing LLMs for Quantitative Knowledge
Novel framework for extracting numerical data from language models
This research establishes a framework for reliably extracting quantitative information from LLMs to support Bayesian modeling and data imputation tasks.
- Evaluates LLMs as sources of numerical data and probability distributions
- Introduces methods to enhance Bayesian workflows through LLM-assisted prior elicitation
- Demonstrates applications for missing data imputation with numerical precision
- Provides a systematic approach to quantify uncertainty in LLM-retrieved values
For medical applications, this framework enables more robust clinical predictions by leveraging LLMs to fill data gaps and improve Bayesian modeling of patient outcomes, offering a powerful new approach to healthcare analytics where complete data is often unavailable.
Had enough of experts? Quantitative knowledge retrieval from large language models