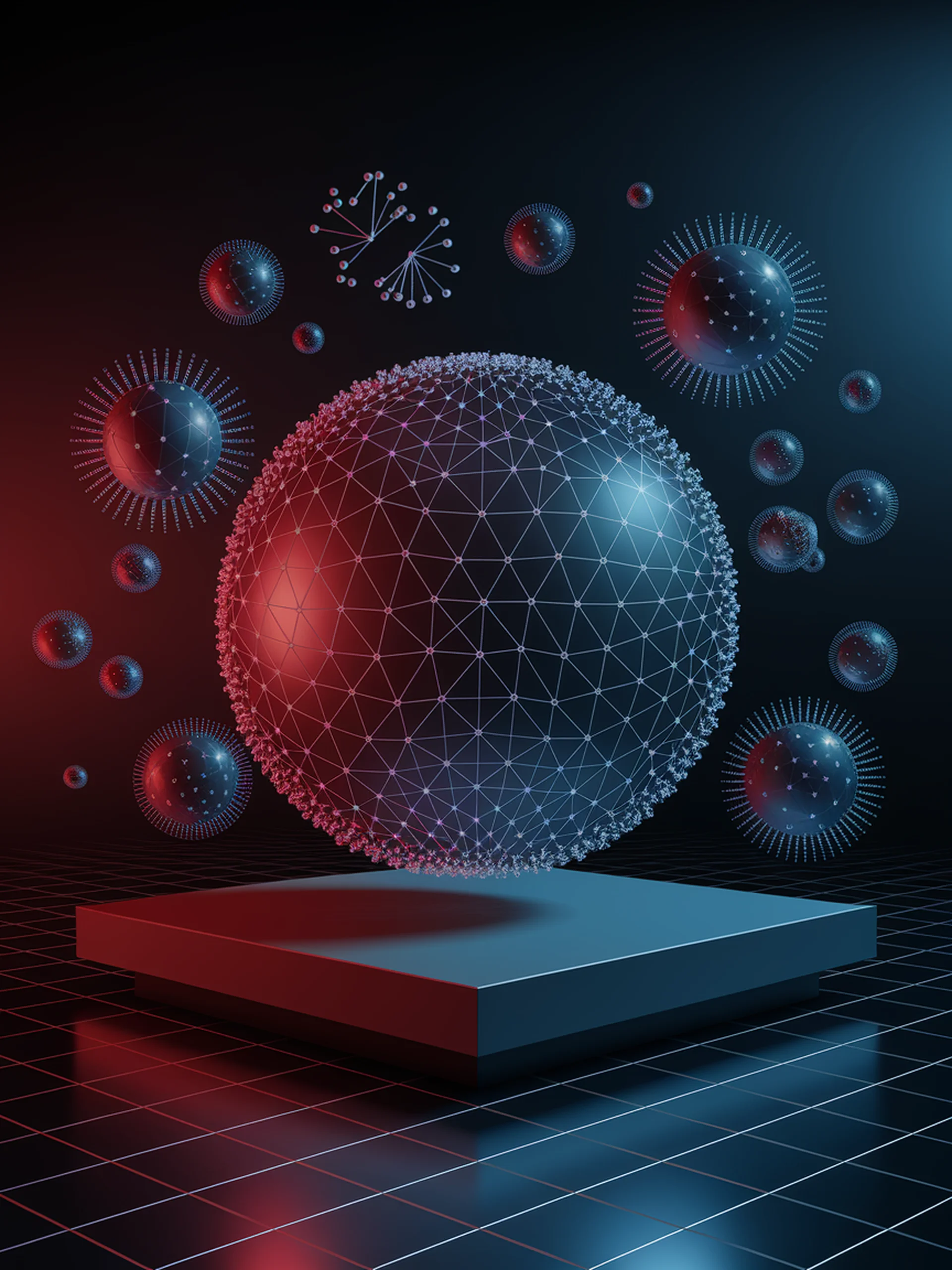
Quantum Computing Meets LLMs
Overcoming LoRA's Limitations with Quantum-Enhanced Fine-Tuning
This research introduces Quantum Weighted Tensor Hybrid Networks (QWTHN), a novel approach that leverages quantum computing principles to enhance the fine-tuning capabilities of large language models beyond classical LoRA methods.
- Addresses the expressive bottleneck of traditional low-rank approximation techniques
- Significantly improves model adaptability for complex tasks and high-rank dependency scenarios
- Demonstrates how quantum computing techniques can be practically applied to enhance LLM performance
- Represents a promising direction for parameter-efficient fine-tuning at scale
This breakthrough matters for AI engineering by potentially enabling more efficient adaptation of large models to specialized domains without the computational overhead of full fine-tuning, while maintaining higher expressivity than current methods.