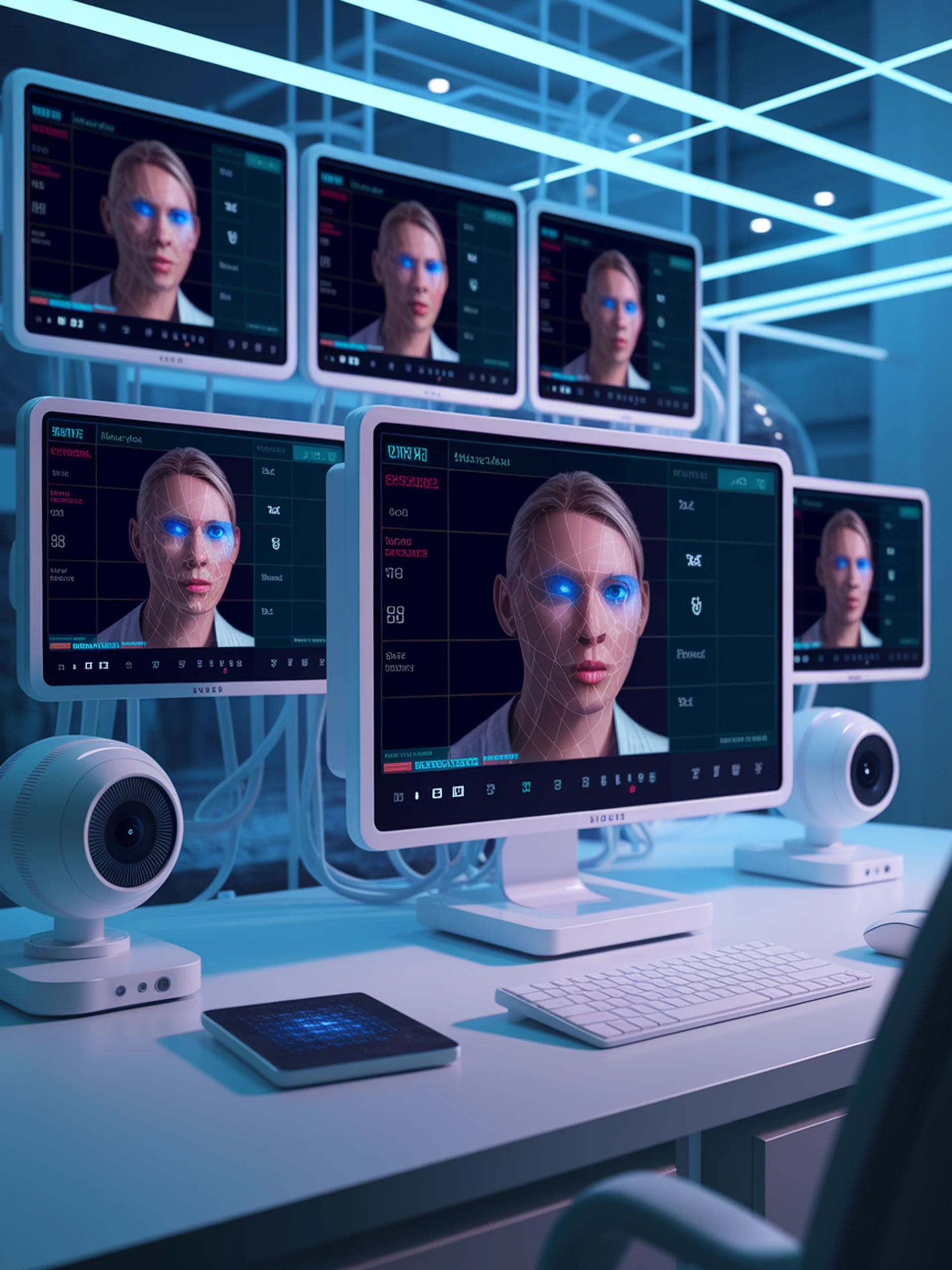
Privacy-Preserving Emotion AI
Advancing long-term emotion analysis with de-identification safeguards
This research introduces EALD-MLLM, a novel multi-modal framework that analyzes emotions in long-duration videos while protecting privacy through de-identification techniques.
- Addresses critical limitations in current emotion AI by focusing on long sequential videos that capture authentic emotional states rather than just momentary reactions
- Employs de-identification techniques that preserve emotional signals while removing personally identifiable information
- Leverages multi-modal large language models to process both visual and textual information for comprehensive emotion analysis
- Demonstrates superior performance in emotion recognition while maintaining privacy standards
This advancement has significant implications for security and privacy applications, enabling emotion-aware systems that can function without compromising user identities or storing sensitive biometric data.