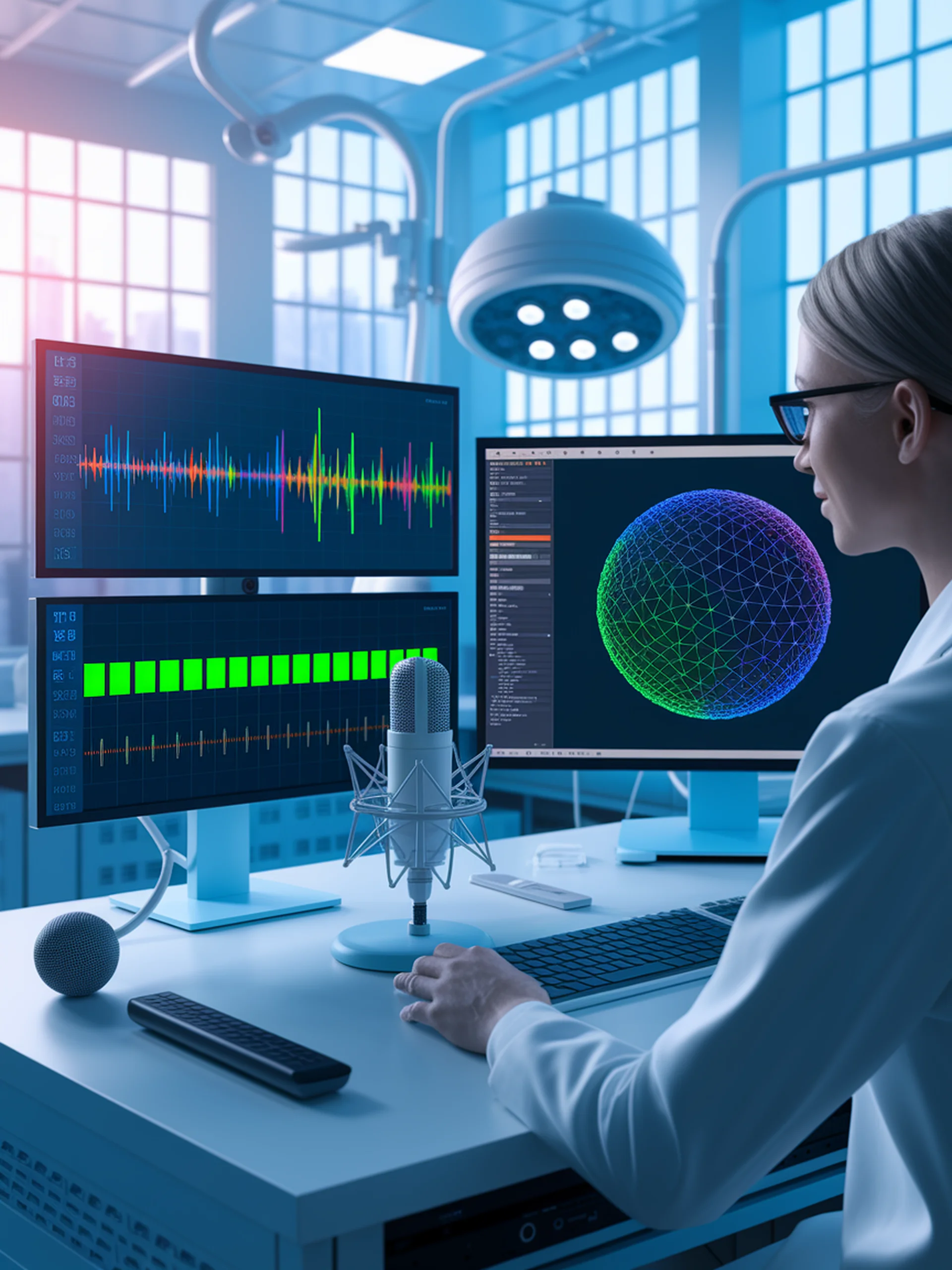
Enhancing Depression Detection with Speech Patterns
Leveraging speech timing data improves LLM diagnostic accuracy
This research introduces SpeechT-RAG, a novel approach that significantly improves LLMs' depression detection capabilities by incorporating speech timing information.
- Conventional text-based RAG systems show limited improvements in depression detection
- Speech timing features (pause duration, speech rate) contain critical depression markers missed in text
- SpeechT-RAG achieves 15.3% higher accuracy over standard LLM approaches
- Includes confidence estimation to improve clinical reliability
This advancement matters for mental health assessment by providing more accessible, accurate diagnostic tools that could expand screening capabilities while maintaining clinical relevance. The approach demonstrates how multimodal data integration can overcome limitations in text-only mental health analysis.