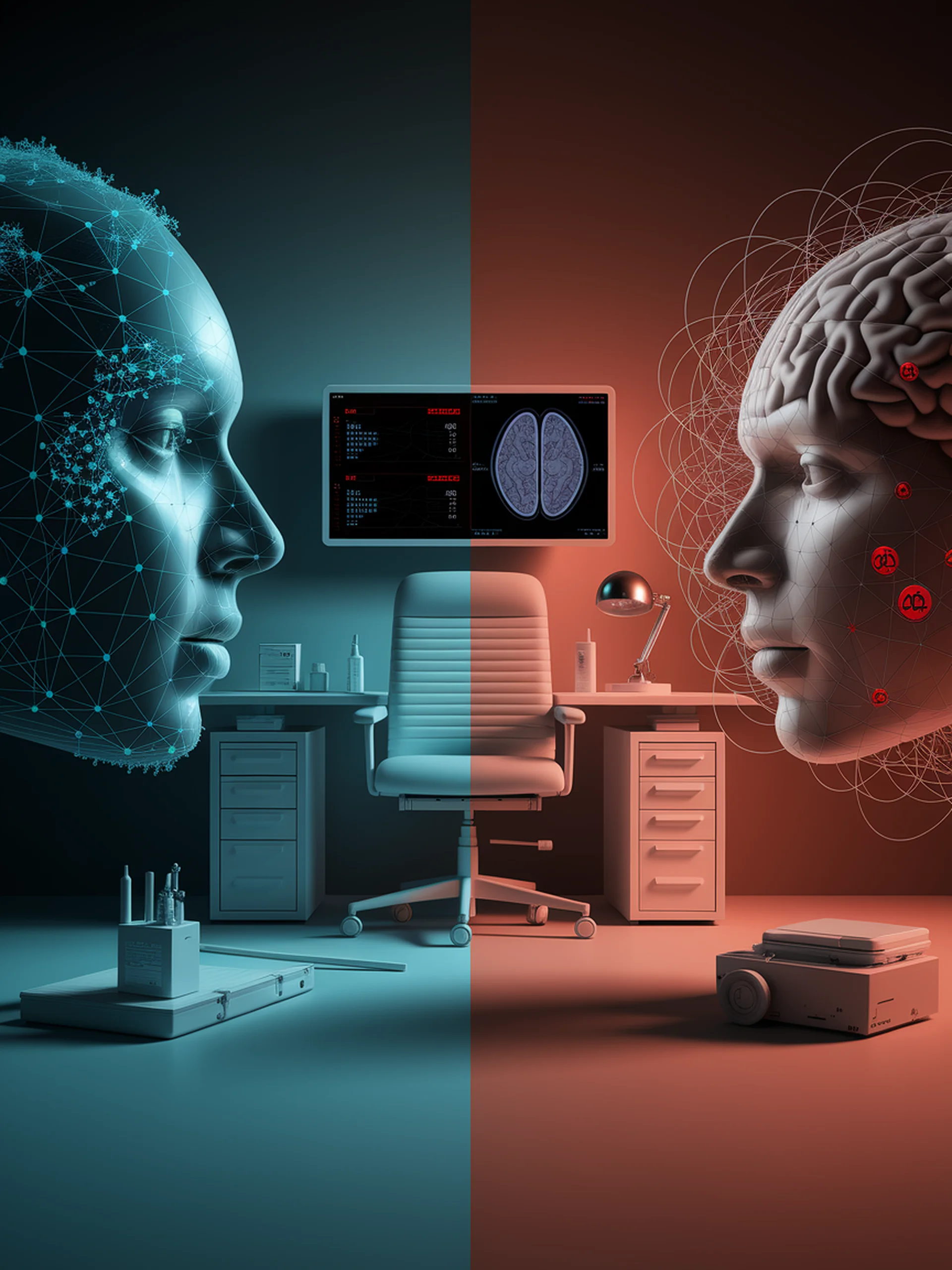
The Unexpected Failure of Pre-trained Models in Depression Detection
Examining why sophisticated AI models underperform in mental health applications
This research investigates why advanced pre-trained models perform poorly in depression detection without extensive data augmentation.
- Speech self-supervised models show unexpectedly low performance in depression detection settings
- Research identifies feature entanglement as a potential root cause of poor performance
- Study explores the untapped potential of Large Language Models for multi-modal depression detection
- Findings have significant implications for developing reliable AI-based mental health screening tools
This work addresses a critical gap in medical AI applications, as improved depression detection systems could help address a pressing global mental health challenge through earlier, more accurate diagnosis.
Why Pre-trained Models Fail: Feature Entanglement in Multi-modal Depression Detection