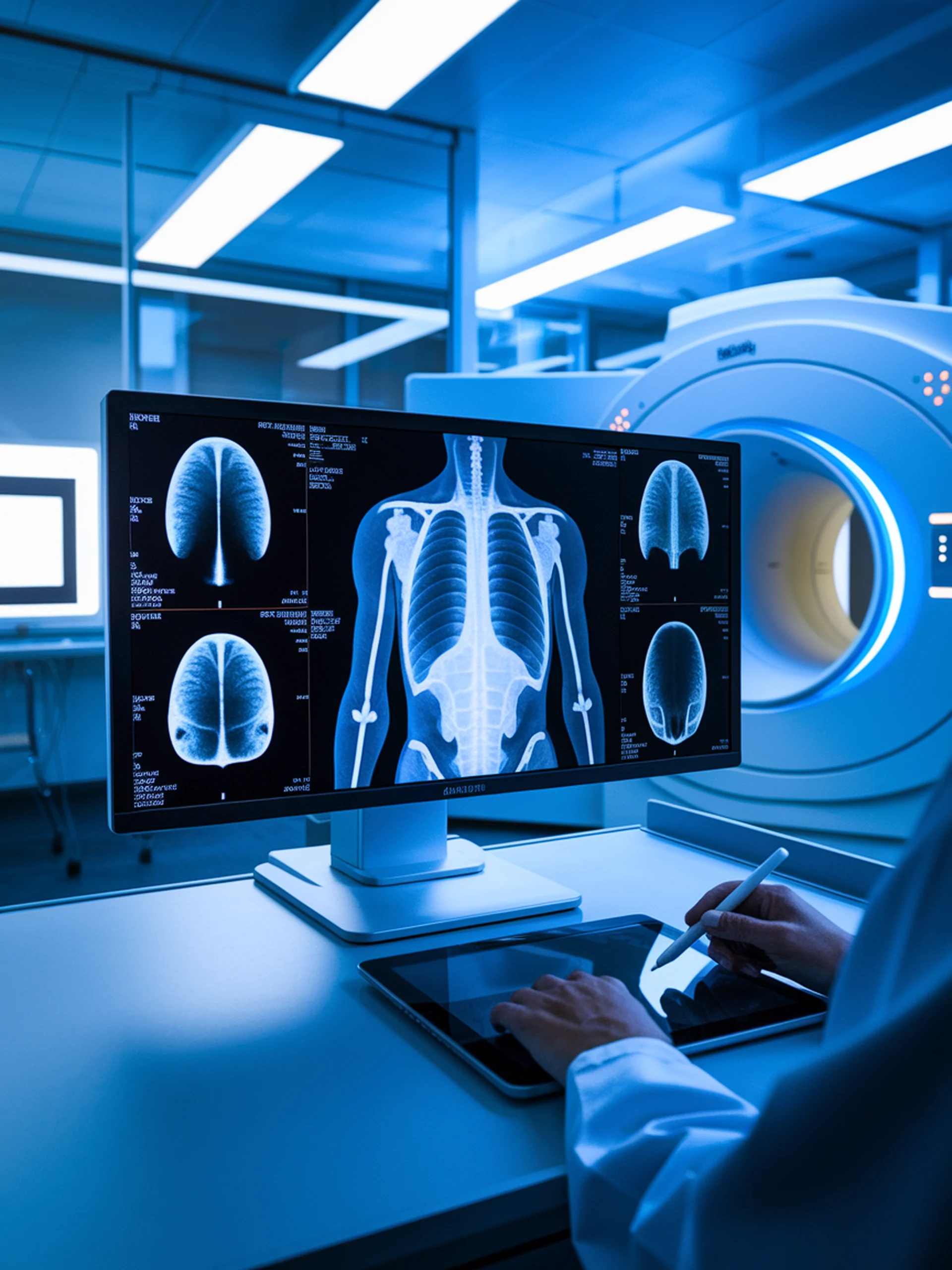
SAT: Revolutionizing Medical Image Segmentation
Universal segmentation of radiology scans using text prompts
This research introduces SAT (Segment Anything with Text), a universal model for medical image segmentation that uses text prompts to identify anatomical structures in radiology scans.
- Created the first multi-modal knowledge tree with 6,502 anatomical terminologies
- Built the largest medical segmentation dataset using 22K+ 3D scans from 72 datasets
- Enables precise identification of anatomical structures using natural language prompts
- Demonstrates strong zero-shot generalization across diverse medical imaging modalities
This breakthrough matters for healthcare by providing radiologists with a flexible, accurate tool that responds to text commands, potentially accelerating diagnoses and improving treatment planning across various medical imaging scenarios.
One Model to Rule them All: Towards Universal Segmentation for Medical Images with Text Prompts