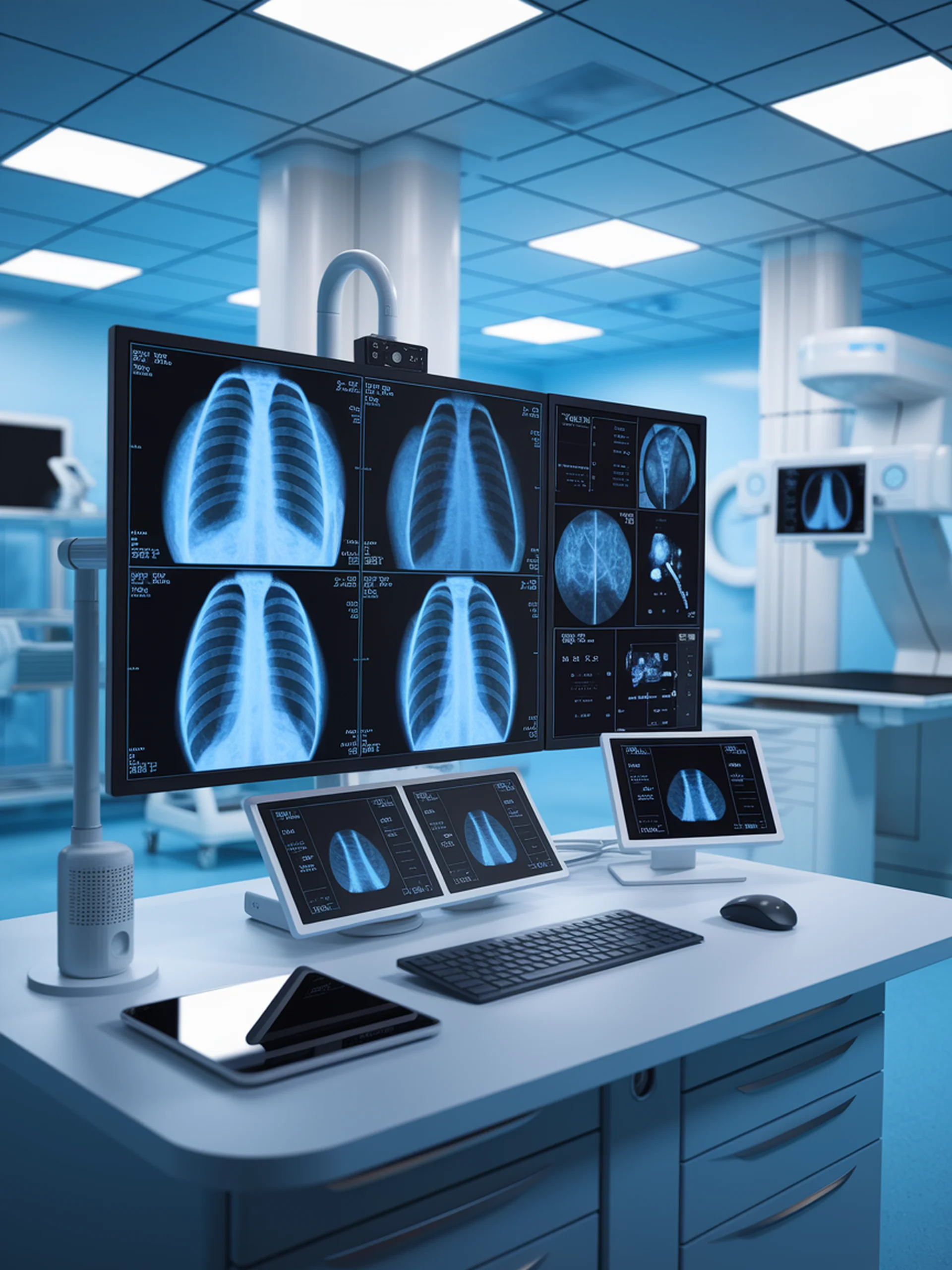
Reimagining X-ray Reports
Advancing Radiology Report Generation with Robust Evaluation Methods
This research addresses fundamental challenges in automated radiology report generation (RRG) systems while introducing improved evaluation metrics that better reflect clinical utility.
- Template Dependency Problem: Existing RRG systems can achieve high metric scores by simply mimicking report templates rather than providing accurate diagnoses
- Enhanced Evaluation Framework: Introduces robust metrics that more fairly assess RRG performance across different models
- Clinical Relevance: Improves the quality of automated medical documentation, potentially reducing radiologist workload and improving patient care
- Practical Applications: Demonstrates how better evaluation metrics lead to more clinically valuable AI-generated reports
Original Paper: X-ray Made Simple: Lay Radiology Report Generation and Robust Evaluation