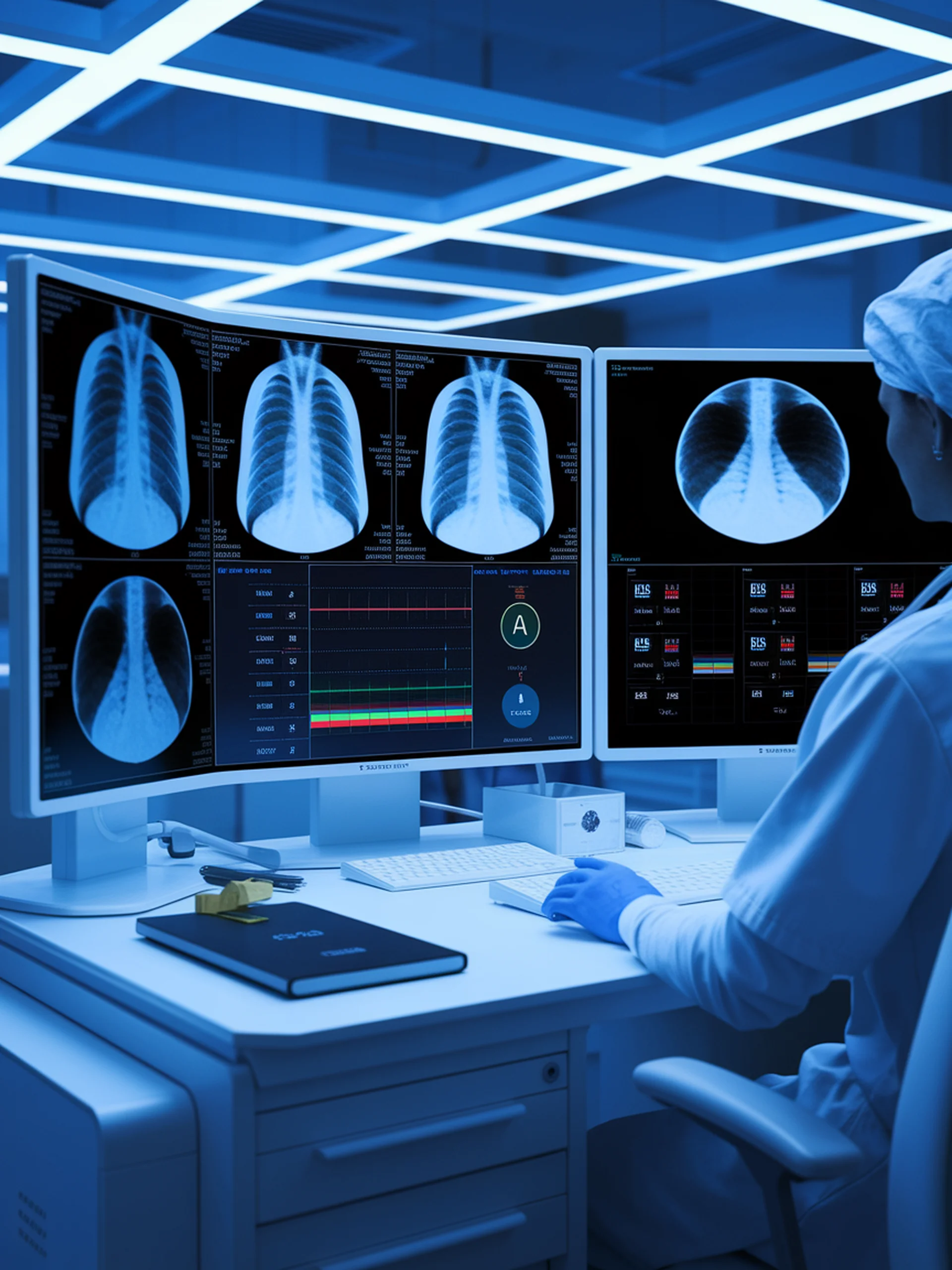
Reducing AI Hallucinations in Medical Reporting
A novel uncertainty quantification approach for factual radiology reports
This research introduces a semantic consistency-based uncertainty quantification framework to identify and reduce hallucinations in AI-generated radiology reports.
- Addresses critical factuality challenges in automated medical report generation
- Proposes a new uncertainty measurement based on semantic consistency
- Demonstrates improved accuracy by identifying areas where the AI model might be uncertain
- Enhances patient safety by flagging potentially inaccurate diagnostic information
For healthcare organizations, this advancement represents a significant step toward safer deployment of AI-assisted radiology reporting systems that radiologists can trust, reducing risks and improving clinical workflow efficiency.
Semantic Consistency-Based Uncertainty Quantification for Factuality in Radiology Report Generation