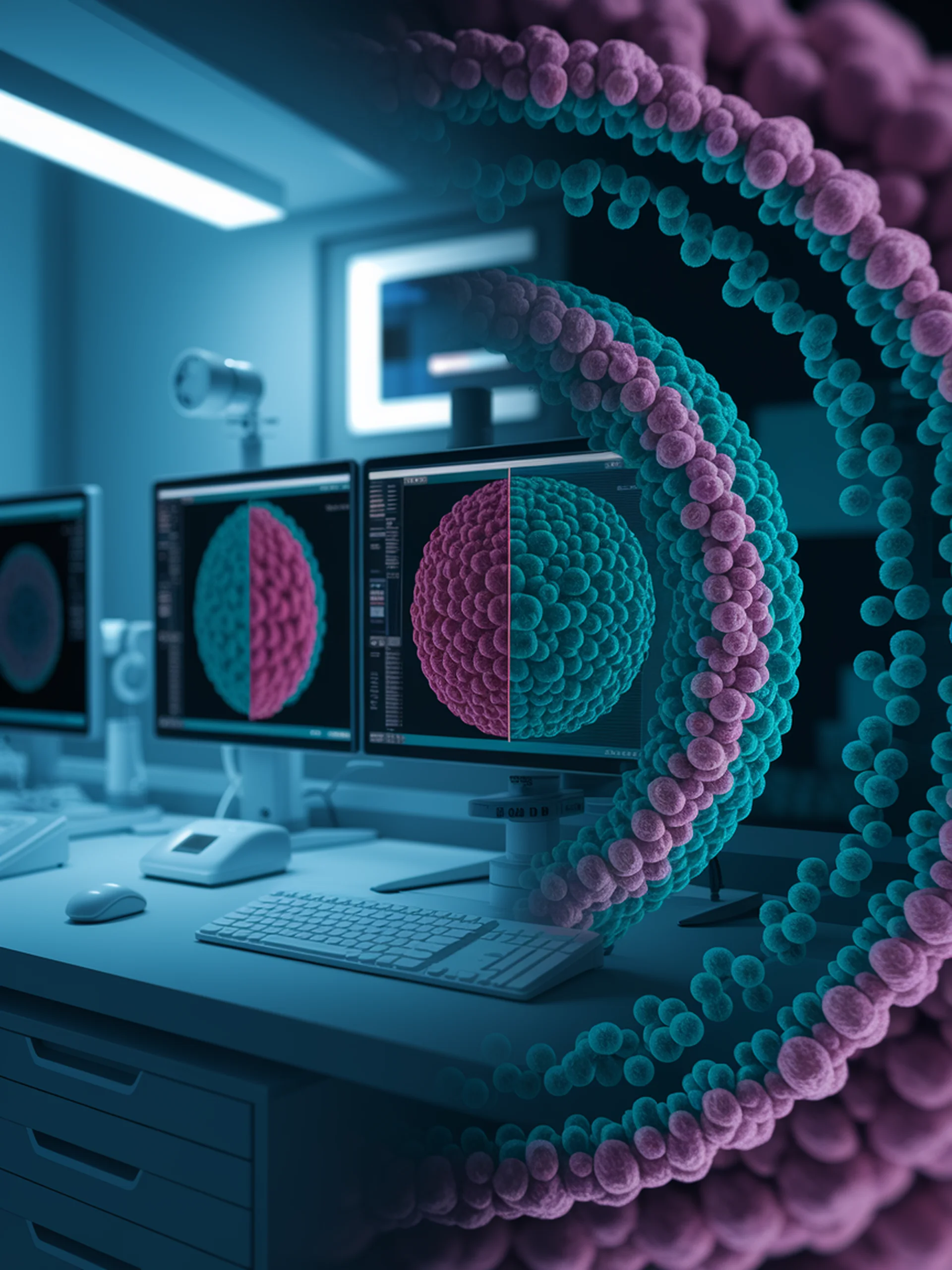
Bridging Vision & Language for Medical Image Analysis
A dual-scale approach to improve cancer classification from pathology images
ViLa-MIL introduces a novel dual-scale vision-language framework that enhances whole slide image classification in pathology by leveraging language models.
- Combines multiple instance learning with vision-language models to analyze gigapixel-sized pathology images
- Uses global-local feature alignment to capture both detailed cellular patterns and broader tissue structures
- Achieves superior performance on multi-cancer classification tasks with reduced dependency on labeled data
- Demonstrates greater robustness against variations in data distribution compared to traditional methods
This research matters because it addresses critical challenges in digital pathology diagnostics, potentially improving cancer subtyping accuracy while requiring fewer labeled examples - a significant advancement for clinical applications.
ViLa-MIL: Dual-scale Vision-Language Multiple Instance Learning for Whole Slide Image Classification