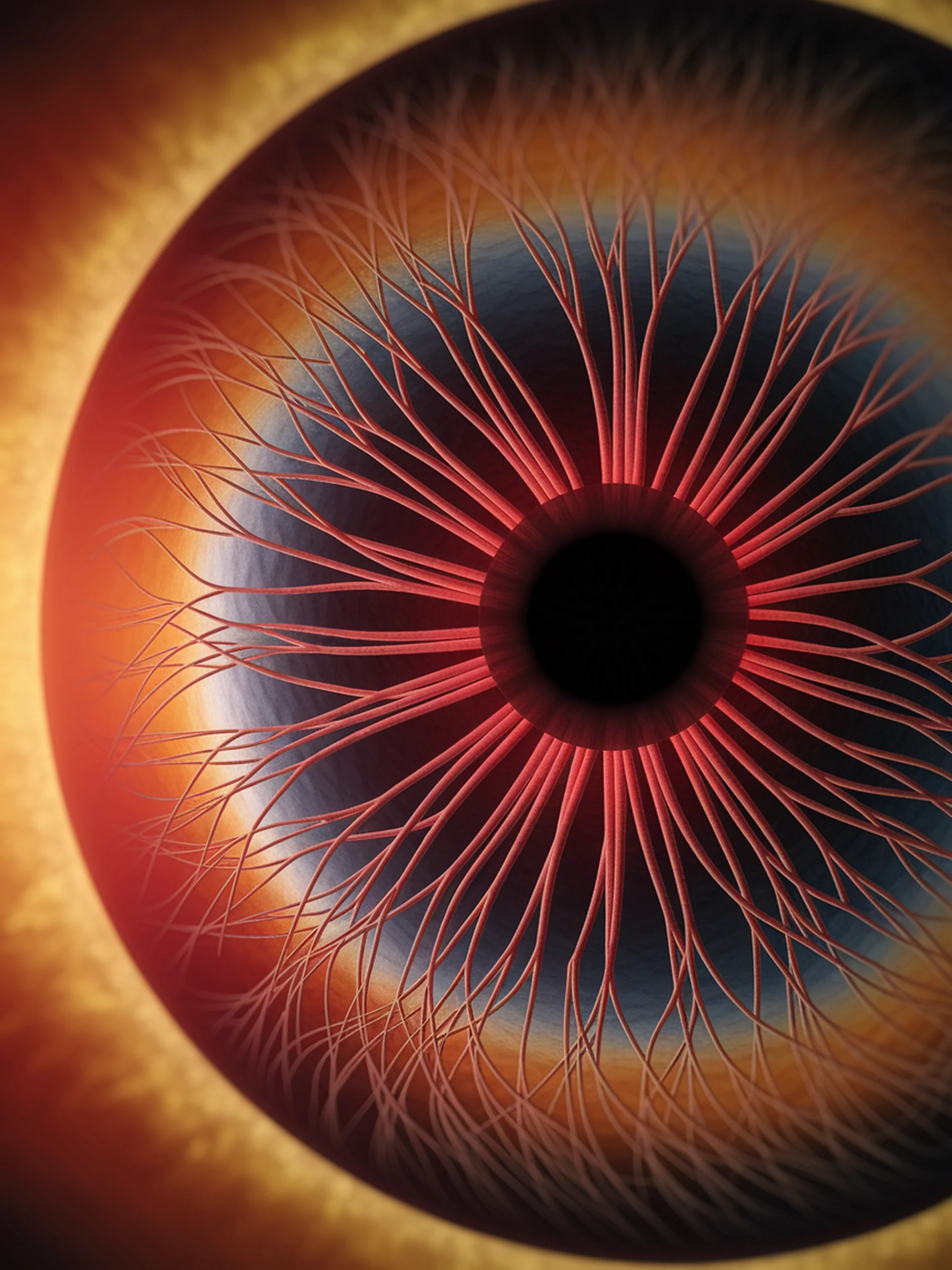
Evaluating AI's Eye for Disease
First comprehensive benchmark for AI models in fundus image interpretation
FunBench introduces a novel benchmark to evaluate how well Multimodal Large Language Models (MLLMs) can interpret retinal fundus images for ophthalmology applications.
- Provides fine-grained evaluation across 5 key tasks in fundus image interpretation
- Separately assesses the vision encoder and language model components of MLLMs
- Reveals significant performance gaps between AI models and human experts
- Identifies specific improvement areas for advancing AI in ophthalmology diagnostics
This research matters because accurate fundus image interpretation is critical for early detection of serious eye conditions and systemic diseases like diabetes and hypertension, potentially expanding access to screening in underserved regions.