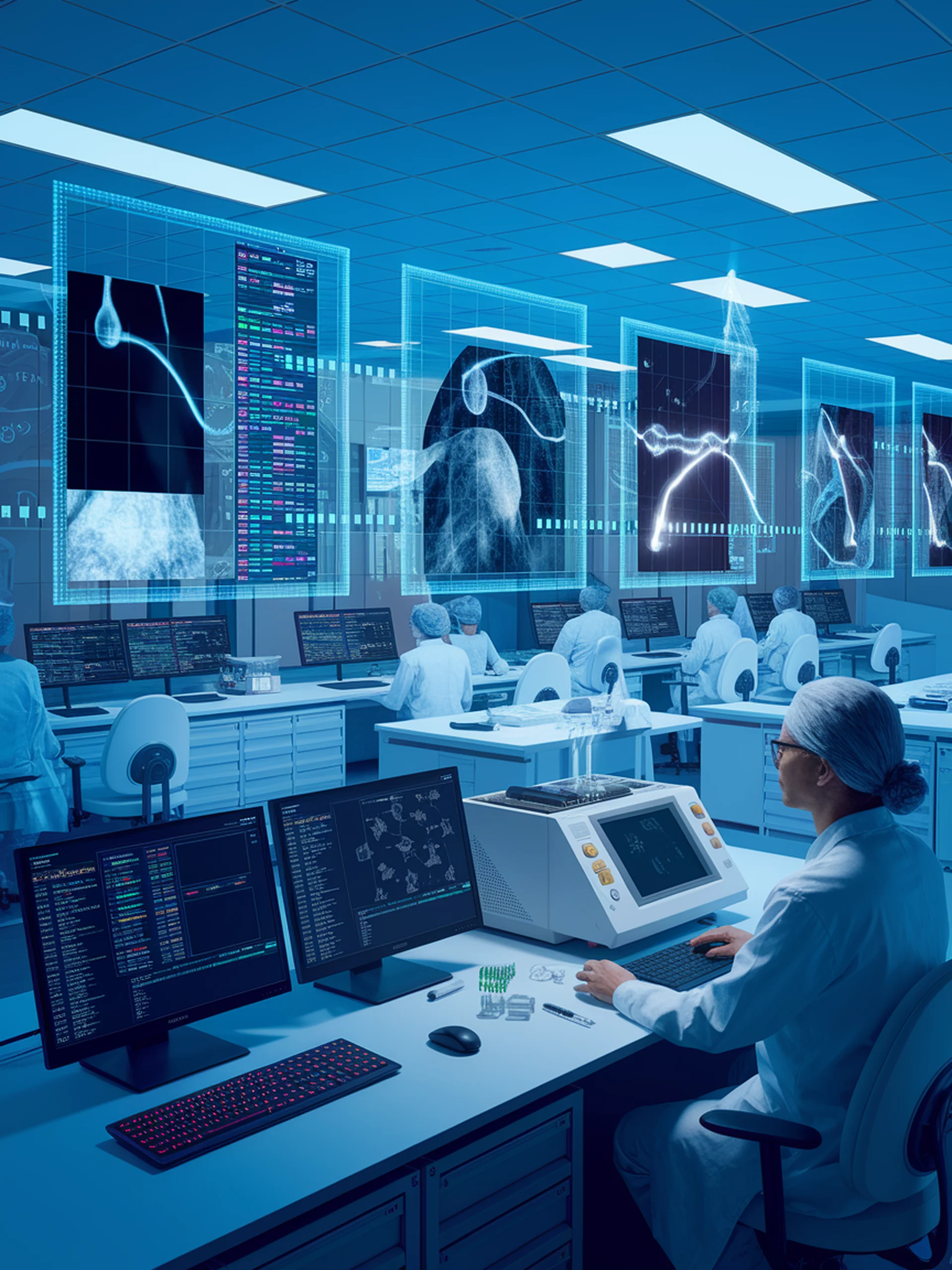
Overcoming Missing Data in Medical Predictions
A novel approach for survival prediction with incomplete multimodal data
This research introduces Distilled Prompt Learning to improve survival predictions when facing incomplete medical data sources (like missing pathology images or gene profiles).
- Develops a framework that effectively compensates for missing modalities in medical data
- Leverages prompt learning to extract and distill knowledge from available data sources
- Enables more reliable patient survival predictions even with incomplete information
- Addresses a critical gap between research models and real-world clinical application
Business Impact: This approach could significantly enhance clinical decision support systems by making multimodal prediction models practical in everyday healthcare settings where complete data is often unavailable.
Distilled Prompt Learning for Incomplete Multimodal Survival Prediction