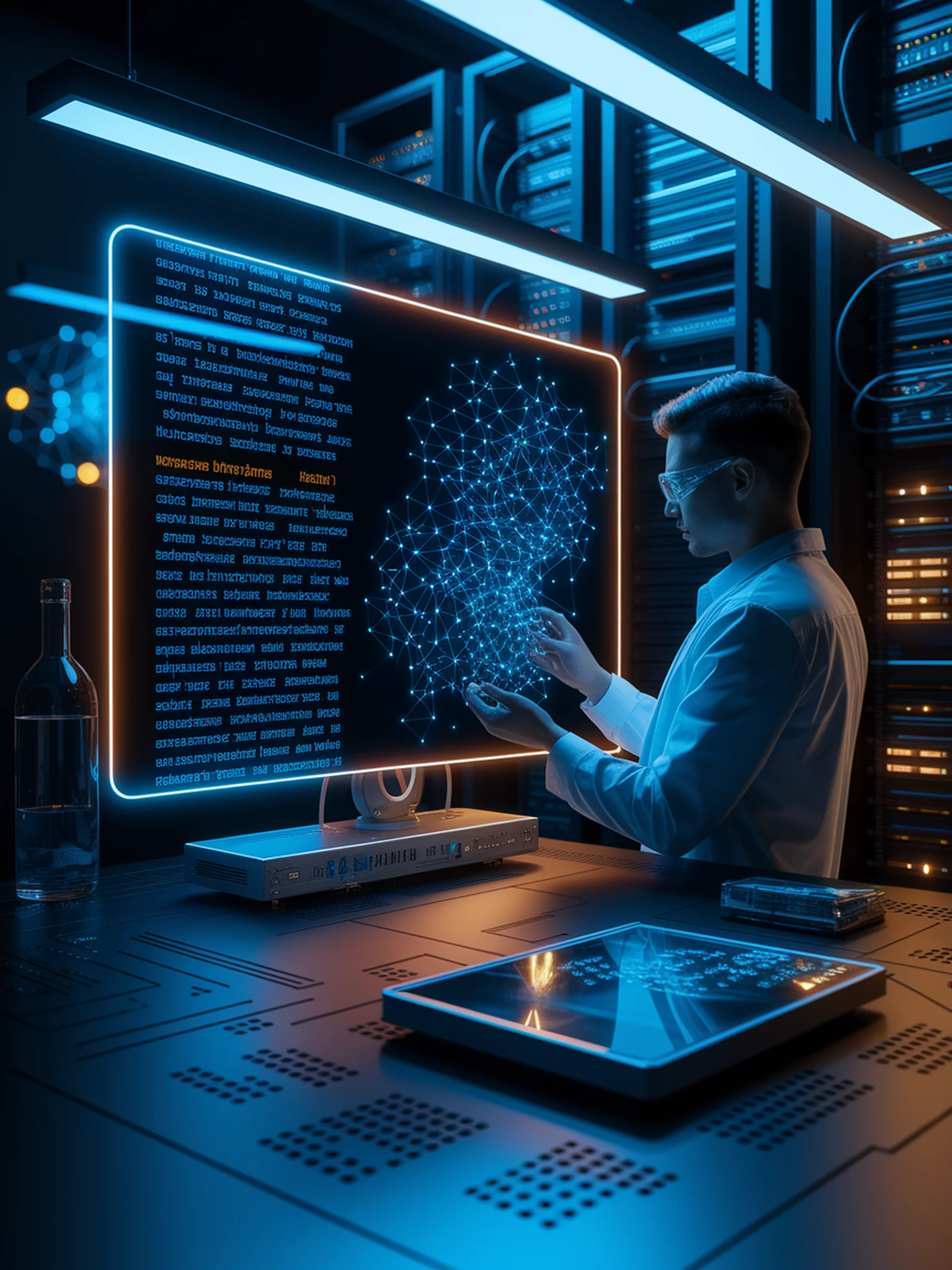
Cracking the Code on AI-Generated Text
Using Sparse Autoencoders to Enhance Detection Interpretability
This research introduces a novel approach to Artificial Text Detection (ATD) by using Sparse Autoencoders to extract interpretable features from LLM internal representations.
- Identified both human-specific and AI-specific features in text generation patterns
- Demonstrated that sparse autoencoders significantly improve ATD interpretability
- Established a foundation for more reliable detection systems that can generalize to new LLMs
- Created a practical framework for understanding why and how AI-generated text differs from human writing
These advances are crucial for security applications as they enable more transparent, explainable detection systems that can adapt to increasingly sophisticated language models and potential misuse.
Original Paper: Feature-Level Insights into Artificial Text Detection with Sparse Autoencoders