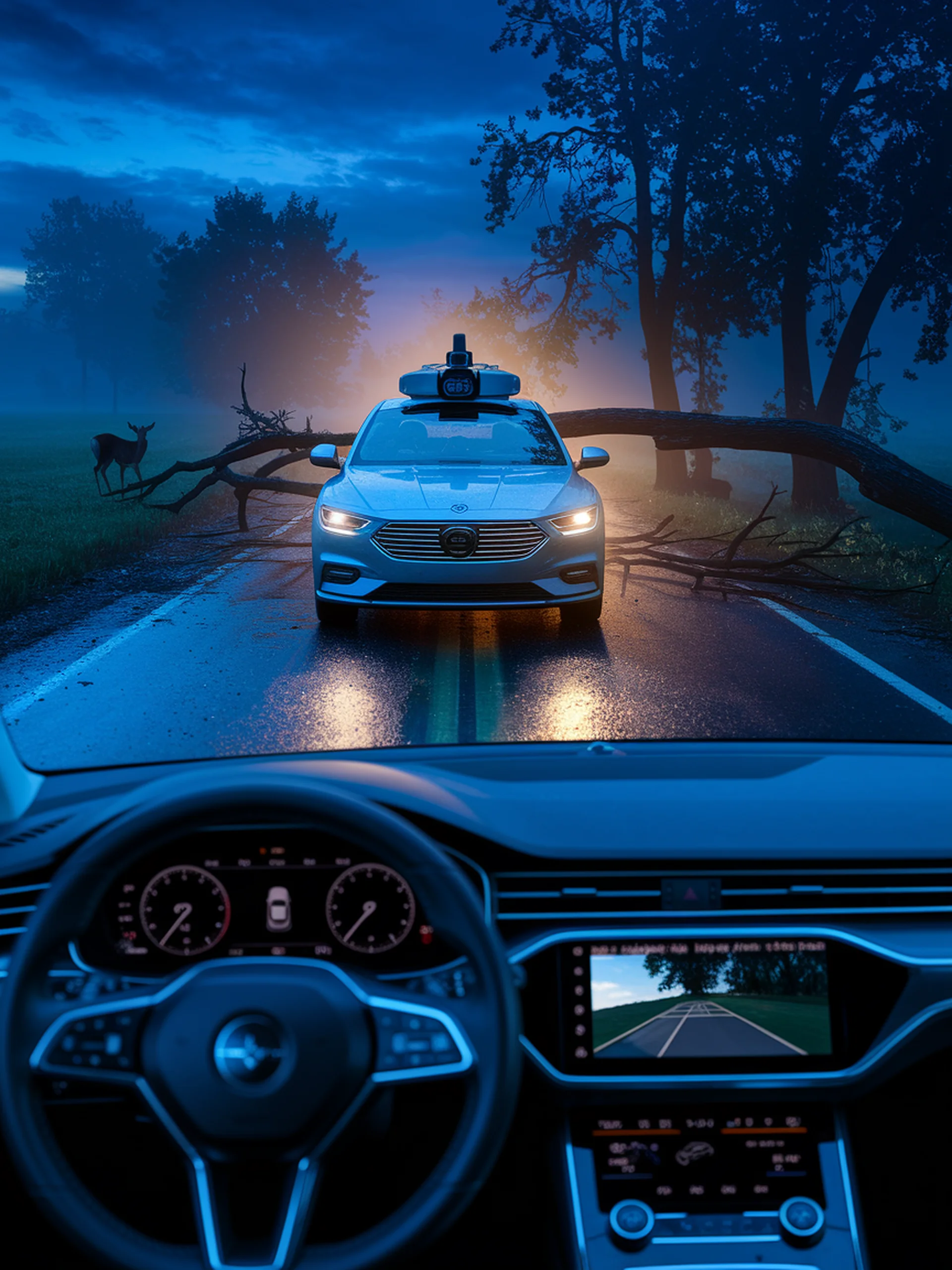
Making Self-Driving Cars Safer in Edge Cases
Vision-Language Models for Handling Rare but Critical Driving Scenarios
VLM-C4L introduces a novel approach to address the critical safety challenge of autonomous vehicles in rare but dangerous driving scenarios.
- Leverages vision-language models to identify and optimize for corner cases
- Creates a continual learning framework that prioritizes safety-critical scenarios
- Addresses a documented safety gap, with hundreds of autonomous vehicle crashes reported annually
- Enhances autonomous driving safety through better handling of complex edge cases
This research directly enhances security and safety in autonomous vehicles by addressing the most dangerous scenarios that currently lead to accidents, making self-driving technology more robust and trustworthy.