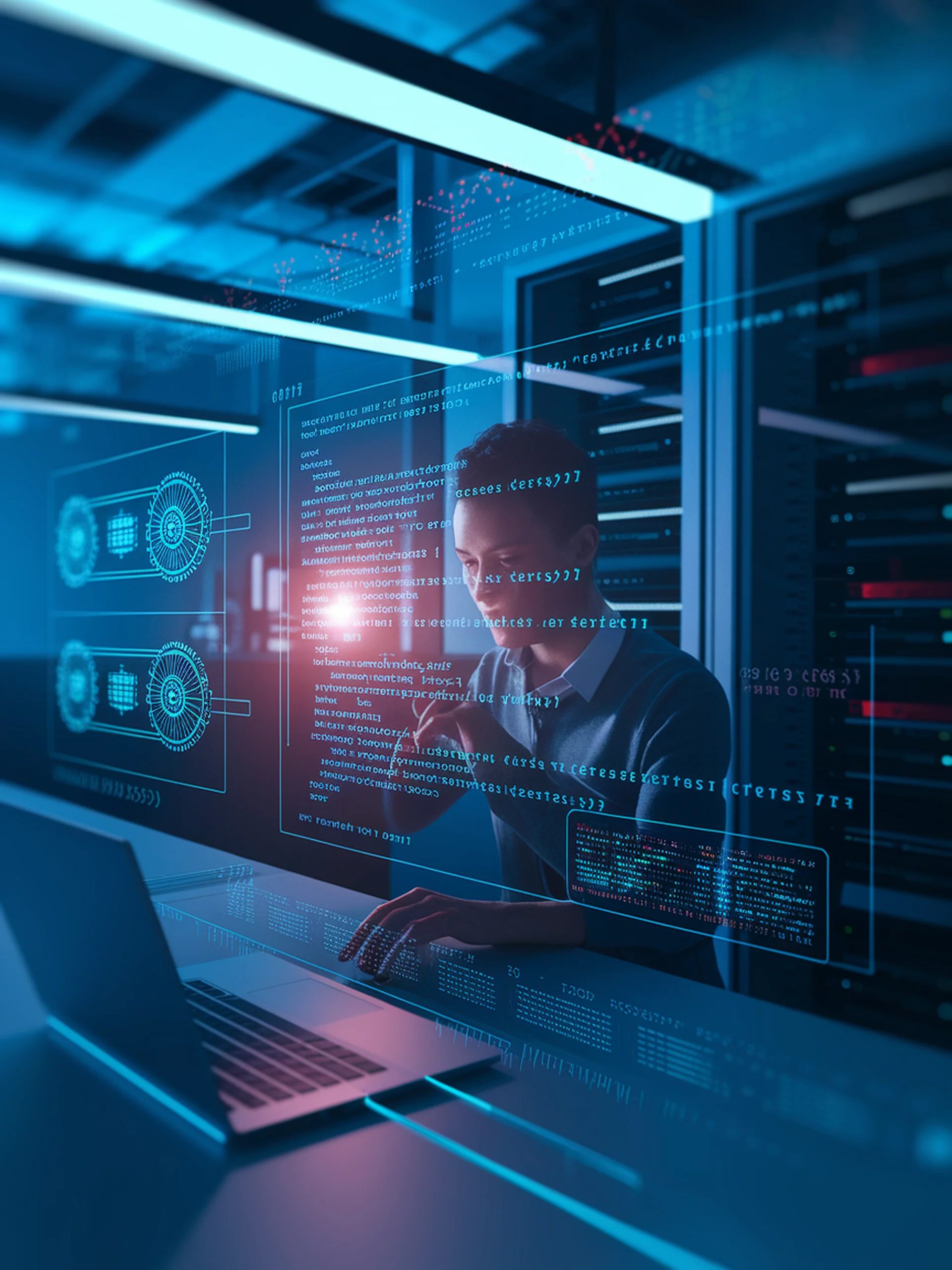
Reinforced Reasoning for Vulnerability Detection
Enhancing LLM security analysis with structured reasoning and reinforcement learning
This research introduces R2Vul, a novel approach that significantly improves LLMs' capability to detect software vulnerabilities through reinforced structured reasoning.
- Combines reinforcement learning with structured reasoning distillation to enhance vulnerability detection
- Addresses limitations of standard chain-of-thought approaches in security contexts
- Teaches LLMs to distinguish between well-founded security assessments and plausible but misleading ones
- Demonstrates superior performance in detecting real-world software vulnerabilities
For security professionals, this advancement represents a critical step toward more reliable automated vulnerability detection, potentially reducing false positives and providing more actionable security insights.