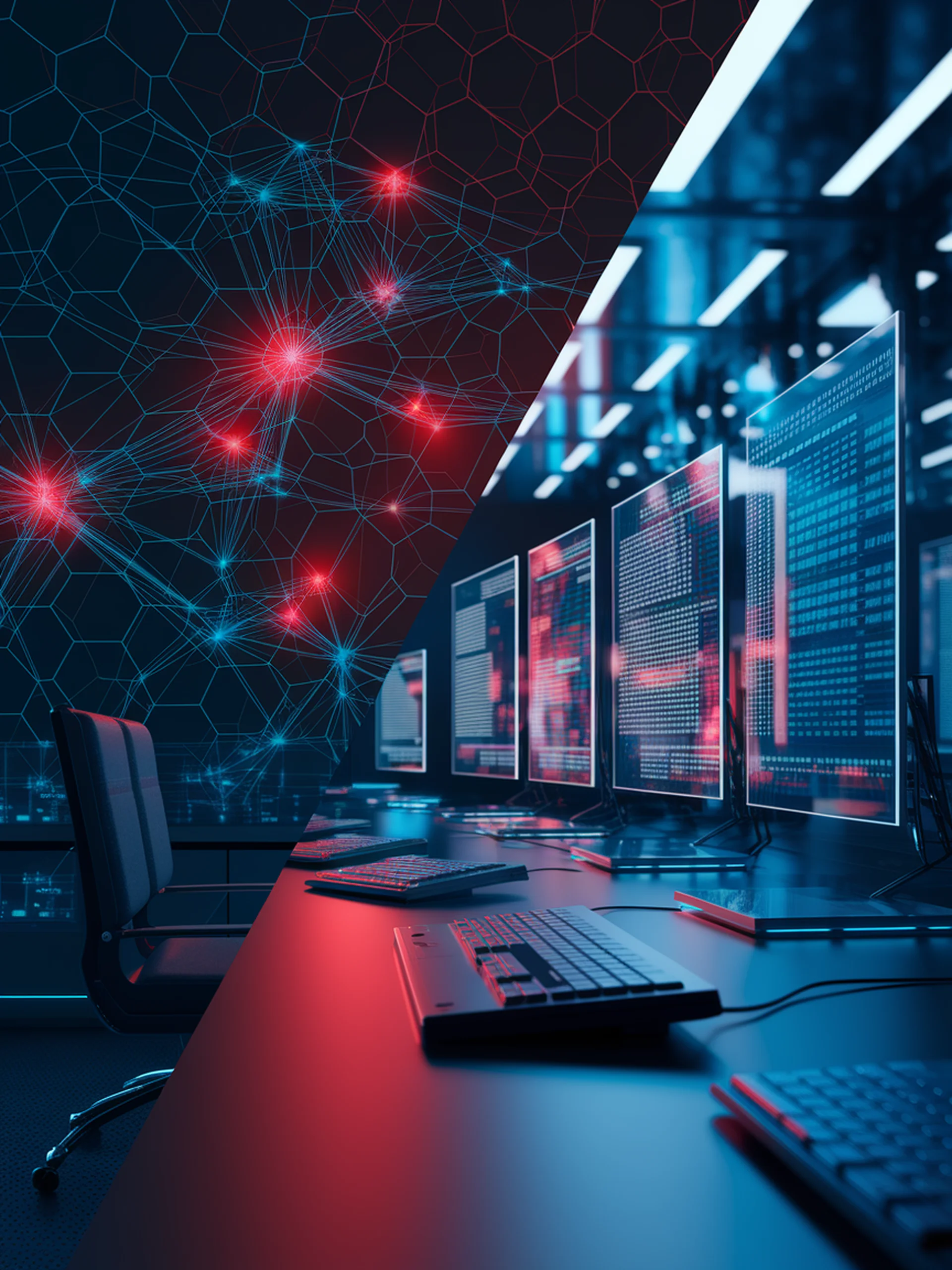
Exposing the Blind Spot in Multimodal LLMs
A Framework to Detect Data Contamination Across Text & Images
This research introduces MM-Detect, a systematic framework for identifying data contamination in multimodal large language models (MLLMs) that can compromise security and evaluation integrity.
- Reveals that both text and images can leak data between training and testing sets
- Demonstrates contamination across multiple commercial MLLMs including GPT-4V and Claude 3
- Provides a comprehensive detection methodology that works across different modalities
- Shows contamination can lead to artificially inflated performance metrics on benchmarks
This work matters for Security professionals because it exposes fundamental vulnerabilities in how MLLMs are trained and evaluated, enabling more reliable model assessment and reducing potential exploitation of contaminated models in security-critical applications.
Both Text and Images Leaked! A Systematic Analysis of Multimodal LLM Data Contamination