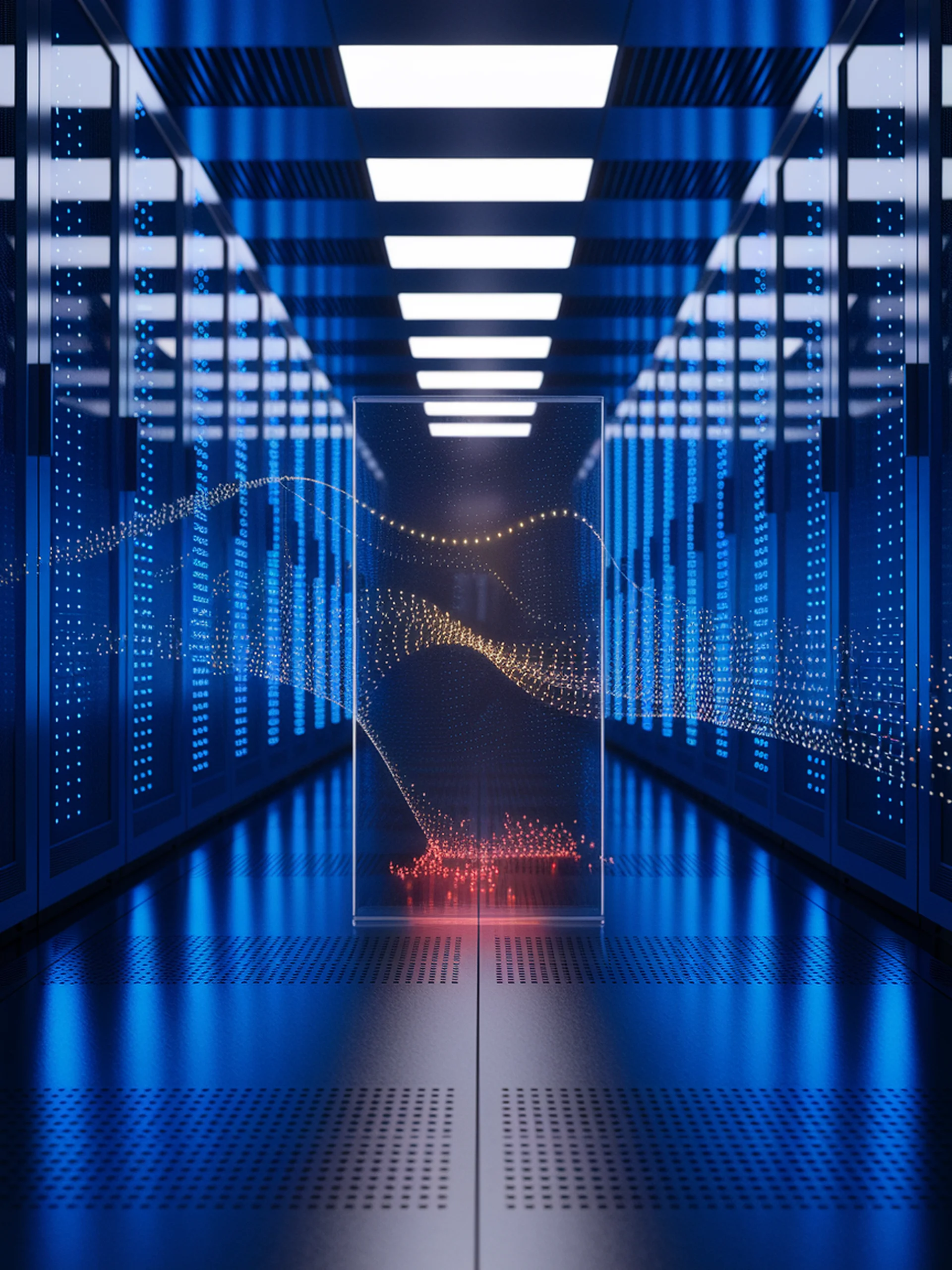
Quantifying Data Extraction Risks in LLMs
A sequence-level probability approach to measuring training data leakage
This research presents a novel approach to measuring training data leakage risks in large language models by analyzing sequence-level probabilities.
- Provides finer-grained analysis of extraction vulnerabilities than previous benchmark methods
- Re-examines effects of decoding schemes, model sizes, and prefix lengths on data leakage
- Reveals new insights about extraction patterns that weren't visible with traditional metrics
- Identifies specific security vulnerabilities related to partial sequence extraction
This work is critical for organizations deploying LLMs, as it helps quantify privacy risks and informs better protection measures for sensitive training data. Understanding these risks is essential for developing more secure AI systems and maintaining compliance with data protection regulations.
Sequence-Level Leakage Risk of Training Data in Large Language Models