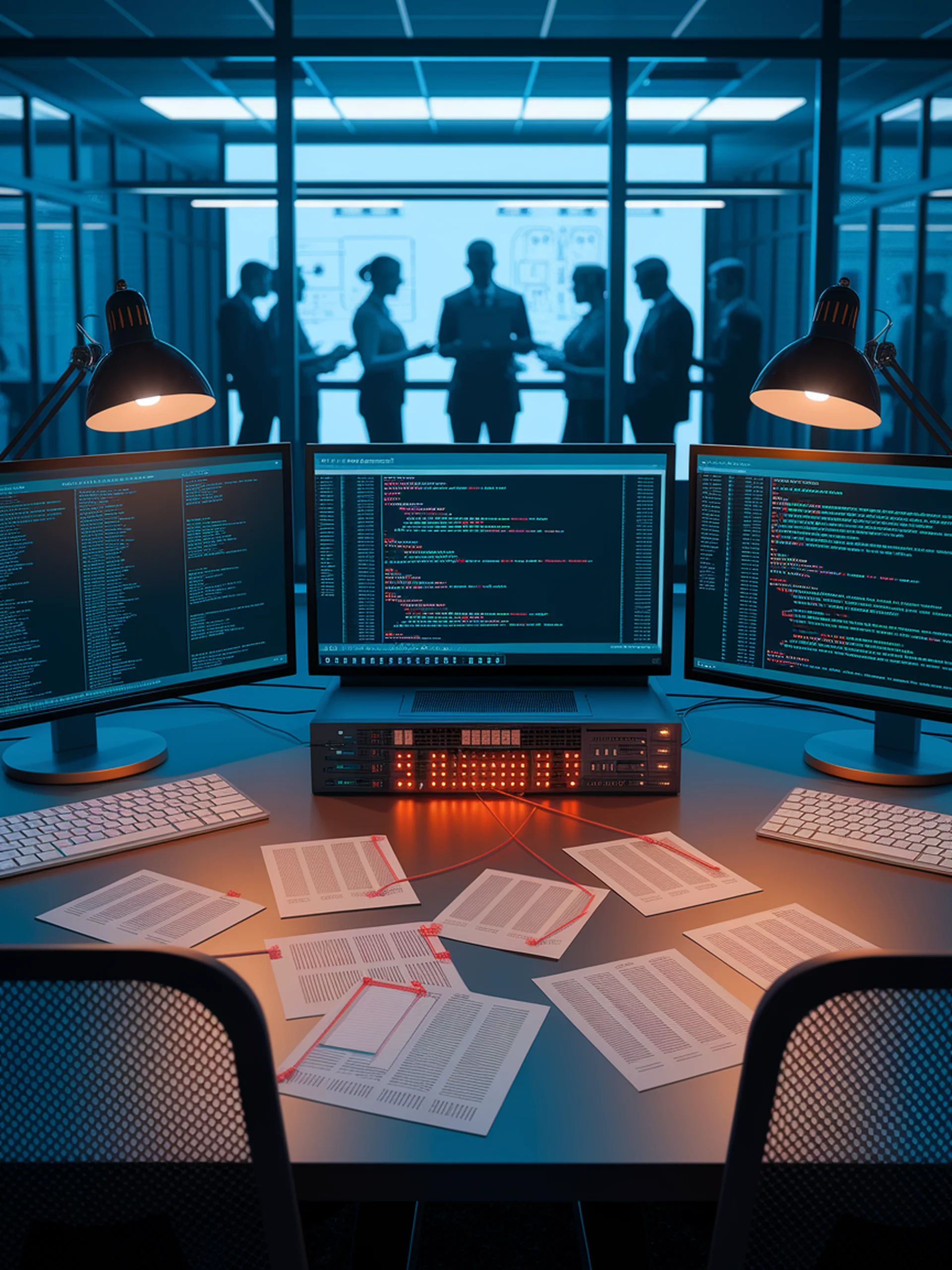
The LLM Memorization Challenge
How language models can complete texts they weren't explicitly trained on
This research reveals a critical gap in how we test whether text was used to train large language models, demonstrating that conventional testing methods can be manipulated.
Key findings:
- Current completion tests for determining training data are vulnerable to manipulation
- LLMs can verbatim reproduce content without explicit training through n-gram overlap
- This creates significant security vulnerabilities in model auditing approaches
- Traditional membership definitions based on n-gram presence are insufficient for security guarantees
For security professionals and AI governance teams, this research highlights the urgent need to develop more robust methods for verifying training data and protecting against potential data leakage or misuse.
Language Models May Verbatim Complete Text They Were Not Explicitly Trained On