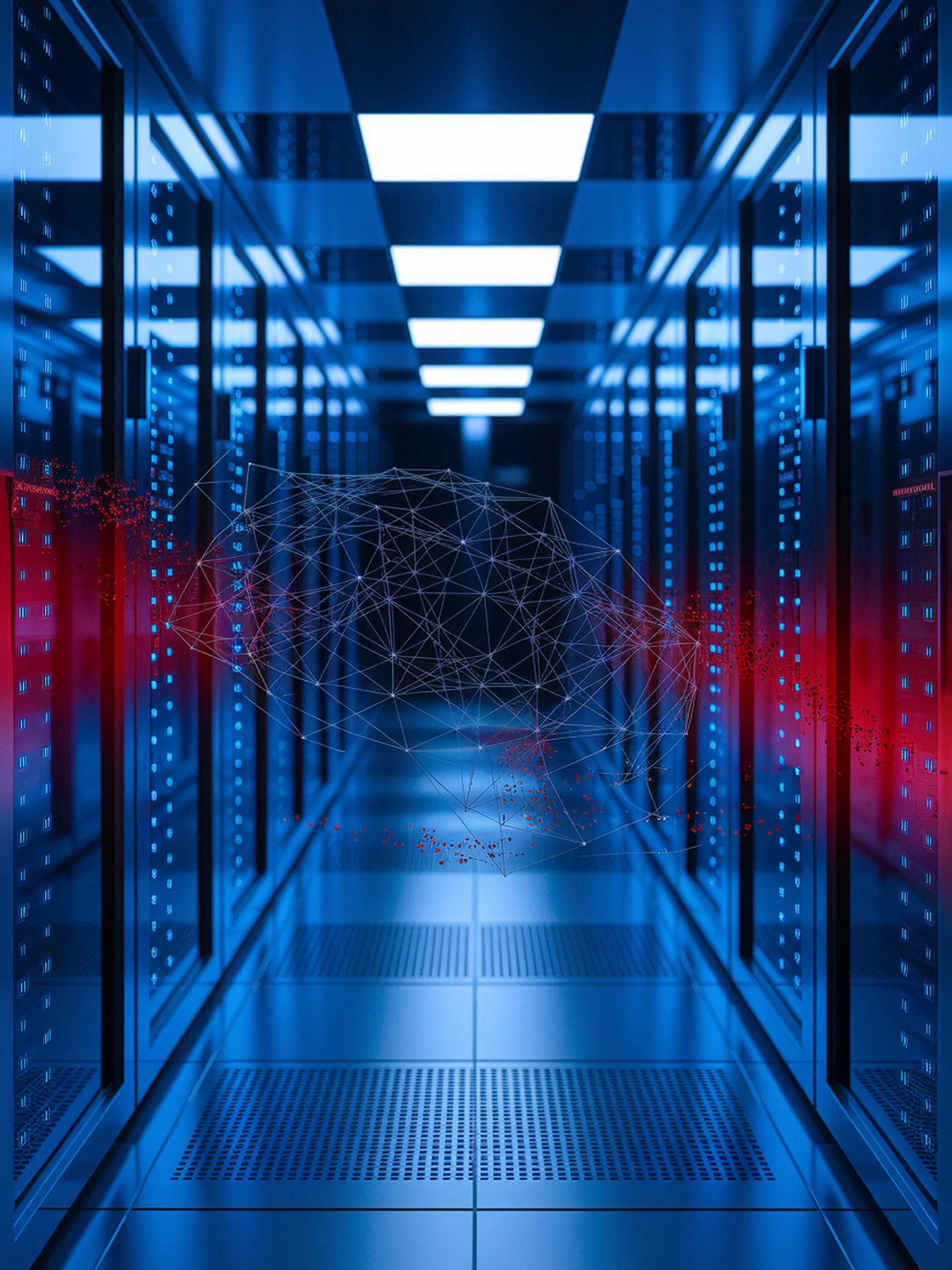
Defending Against Data Poisoning
Understanding threats to deep learning security
This comprehensive survey examines how malicious data manipulation can compromise deep learning models during training, threatening AI security and reliability.
- Analyzes various attack mechanisms used to degrade model accuracy or induce targeted behaviors
- Maps the evolution of poisoning techniques across different deep learning applications
- Evaluates existing defense strategies and their effectiveness against sophisticated attacks
- Identifies critical gaps in current protection approaches and future research directions
For security professionals, this research provides essential insights into protecting AI systems from adversarial training data manipulation, a growing concern as models increasingly depend on large, potentially contaminated datasets.