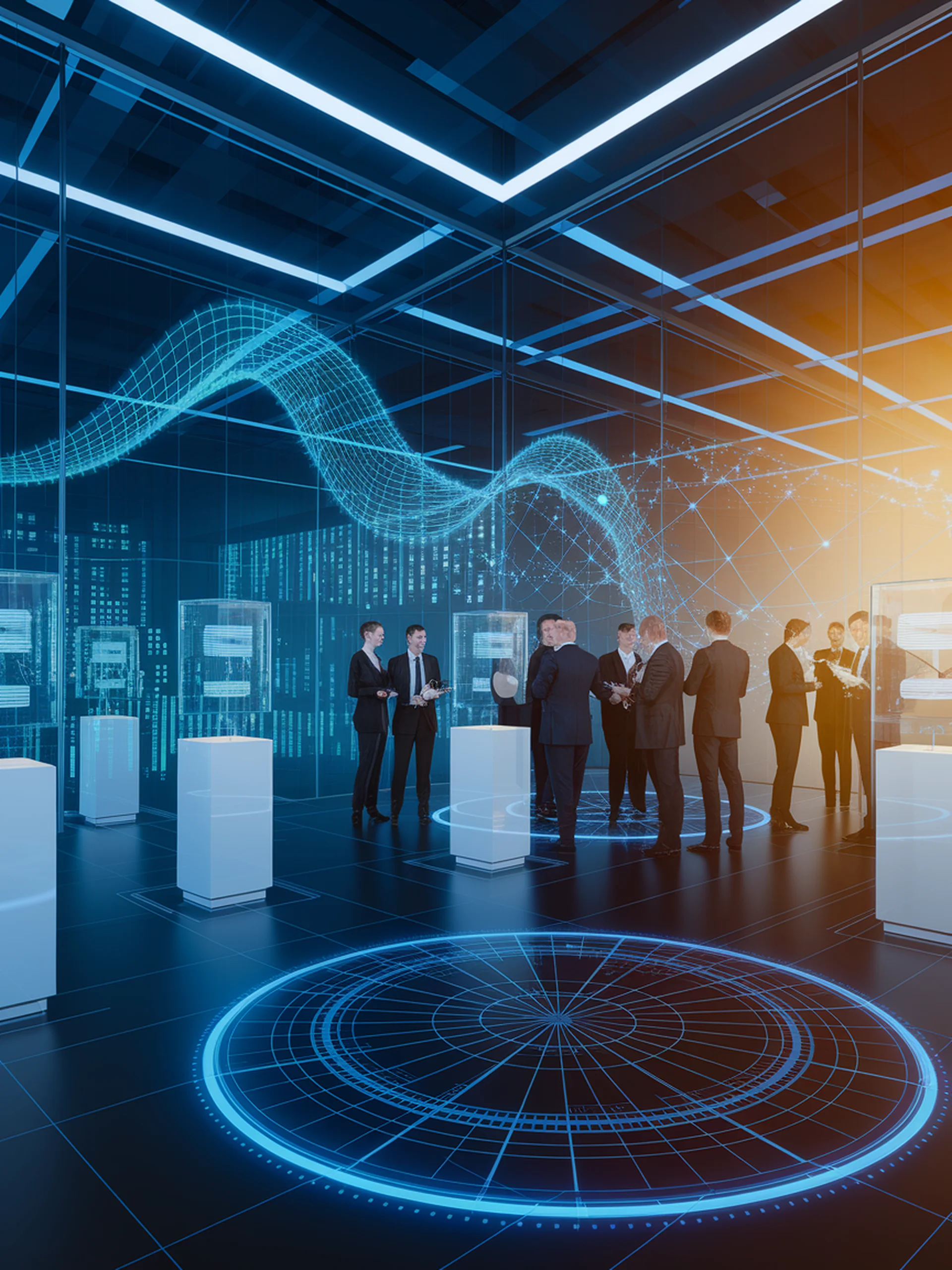
Rethinking Data Markets for LLMs
Game theory reveals flaws in current data valuation methods
This research examines how we can design truthful data markets that fairly compensate data contributors for large language models.
- Traditional valuation methods (Leave-One-Out, Data Shapley) fail to ensure honest cost reporting
- Game-theoretic analysis reveals fundamental inefficiencies in current market designs
- Authors propose alternative mechanisms for truthful cost revelation
- Implications directly impact data security and fair compensation strategies
For security professionals, this work highlights the importance of incentive-compatible data sharing frameworks that protect both contributors and the integrity of AI training processes.
From Fairness to Truthfulness: Rethinking Data Valuation Design