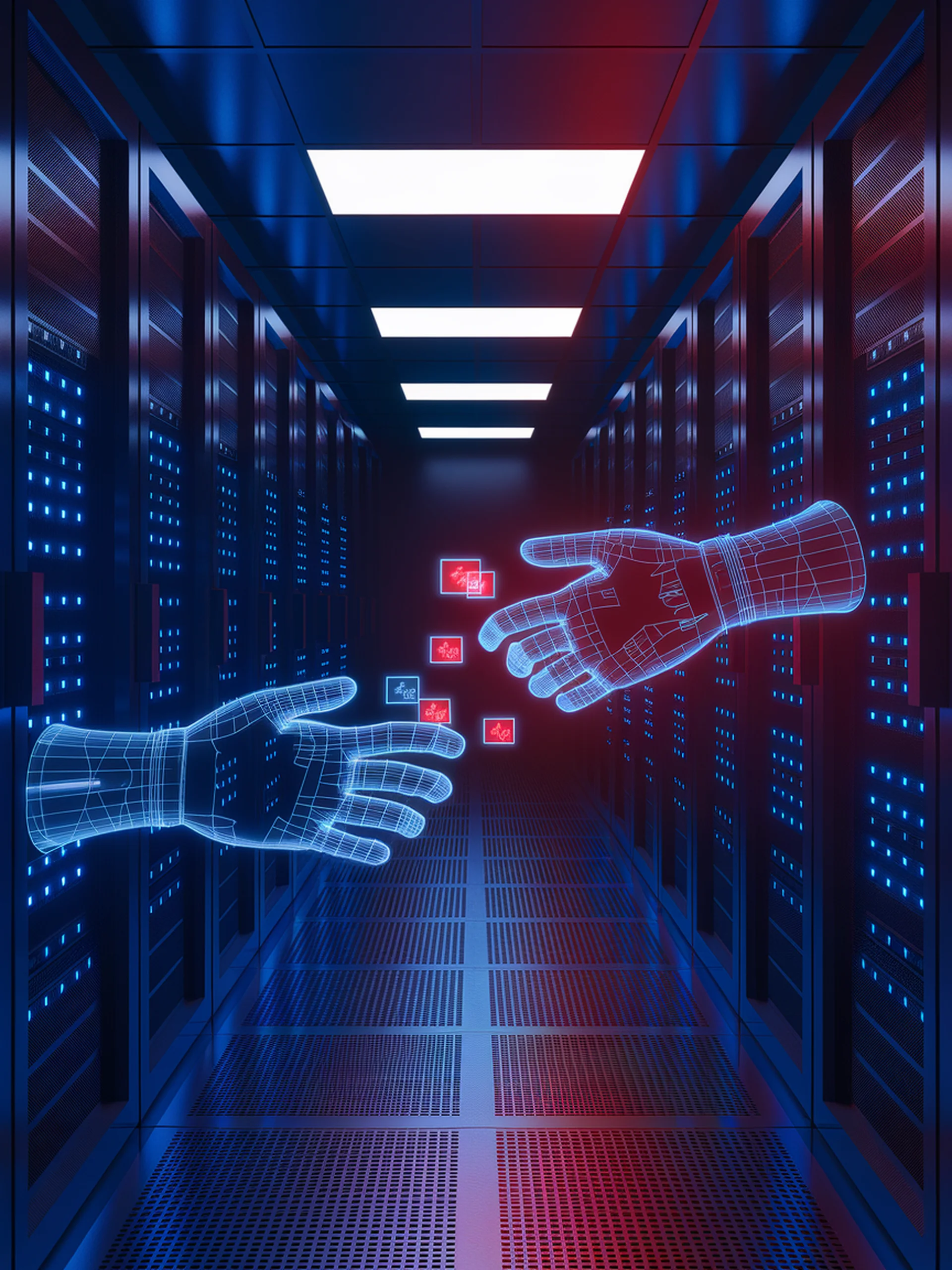
Privacy Vulnerabilities in Federated Learning
How attackers can steal sensitive data from language models without accessing raw data
This research reveals how malicious participants can extract private information from federated learning systems through strategic weight manipulation despite never seeing the original training data.
- Introduces FLTrojan, a novel attack that selectively tampers with model weights to extract sensitive data like medical records and credentials
- Demonstrates successful extraction of up to 96% of targeted sensitive information
- Shows current aggregation defenses are insufficient against these sophisticated attacks
- Proposes potential countermeasures to detect and mitigate these vulnerabilities
This work highlights critical security implications for organizations implementing federated learning in privacy-sensitive domains like healthcare and finance, where data protection is paramount.