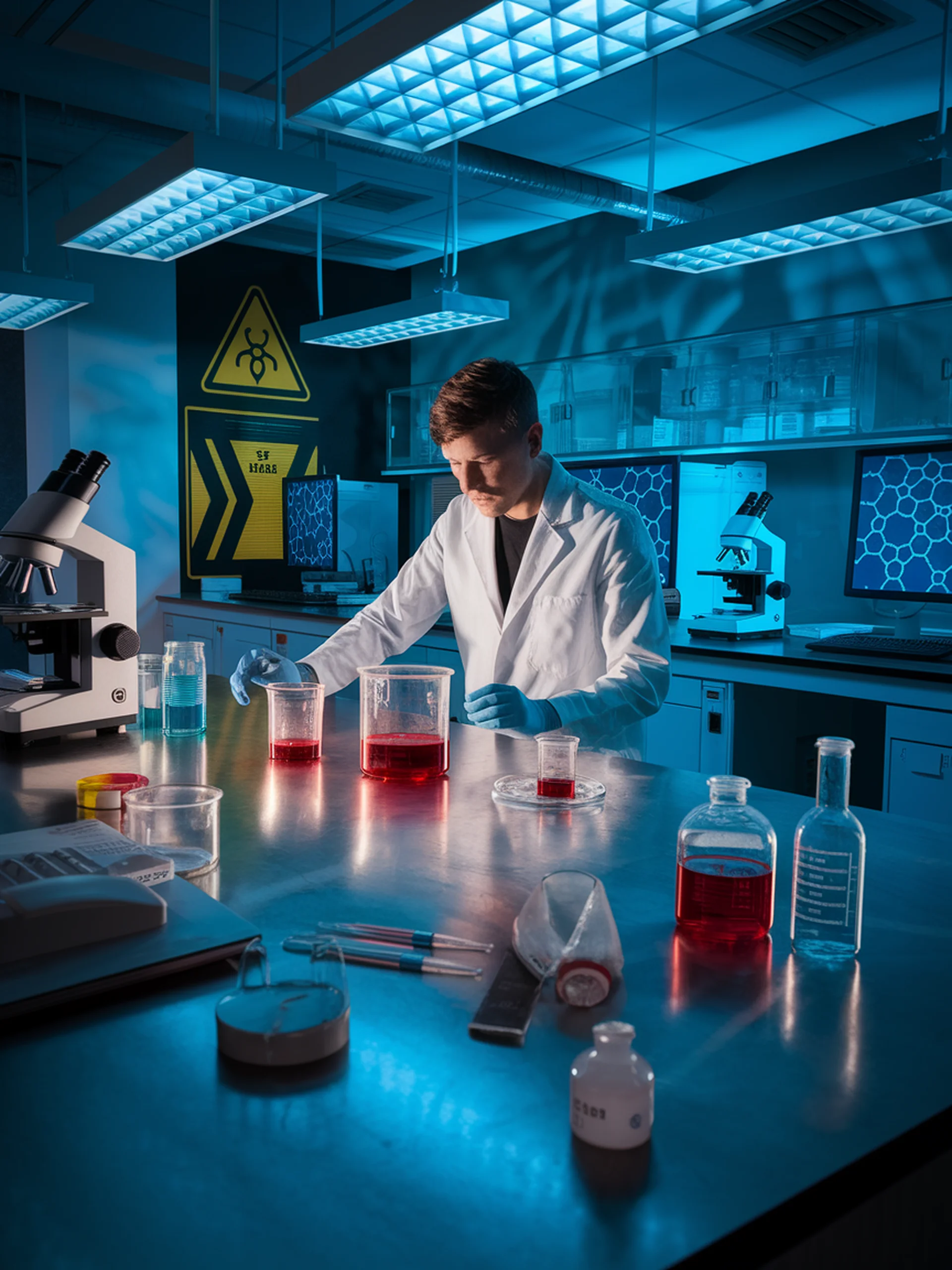
Safety Blind Spots in AI Lab Assistants
Benchmarking LLMs on Critical Laboratory Safety Risks
This research establishes a novel benchmark for evaluating how well large language models (LLMs) handle safety-critical laboratory scenarios, revealing concerning gaps in their reliability for high-stakes scientific environments.
- LLMs frequently fail to identify hazards and provide unsafe recommendations in laboratory contexts
- Models exhibit an illusion of understanding that may lead researchers to overestimate their reliability
- Even leading models struggle with safety protocols and risk assessment aligned with OSHA standards
- The research introduces a specialized benchmark to systematically evaluate and improve LLM safety performance in scientific settings
This work has significant implications for security in scientific research, highlighting the dangers of uncritical AI adoption in labs where failures could result in physical harm, contamination, or regulatory violations.
LabSafety Bench: Benchmarking LLMs on Safety Issues in Scientific Labs